Note
Click here to download the full example code or to run this example in your browser via Binder
02. Convert MNE sample data to BIDS format¶
In this example we will use MNE-BIDS to organize the MNE sample data according to the BIDS standard. In a second step we will read the organized dataset using MNE-BIDS.
# Authors: Mainak Jas <mainak.jas@telecom-paristech.fr>
# Alexandre Gramfort <alexandre.gramfort@telecom-paristech.fr>
# Teon Brooks <teon.brooks@gmail.com>
# Stefan Appelhoff <stefan.appelhoff@mailbox.org>
# Richard Höchenberger <richard.hoechenberger@gmail.com>
#
# License: BSD (3-clause)
First we import some basic Python libraries, followed by MNE-Python and its sample data, and then finally the MNE-BIDS functions we need for this example
import os.path as op
import shutil
import mne
from mne.datasets import sample
from mne_bids import (write_raw_bids, read_raw_bids, write_meg_calibration,
write_meg_crosstalk, BIDSPath, print_dir_tree)
from mne_bids.stats import count_events
Now we can read the MNE sample data. We define an event_id based on our knowledge of the data, to give meaning to events in the data.
With raw_fname and events_data, we determine where to get the sample data from. output_path determines where we will write the BIDS conversion to.
data_path = sample.data_path()
event_id = {'Auditory/Left': 1, 'Auditory/Right': 2, 'Visual/Left': 3,
'Visual/Right': 4, 'Smiley': 5, 'Button': 32}
raw_fname = op.join(data_path, 'MEG', 'sample', 'sample_audvis_raw.fif')
events_data = op.join(data_path, 'MEG', 'sample', 'sample_audvis_raw-eve.fif')
output_path = op.join(data_path, '..', 'MNE-sample-data-bids')
To ensure the output path doesn’t contain any leftover files from previous tests and example runs, we simply delete it.
Warning
Do not delete directories that may contain important data!
Note
mne-bids
will try to infer as much information from the data as
possible to then save this data in BIDS-specific “sidecar” files. For
example the manufacturer information, which is inferred from the data file
extension. However, sometimes inferring is ambiguous (e.g., if your file
format is non-standard for the manufacturer). In these cases, MNE-BIDS does
not guess and you will have to update your BIDS fields manually.
Based on our path definitions above, we read the raw data file, define a new BIDS name for it, and then run the automatic BIDS conversion.
raw = mne.io.read_raw_fif(raw_fname)
raw.info['line_freq'] = 60 # specify power line frequency as required by BIDS
bids_path = BIDSPath(subject='01', session='01',
task='audiovisual', run='01', root=output_path)
write_raw_bids(raw, bids_path, events_data=events_data,
event_id=event_id, overwrite=True)
Out:
Opening raw data file /Users/hoechenberger/mne_data/MNE-sample-data/MEG/sample/sample_audvis_raw.fif...
Read a total of 3 projection items:
PCA-v1 (1 x 102) idle
PCA-v2 (1 x 102) idle
PCA-v3 (1 x 102) idle
Range : 25800 ... 192599 = 42.956 ... 320.670 secs
Ready.
Opening raw data file /Users/hoechenberger/mne_data/MNE-sample-data/MEG/sample/sample_audvis_raw.fif...
Read a total of 3 projection items:
PCA-v1 (1 x 102) idle
PCA-v2 (1 x 102) idle
PCA-v3 (1 x 102) idle
Range : 25800 ... 192599 = 42.956 ... 320.670 secs
Ready.
Writing '/Users/hoechenberger/mne_data/MNE-sample-data/../MNE-sample-data-bids/README'...
References
----------
Appelhoff, S., Sanderson, M., Brooks, T., Vliet, M., Quentin, R., Holdgraf, C., Chaumon, M., Mikulan, E., Tavabi, K., Höchenberger, R., Welke, D., Brunner, C., Rockhill, A., Larson, E., Gramfort, A. and Jas, M. (2019). MNE-BIDS: Organizing electrophysiological data into the BIDS format and facilitating their analysis. Journal of Open Source Software 4: (1896). https://doi.org/10.21105/joss.01896
Niso, G., Gorgolewski, K. J., Bock, E., Brooks, T. L., Flandin, G., Gramfort, A., Henson, R. N., Jas, M., Litvak, V., Moreau, J., Oostenveld, R., Schoffelen, J., Tadel, F., Wexler, J., Baillet, S. (2018). MEG-BIDS, the brain imaging data structure extended to magnetoencephalography. Scientific Data, 5, 180110. https://doi.org/10.1038/sdata.2018.110
Writing '/Users/hoechenberger/mne_data/MNE-sample-data/../MNE-sample-data-bids/participants.tsv'...
participant_id age sex hand
sub-01 n/a n/a n/a
Writing '/Users/hoechenberger/mne_data/MNE-sample-data/../MNE-sample-data-bids/participants.json'...
{
"participant_id": {
"Description": "Unique participant identifier"
},
"age": {
"Description": "Age of the participant at time of testing",
"Units": "years"
},
"sex": {
"Description": "Biological sex of the participant",
"Levels": {
"F": "female",
"M": "male"
}
},
"hand": {
"Description": "Handedness of the participant",
"Levels": {
"R": "right",
"L": "left",
"A": "ambidextrous"
}
}
}
Writing '/Users/hoechenberger/mne_data/MNE-sample-data/../MNE-sample-data-bids/sub-01/ses-01/meg/sub-01_ses-01_coordsystem.json'...
{
"MEGCoordinateSystem": "ElektaNeuromag",
"MEGCoordinateUnits": "m",
"MEGCoordinateSystemDescription": "RAS orientation and the origin between the ears",
"HeadCoilCoordinates": {
"NAS": [
3.725290298461914e-09,
0.10260561108589172,
4.190951585769653e-09
],
"LPA": [
-0.07137660682201385,
0.0,
5.122274160385132e-09
],
"RPA": [
0.07526767998933792,
0.0,
5.587935447692871e-09
],
"coil1": [
0.032922741025686264,
0.09897983074188232,
0.07984329760074615
],
"coil2": [
-0.06998106092214584,
0.06771647930145264,
0.06888450682163239
],
"coil3": [
-0.07260829955339432,
-0.02086828649044037,
0.0971473976969719
],
"coil4": [
0.04996863007545471,
-0.007233052980154753,
0.1228904277086258
]
},
"HeadCoilCoordinateSystem": "ElektaNeuromag",
"HeadCoilCoordinateUnits": "m"
}
Writing '/Users/hoechenberger/mne_data/MNE-sample-data/../MNE-sample-data-bids/sub-01/ses-01/meg/sub-01_ses-01_task-audiovisual_run-01_events.tsv'...
onset duration trial_type value sample
3.6246181587150867 0.0 Auditory/Right 2 2177
4.237323479067476 0.0 Visual/Left 5 2545
4.946596485779753 0.0 Auditory/Left 1 2971
5.692498614904401 0.0 Visual/Right 6 3419
6.41342634238425 0.0 Auditory/Right 2 3852
Writing '/Users/hoechenberger/mne_data/MNE-sample-data/../MNE-sample-data-bids/dataset_description.json'...
{
"Name": " ",
"BIDSVersion": "1.4.0",
"DatasetType": "raw",
"Authors": [
"Please cite MNE-BIDS in your publication before removing this (citations in README)"
]
}
Reading 0 ... 166799 = 0.000 ... 277.714 secs...
Writing '/Users/hoechenberger/mne_data/MNE-sample-data/../MNE-sample-data-bids/sub-01/ses-01/meg/sub-01_ses-01_task-audiovisual_run-01_meg.json'...
{
"TaskName": "audiovisual",
"Manufacturer": "Elekta",
"PowerLineFrequency": 60,
"SamplingFrequency": 600.614990234375,
"SoftwareFilters": "n/a",
"RecordingDuration": 277.7136813300495,
"RecordingType": "continuous",
"DewarPosition": "n/a",
"DigitizedLandmarks": false,
"DigitizedHeadPoints": false,
"MEGChannelCount": 306,
"MEGREFChannelCount": 0,
"EEGChannelCount": 60,
"EOGChannelCount": 1,
"ECGChannelCount": 0,
"EMGChannelCount": 0,
"MiscChannelCount": 0,
"TriggerChannelCount": 9
}
Writing '/Users/hoechenberger/mne_data/MNE-sample-data/../MNE-sample-data-bids/sub-01/ses-01/meg/sub-01_ses-01_task-audiovisual_run-01_channels.tsv'...
name type units low_cutoff high_cutoff description sampling_frequency status status_description
MEG 0113 MEGGRADPLANAR T/m 0.10000000149011612 172.17630004882812 Planar Gradiometer 600.614990234375 good n/a
MEG 0112 MEGGRADPLANAR T/m 0.10000000149011612 172.17630004882812 Planar Gradiometer 600.614990234375 good n/a
MEG 0111 MEGMAG T 0.10000000149011612 172.17630004882812 Magnetometer 600.614990234375 good n/a
MEG 0122 MEGGRADPLANAR T/m 0.10000000149011612 172.17630004882812 Planar Gradiometer 600.614990234375 good n/a
MEG 0123 MEGGRADPLANAR T/m 0.10000000149011612 172.17630004882812 Planar Gradiometer 600.614990234375 good n/a
Copying data files to sub-01_ses-01_task-audiovisual_run-01_meg.fif
Reserving possible split file sub-01_ses-01_task-audiovisual_run-01_split-01_meg.fif
Writing /Users/hoechenberger/mne_data/MNE-sample-data-bids/sub-01/ses-01/meg/sub-01_ses-01_task-audiovisual_run-01_meg.fif
Closing /Users/hoechenberger/mne_data/MNE-sample-data-bids/sub-01/ses-01/meg/sub-01_ses-01_task-audiovisual_run-01_meg.fif
[done]
Writing '/Users/hoechenberger/mne_data/MNE-sample-data/../MNE-sample-data-bids/sub-01/ses-01/sub-01_ses-01_scans.tsv'...
filename acq_time
meg/sub-01_ses-01_task-audiovisual_run-01_meg.fif 2002-12-03T19:01:10.720100Z
Wrote /Users/hoechenberger/mne_data/MNE-sample-data/../MNE-sample-data-bids/sub-01/ses-01/sub-01_ses-01_scans.tsv entry with meg/sub-01_ses-01_task-audiovisual_run-01_meg.fif.
BIDSPath(
root: /Users/hoechenberger/mne_data/MNE-sample-data/../MNE-sample-data-bids
datatype: meg
basename: sub-01_ses-01_task-audiovisual_run-01_meg.fif)
The sample MEG dataset comes with fine-calibration and crosstalk files that are required when processing Elekta/Neuromag/MEGIN data using MaxFilter®. Let’s store these data in appropriate places, too.
Out:
Writing fine-calibration file to /Users/hoechenberger/mne_data/MNE-sample-data/../MNE-sample-data-bids/sub-01/ses-01/meg/sub-01_ses-01_acq-calibration_meg.dat
Writing crosstalk file to /Users/hoechenberger/mne_data/MNE-sample-data/../MNE-sample-data-bids/sub-01/ses-01/meg/sub-01_ses-01_acq-crosstalk_meg.fif
Now let’s see the structure of the BIDS folder we created.
Out:
|MNE-sample-data-bids/
|--- README
|--- dataset_description.json
|--- participants.json
|--- participants.tsv
|--- sub-01/
|------ ses-01/
|--------- sub-01_ses-01_scans.tsv
|--------- meg/
|------------ sub-01_ses-01_acq-calibration_meg.dat
|------------ sub-01_ses-01_acq-crosstalk_meg.fif
|------------ sub-01_ses-01_coordsystem.json
|------------ sub-01_ses-01_task-audiovisual_run-01_channels.tsv
|------------ sub-01_ses-01_task-audiovisual_run-01_events.tsv
|------------ sub-01_ses-01_task-audiovisual_run-01_meg.fif
|------------ sub-01_ses-01_task-audiovisual_run-01_meg.json
Now let’s get an overview of the events on the whole dataset
audiovisual | ||||||||
---|---|---|---|---|---|---|---|---|
trial_type | Auditory/Left | Auditory/Right | Button | Smiley | Visual/Left | Visual/Right | ||
subject | session | run | ||||||
01 | 01 | 01 | 72 | 73 | 16 | 15 | 73 | 71 |
A big advantage of having data organized according to BIDS is that software
packages can automate your workflow. For example, reading the data back
into MNE-Python can easily be done using read_raw_bids()
.
Out:
Opening raw data file /Users/hoechenberger/mne_data/MNE-sample-data/../MNE-sample-data-bids/sub-01/ses-01/meg/sub-01_ses-01_task-audiovisual_run-01_meg.fif...
Read a total of 3 projection items:
PCA-v1 (1 x 102) idle
PCA-v2 (1 x 102) idle
PCA-v3 (1 x 102) idle
Range : 25800 ... 192599 = 42.956 ... 320.670 secs
Ready.
Reading events from /Users/hoechenberger/mne_data/MNE-sample-data/../MNE-sample-data-bids/sub-01/ses-01/meg/sub-01_ses-01_task-audiovisual_run-01_events.tsv.
Reading channel info from /Users/hoechenberger/mne_data/MNE-sample-data/../MNE-sample-data-bids/sub-01/ses-01/meg/sub-01_ses-01_task-audiovisual_run-01_channels.tsv.
/Users/hoechenberger/Development/mne-bids/mne_bids/read.py:339: RuntimeWarning: The unit for channel(s) STI 001, STI 002, STI 003, STI 004, STI 005, STI 006, STI 014, STI 015, STI 016 has changed from V to NA.
raw.set_channel_types(channel_type_dict)
The resulting data is already in a convenient form to create epochs and evoked data.
events, event_id = mne.events_from_annotations(raw)
epochs = mne.Epochs(raw, events, event_id)
epochs['Auditory'].average().plot()
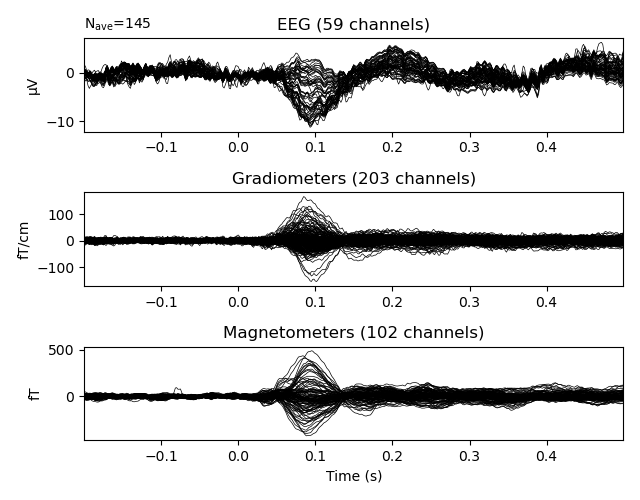
Out:
Used Annotations descriptions: ['Auditory/Left', 'Auditory/Right', 'Button', 'Smiley', 'Visual/Left', 'Visual/Right']
Not setting metadata
Not setting metadata
320 matching events found
Setting baseline interval to [-0.19979521315838786, 0.0] sec
Applying baseline correction (mode: mean)
Created an SSP operator (subspace dimension = 3)
3 projection items activated
<Figure size 640x500 with 3 Axes>
It is trivial to retrieve the path of the fine-calibration and crosstalk files, too.
print(bids_path.meg_calibration_fpath)
print(bids_path.meg_crosstalk_fpath)
Out:
/Users/hoechenberger/mne_data/MNE-sample-data/../MNE-sample-data-bids/sub-01/ses-01/meg/sub-01_ses-01_acq-calibration_meg.dat
/Users/hoechenberger/mne_data/MNE-sample-data/../MNE-sample-data-bids/sub-01/ses-01/meg/sub-01_ses-01_acq-crosstalk_meg.fif
The README created by write_raw_bids()
also takes care of the citation
for mne-bids. If you are preparing a manuscript, please make sure to also
cite MNE-BIDS there.
Out:
References
----------
Appelhoff, S., Sanderson, M., Brooks, T., Vliet, M., Quentin, R., Holdgraf, C., Chaumon, M., Mikulan, E., Tavabi, K., Höchenberger, R., Welke, D., Brunner, C., Rockhill, A., Larson, E., Gramfort, A. and Jas, M. (2019). MNE-BIDS: Organizing electrophysiological data into the BIDS format and facilitating their analysis. Journal of Open Source Software 4: (1896). https://doi.org/10.21105/joss.01896
Niso, G., Gorgolewski, K. J., Bock, E., Brooks, T. L., Flandin, G., Gramfort, A., Henson, R. N., Jas, M., Litvak, V., Moreau, J., Oostenveld, R., Schoffelen, J., Tadel, F., Wexler, J., Baillet, S. (2018). MEG-BIDS, the brain imaging data structure extended to magnetoencephalography. Scientific Data, 5, 180110. https://doi.org/10.1038/sdata.2018.110
Total running time of the script: ( 0 minutes 3.913 seconds)