Note
Click here to download the full example code
Compute induced power in the source space with dSPM¶
Returns STC files ie source estimates of induced power for different bands in the source space. The inverse method is linear based on dSPM inverse operator.
# Authors: Alexandre Gramfort <alexandre.gramfort@inria.fr>
#
# License: BSD (3-clause)
import matplotlib.pyplot as plt
import mne
from mne import io
from mne.datasets import sample
from mne.minimum_norm import read_inverse_operator, source_band_induced_power
print(__doc__)
Set parameters
data_path = sample.data_path()
raw_fname = data_path + '/MEG/sample/sample_audvis_raw.fif'
fname_inv = data_path + '/MEG/sample/sample_audvis-meg-oct-6-meg-inv.fif'
tmin, tmax, event_id = -0.2, 0.5, 1
# Setup for reading the raw data
raw = io.read_raw_fif(raw_fname)
events = mne.find_events(raw, stim_channel='STI 014')
inverse_operator = read_inverse_operator(fname_inv)
include = []
raw.info['bads'] += ['MEG 2443', 'EEG 053'] # bads + 2 more
# picks MEG gradiometers
picks = mne.pick_types(raw.info, meg=True, eeg=False, eog=True,
stim=False, include=include, exclude='bads')
# Load condition 1
event_id = 1
events = events[:10] # take 10 events to keep the computation time low
# Use linear detrend to reduce any edge artifacts
epochs = mne.Epochs(raw, events, event_id, tmin, tmax, picks=picks,
baseline=(None, 0), reject=dict(grad=4000e-13, eog=150e-6),
preload=True, detrend=1)
# Compute a source estimate per frequency band
bands = dict(alpha=[9, 11], beta=[18, 22])
stcs = source_band_induced_power(epochs, inverse_operator, bands, n_cycles=2,
use_fft=False, n_jobs=1)
for b, stc in stcs.items():
stc.save('induced_power_%s' % b)
Out:
Opening raw data file /home/circleci/mne_data/MNE-sample-data/MEG/sample/sample_audvis_raw.fif...
Read a total of 3 projection items:
PCA-v1 (1 x 102) idle
PCA-v2 (1 x 102) idle
PCA-v3 (1 x 102) idle
Range : 25800 ... 192599 = 42.956 ... 320.670 secs
Ready.
320 events found
Event IDs: [ 1 2 3 4 5 32]
Reading inverse operator decomposition from /home/circleci/mne_data/MNE-sample-data/MEG/sample/sample_audvis-meg-oct-6-meg-inv.fif...
Reading inverse operator info...
[done]
Reading inverse operator decomposition...
[done]
305 x 305 full covariance (kind = 1) found.
Read a total of 4 projection items:
PCA-v1 (1 x 102) active
PCA-v2 (1 x 102) active
PCA-v3 (1 x 102) active
Average EEG reference (1 x 60) active
Noise covariance matrix read.
22494 x 22494 diagonal covariance (kind = 2) found.
Source covariance matrix read.
22494 x 22494 diagonal covariance (kind = 6) found.
Orientation priors read.
22494 x 22494 diagonal covariance (kind = 5) found.
Depth priors read.
Did not find the desired covariance matrix (kind = 3)
Reading a source space...
Computing patch statistics...
Patch information added...
Distance information added...
[done]
Reading a source space...
Computing patch statistics...
Patch information added...
Distance information added...
[done]
2 source spaces read
Read a total of 4 projection items:
PCA-v1 (1 x 102) active
PCA-v2 (1 x 102) active
PCA-v3 (1 x 102) active
Average EEG reference (1 x 60) active
Source spaces transformed to the inverse solution coordinate frame
Not setting metadata
Not setting metadata
2 matching events found
Setting baseline interval to [-0.19979521315838786, 0.0] sec
Applying baseline correction (mode: mean)
Created an SSP operator (subspace dimension = 3)
3 projection items activated
Loading data for 2 events and 421 original time points ...
0 bad epochs dropped
Preparing the inverse operator for use...
Scaled noise and source covariance from nave = 1 to nave = 1
Created the regularized inverter
Created an SSP operator (subspace dimension = 3)
Created the whitener using a noise covariance matrix with rank 302 (3 small eigenvalues omitted)
Computing noise-normalization factors (dSPM)...
[done]
Picked 305 channels from the data
Computing inverse...
Eigenleads need to be weighted ...
Reducing data rank 305 -> 302
Computing source power ...
[done]
[done]
Writing STC to disk...
[done]
Writing STC to disk...
[done]
plot mean power
plt.plot(stcs['alpha'].times, stcs['alpha'].data.mean(axis=0), label='Alpha')
plt.plot(stcs['beta'].times, stcs['beta'].data.mean(axis=0), label='Beta')
plt.xlabel('Time (ms)')
plt.ylabel('Power')
plt.legend()
plt.title('Mean source induced power')
plt.show()
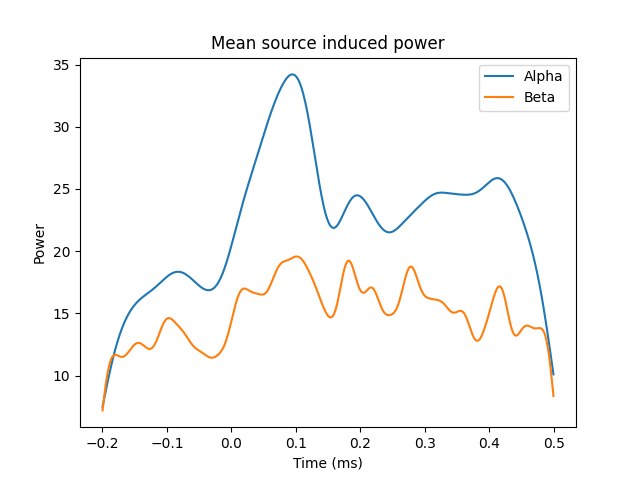
Total running time of the script: ( 0 minutes 8.364 seconds)
Estimated memory usage: 586 MB