Note
Go to the end to download the full example code.
Interactive data inspection and bad channel selection#
You can use MNE-BIDS interactively inspect your MEG or (i)EEG data. Problematic channels can be marked as “bad”, for example if the connected sensor produced mostly noise - or no signal at all. Similarly, you can declare channels as “good”, should you discover they were incorrectly marked as bad. Bad channel selection can also be performed non-interactively.
Furthermore, you can view and edit the experimental events and mark time segments as “bad”.
# Authors: The MNE-BIDS developers
# SPDX-License-Identifier: BSD-3-Clause
We will demonstrate how to mark individual channels as bad on the MNE “sample” dataset. After that, we will mark channels as good again.
Let’s start by importing the required modules and functions, reading the “sample” data, and writing it in the BIDS format.
import shutil
import mne
from mne_bids import (
BIDSPath,
inspect_dataset,
mark_channels,
read_raw_bids,
write_raw_bids,
)
data_path = mne.datasets.sample.data_path()
raw_fname = data_path / "MEG" / "sample" / "sample_audvis_raw.fif"
events_fname = data_path / "MEG" / "sample" / "sample_audvis_raw-eve.fif"
event_id = {
"Auditory/Left": 1,
"Auditory/Right": 2,
"Visual/Left": 3,
"Visual/Right": 4,
"Smiley": 5,
"Button": 32,
}
bids_root = data_path.parent / "MNE-sample-data-bids"
bids_path = BIDSPath(
subject="01", session="01", task="audiovisual", run="01", root=bids_root
)
To ensure the output path doesn’t contain any leftover files from previous tests and example runs, we simply delete it.
Warning
Do not delete directories that may contain important data!
Now write the raw data to BIDS.
raw = mne.io.read_raw_fif(raw_fname, verbose=False)
raw.info["line_freq"] = 60 # Specify power line frequency as required by BIDS.
write_raw_bids(
raw,
bids_path=bids_path,
events=events_fname,
event_id=event_id,
overwrite=True,
verbose=False,
)
BIDSPath(
root: /home/circleci/mne_data/MNE-sample-data-bids
datatype: meg
basename: sub-01_ses-01_task-audiovisual_run-01_meg.fif)
Interactive use#
Using mne_bids.inspect_dataset()
, we can interactively explore the raw
data and toggle the channel status – bad
or good
– by clicking on the
respective traces or channel names. If there are any SSP projectors stored
with the data, a small popup window will allow you to toggle the projectors
on and off. If you changed the selection of bad channels, you will be
prompted whether you would like to save the changes when closing the main
window. Your raw data and the *_channels.tsv sidecar file will be updated
upon saving.
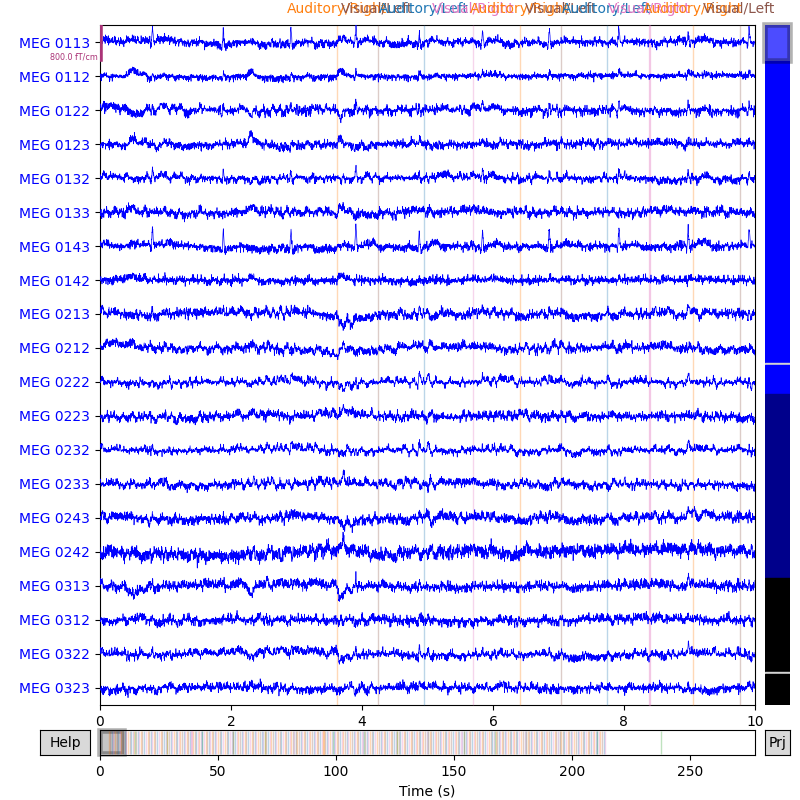
Reading 0 ... 166799 = 0.000 ... 277.714 secs...
Finding segments below or above PTP threshold.
You can even apply frequency filters when viewing the data: A high-pass filter can remove slow drifts, while a low-pass filter will get rid of high-frequency artifacts. This can make visual inspection easier. Let’s apply filters with a 1-Hz high-pass cutoff, and a 30-Hz low-pass cutoff:
inspect_dataset(bids_path, l_freq=1.0, h_freq=30.0)
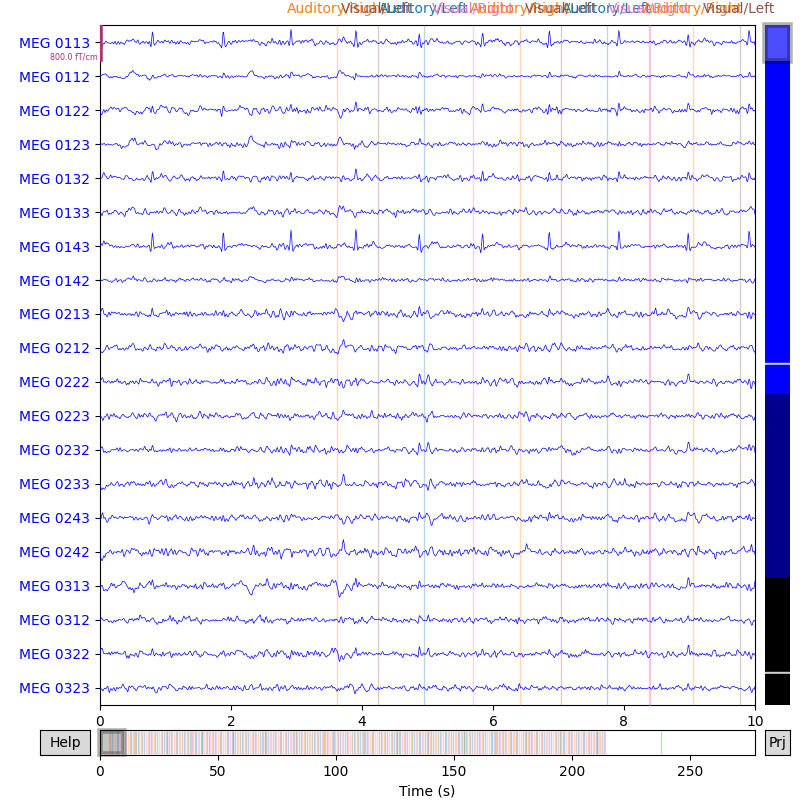
Reading 0 ... 166799 = 0.000 ... 277.714 secs...
Finding segments below or above PTP threshold.
By pressing the A
key, you can toggle annotation mode to add, edit, or
remove experimental events, or to mark entire time periods as bad. Please see
the MNE-Python Annotations tutorial for an introduction to the interactive
interface. If you’re closing the main window after changing the annotations,
you will be prompted whether you wish to save the changes. Your raw data and
the *_events.tsv sidecar file will be updated upon saving.
Non-interactive (programmatic) bad channel selection#
Read the (now BIDS-formatted) data and print a list of channels currently marked as bad.
raw = read_raw_bids(bids_path=bids_path, verbose=False)
print(
f"The following channels are currently marked as bad:\n"
f" {', '.join(raw.info['bads'])}\n"
)
The following channels are currently marked as bad:
MEG 2443, EEG 053
So currently, two channels are marked as bad: EEG 053
and MEG 2443
.
Let’s assume that through visual data inspection, we found that two more
MEG channels are problematic, and we would like to mark them as bad as well.
To do that, we simply add them to a list, which we then pass to
mne_bids.mark_channels()
:
bads = ["MEG 0112", "MEG 0131"]
mark_channels(bids_path=bids_path, ch_names=bads, status="bad", verbose=False)
That’s it! Let’s verify the result.
raw = read_raw_bids(bids_path=bids_path, verbose=False)
print(
f"After marking MEG 0112 and MEG 0131 as bad, the following channels "
f"are now marked as bad:\n {', '.join(raw.info['bads'])}\n"
)
After marking MEG 0112 and MEG 0131 as bad, the following channels are now marked as bad:
MEG 0112, MEG 0131, MEG 2443, EEG 053
As you can see, now a total of four channels is marked as bad: the ones
that were already bad when we started – EEG 053
and MEG 2443
– and
the two channels we passed to mne_bids.mark_channels()
–
MEG 0112
and MEG 0131
. This shows that marking bad channels via
mne_bids.mark_channels()
, by default, is an additive procedure,
which allows you to mark additional channels as bad while retaining the
information about all channels that had previously been marked as bad.
If you instead would like to replace the collection of bad channels
entirely, pass the argument overwrite=True
:
bads = ["MEG 0112", "MEG 0131"]
mark_channels(bids_path=bids_path, ch_names=bads, status="bad", verbose=False)
raw = read_raw_bids(bids_path=bids_path, verbose=False)
print(
f"After marking MEG 0112 and MEG 0131 as bad and passing "
f"`overwrite=True`, the following channels "
f"are now marked as bad:\n {', '.join(raw.info['bads'])}\n"
)
After marking MEG 0112 and MEG 0131 as bad and passing `overwrite=True`, the following channels are now marked as bad:
MEG 0112, MEG 0131, MEG 2443, EEG 053
Lastly, if you’re looking for a way to mark all channels as good, simply
pass ch_names="all"
, combined with overwrite=True
:
bads = "all"
mark_channels(bids_path=bids_path, ch_names=bads, status="bad", verbose=False)
raw = read_raw_bids(bids_path=bids_path, verbose=False)
print(
f"After passing `ch_names=[]` and `overwrite=True`, the following "
f"channels are now marked as bad:\n {', '.join(raw.info['bads'])}\n"
)
After passing `ch_names=[]` and `overwrite=True`, the following channels are now marked as bad:
MEG 0113, MEG 0112, MEG 0111, MEG 0122, MEG 0123, MEG 0121, MEG 0132, MEG 0133, MEG 0131, MEG 0143, MEG 0142, MEG 0141, MEG 0213, MEG 0212, MEG 0211, MEG 0222, MEG 0223, MEG 0221, MEG 0232, MEG 0233, MEG 0231, MEG 0243, MEG 0242, MEG 0241, MEG 0313, MEG 0312, MEG 0311, MEG 0322, MEG 0323, MEG 0321, MEG 0333, MEG 0332, MEG 0331, MEG 0343, MEG 0342, MEG 0341, MEG 0413, MEG 0412, MEG 0411, MEG 0422, MEG 0423, MEG 0421, MEG 0432, MEG 0433, MEG 0431, MEG 0443, MEG 0442, MEG 0441, MEG 0513, MEG 0512, MEG 0511, MEG 0523, MEG 0522, MEG 0521, MEG 0532, MEG 0533, MEG 0531, MEG 0542, MEG 0543, MEG 0541, MEG 0613, MEG 0612, MEG 0611, MEG 0622, MEG 0623, MEG 0621, MEG 0633, MEG 0632, MEG 0631, MEG 0642, MEG 0643, MEG 0641, MEG 0713, MEG 0712, MEG 0711, MEG 0723, MEG 0722, MEG 0721, MEG 0733, MEG 0732, MEG 0731, MEG 0743, MEG 0742, MEG 0741, MEG 0813, MEG 0812, MEG 0811, MEG 0822, MEG 0823, MEG 0821, MEG 0913, MEG 0912, MEG 0911, MEG 0923, MEG 0922, MEG 0921, MEG 0932, MEG 0933, MEG 0931, MEG 0942, MEG 0943, MEG 0941, MEG 1013, MEG 1012, MEG 1011, MEG 1023, MEG 1022, MEG 1021, MEG 1032, MEG 1033, MEG 1031, MEG 1043, MEG 1042, MEG 1041, MEG 1112, MEG 1113, MEG 1111, MEG 1123, MEG 1122, MEG 1121, MEG 1133, MEG 1132, MEG 1131, MEG 1142, MEG 1143, MEG 1141, MEG 1213, MEG 1212, MEG 1211, MEG 1223, MEG 1222, MEG 1221, MEG 1232, MEG 1233, MEG 1231, MEG 1243, MEG 1242, MEG 1241, MEG 1312, MEG 1313, MEG 1311, MEG 1323, MEG 1322, MEG 1321, MEG 1333, MEG 1332, MEG 1331, MEG 1342, MEG 1343, MEG 1341, MEG 1412, MEG 1413, MEG 1411, MEG 1423, MEG 1422, MEG 1421, MEG 1433, MEG 1432, MEG 1431, MEG 1442, MEG 1443, MEG 1441, MEG 1512, MEG 1513, MEG 1511, MEG 1522, MEG 1523, MEG 1521, MEG 1533, MEG 1532, MEG 1531, MEG 1543, MEG 1542, MEG 1541, MEG 1613, MEG 1612, MEG 1611, MEG 1622, MEG 1623, MEG 1621, MEG 1632, MEG 1633, MEG 1631, MEG 1643, MEG 1642, MEG 1641, MEG 1713, MEG 1712, MEG 1711, MEG 1722, MEG 1723, MEG 1721, MEG 1732, MEG 1733, MEG 1731, MEG 1743, MEG 1742, MEG 1741, MEG 1813, MEG 1812, MEG 1811, MEG 1822, MEG 1823, MEG 1821, MEG 1832, MEG 1833, MEG 1831, MEG 1843, MEG 1842, MEG 1841, MEG 1912, MEG 1913, MEG 1911, MEG 1923, MEG 1922, MEG 1921, MEG 1932, MEG 1933, MEG 1931, MEG 1943, MEG 1942, MEG 1941, MEG 2013, MEG 2012, MEG 2011, MEG 2023, MEG 2022, MEG 2021, MEG 2032, MEG 2033, MEG 2031, MEG 2042, MEG 2043, MEG 2041, MEG 2113, MEG 2112, MEG 2111, MEG 2122, MEG 2123, MEG 2121, MEG 2133, MEG 2132, MEG 2131, MEG 2143, MEG 2142, MEG 2141, MEG 2212, MEG 2213, MEG 2211, MEG 2223, MEG 2222, MEG 2221, MEG 2233, MEG 2232, MEG 2231, MEG 2242, MEG 2243, MEG 2241, MEG 2312, MEG 2313, MEG 2311, MEG 2323, MEG 2322, MEG 2321, MEG 2332, MEG 2333, MEG 2331, MEG 2343, MEG 2342, MEG 2341, MEG 2412, MEG 2413, MEG 2411, MEG 2423, MEG 2422, MEG 2421, MEG 2433, MEG 2432, MEG 2431, MEG 2442, MEG 2443, MEG 2441, MEG 2512, MEG 2513, MEG 2511, MEG 2522, MEG 2523, MEG 2521, MEG 2533, MEG 2532, MEG 2531, MEG 2543, MEG 2542, MEG 2541, MEG 2612, MEG 2613, MEG 2611, MEG 2623, MEG 2622, MEG 2621, MEG 2633, MEG 2632, MEG 2631, MEG 2642, MEG 2643, MEG 2641, STI 001, STI 002, STI 003, STI 004, STI 005, STI 006, STI 014, STI 015, STI 016, EEG 001, EEG 002, EEG 003, EEG 004, EEG 005, EEG 006, EEG 007, EEG 008, EEG 009, EEG 010, EEG 011, EEG 012, EEG 013, EEG 014, EEG 015, EEG 016, EEG 017, EEG 018, EEG 019, EEG 020, EEG 021, EEG 022, EEG 023, EEG 024, EEG 025, EEG 026, EEG 027, EEG 028, EEG 029, EEG 030, EEG 031, EEG 032, EEG 033, EEG 034, EEG 035, EEG 036, EEG 037, EEG 038, EEG 039, EEG 040, EEG 041, EEG 042, EEG 043, EEG 044, EEG 045, EEG 046, EEG 047, EEG 048, EEG 049, EEG 050, EEG 051, EEG 052, EEG 053, EEG 054, EEG 055, EEG 056, EEG 057, EEG 058, EEG 059, EEG 060, EOG 061
Total running time of the script: (0 minutes 12.688 seconds)