Note
Go to the end to download the full example code
EEG forward operator with a template MRI#
This tutorial explains how to compute the forward operator from EEG data
using the standard template MRI subject fsaverage
.
Caution
Source reconstruction without an individual T1 MRI from the subject will be less accurate. Do not over interpret activity locations which can be off by multiple centimeters.
Adult template MRI (fsaverage)#
First we show how fsaverage
can be used as a surrogate subject.
# Authors: Alexandre Gramfort <alexandre.gramfort@inria.fr>
# Joan Massich <mailsik@gmail.com>
# Eric Larson <larson.eric.d@gmail.com>
#
# License: BSD-3-Clause
import os.path as op
import numpy as np
import mne
from mne.datasets import eegbci
from mne.datasets import fetch_fsaverage
# Download fsaverage files
fs_dir = fetch_fsaverage(verbose=True)
subjects_dir = op.dirname(fs_dir)
# The files live in:
subject = "fsaverage"
trans = "fsaverage" # MNE has a built-in fsaverage transformation
src = op.join(fs_dir, "bem", "fsaverage-ico-5-src.fif")
bem = op.join(fs_dir, "bem", "fsaverage-5120-5120-5120-bem-sol.fif")
0 files missing from root.txt in /home/circleci/mne_data/MNE-fsaverage-data
0 files missing from bem.txt in /home/circleci/mne_data/MNE-fsaverage-data/fsaverage
Load the data#
We use here EEG data from the BCI dataset.
Note
See Plotting sensor layouts of EEG systems to view all the standard EEG montages available in MNE-Python.
(raw_fname,) = eegbci.load_data(subject=1, runs=[6])
raw = mne.io.read_raw_edf(raw_fname, preload=True)
# Clean channel names to be able to use a standard 1005 montage
new_names = dict(
(ch_name, ch_name.rstrip(".").upper().replace("Z", "z").replace("FP", "Fp"))
for ch_name in raw.ch_names
)
raw.rename_channels(new_names)
# Read and set the EEG electrode locations, which are already in fsaverage's
# space (MNI space) for standard_1020:
montage = mne.channels.make_standard_montage("standard_1005")
raw.set_montage(montage)
raw.set_eeg_reference(projection=True) # needed for inverse modeling
# Check that the locations of EEG electrodes is correct with respect to MRI
mne.viz.plot_alignment(
raw.info,
src=src,
eeg=["original", "projected"],
trans=trans,
show_axes=True,
mri_fiducials=True,
dig="fiducials",
)
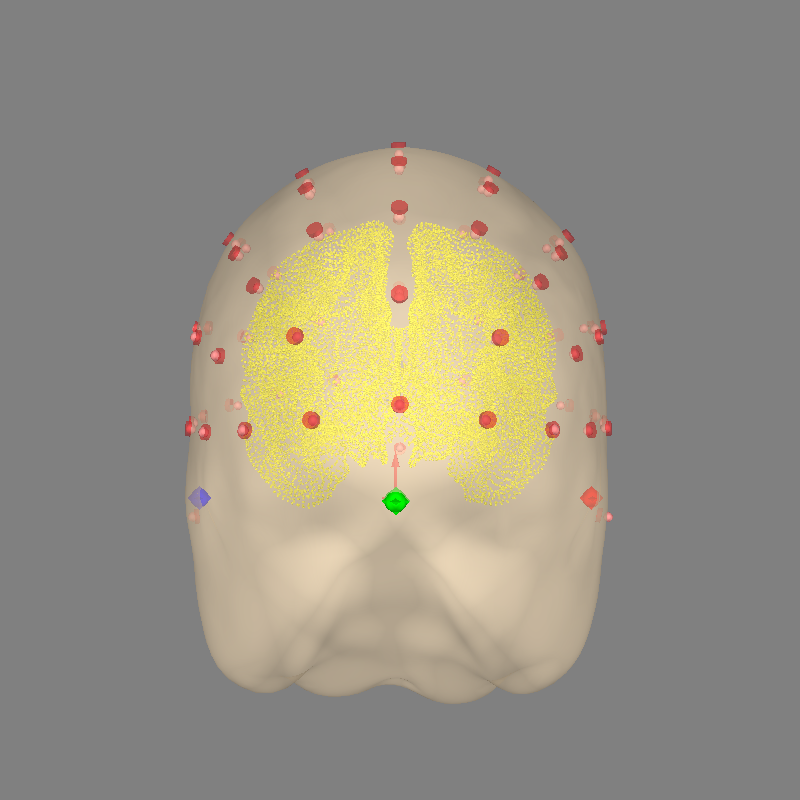
Extracting EDF parameters from /home/circleci/mne_data/MNE-eegbci-data/files/eegmmidb/1.0.0/S001/S001R06.edf...
EDF file detected
Setting channel info structure...
Creating raw.info structure...
Reading 0 ... 19999 = 0.000 ... 124.994 secs...
EEG channel type selected for re-referencing
Adding average EEG reference projection.
1 projection items deactivated
Average reference projection was added, but has not been applied yet. Use the apply_proj method to apply it.
Reading /home/circleci/mne_data/MNE-fsaverage-data/fsaverage/bem/fsaverage-ico-5-src.fif...
Using outer_skin.surf for head surface.
Channel types:: eeg: 64
Projecting sensors to the head surface
Setup source space and compute forward#
Source space : /home/circleci/mne_data/MNE-fsaverage-data/fsaverage/bem/fsaverage-ico-5-src.fif
MRI -> head transform : /home/circleci/project/mne/data/fsaverage/fsaverage-trans.fif
Measurement data : instance of Info
Conductor model : /home/circleci/mne_data/MNE-fsaverage-data/fsaverage/bem/fsaverage-5120-5120-5120-bem-sol.fif
Accurate field computations
Do computations in head coordinates
Free source orientations
Reading /home/circleci/mne_data/MNE-fsaverage-data/fsaverage/bem/fsaverage-ico-5-src.fif...
Read 2 source spaces a total of 20484 active source locations
Coordinate transformation: MRI (surface RAS) -> head
0.999994 0.003552 0.000202 -1.76 mm
-0.003558 0.998389 0.056626 31.09 mm
-0.000001 -0.056626 0.998395 39.60 mm
0.000000 0.000000 0.000000 1.00
Read 64 EEG channels from info
Head coordinate coil definitions created.
Source spaces are now in head coordinates.
Setting up the BEM model using /home/circleci/mne_data/MNE-fsaverage-data/fsaverage/bem/fsaverage-5120-5120-5120-bem-sol.fif...
Loading surfaces...
Loading the solution matrix...
Three-layer model surfaces loaded.
Loaded linear collocation BEM solution from /home/circleci/mne_data/MNE-fsaverage-data/fsaverage/bem/fsaverage-5120-5120-5120-bem-sol.fif
Employing the head->MRI coordinate transform with the BEM model.
BEM model fsaverage-5120-5120-5120-bem-sol.fif is now set up
Source spaces are in head coordinates.
Checking that the sources are inside the surface and at least 5.0 mm away (will take a few...)
Checking surface interior status for 10242 points...
Found 2433/10242 points inside an interior sphere of radius 47.7 mm
Found 0/10242 points outside an exterior sphere of radius 98.3 mm
Found 0/ 7809 points outside using surface Qhull
Found 0/ 7809 points outside using solid angles
Total 10242/10242 points inside the surface
Interior check completed in 4109.4 ms
Checking surface interior status for 10242 points...
Found 2241/10242 points inside an interior sphere of radius 47.7 mm
Found 0/10242 points outside an exterior sphere of radius 98.3 mm
Found 0/ 8001 points outside using surface Qhull
Found 0/ 8001 points outside using solid angles
Total 10242/10242 points inside the surface
Interior check completed in 4236.4 ms
Setting up for EEG...
Computing EEG at 20484 source locations (free orientations)...
Finished.
<Forward | MEG channels: 0 | EEG channels: 64 | Source space: Surface with 20484 vertices | Source orientation: Free>
From here on, standard inverse imaging methods can be used!
Infant MRI surrogates#
We don’t have a sample infant dataset for MNE, so let’s fake a 10-20 one:
ch_names = "Fz Cz Pz Oz Fp1 Fp2 F3 F4 F7 F8 C3 C4 T7 T8 P3 P4 P7 P8 O1 O2".split()
data = np.random.RandomState(0).randn(len(ch_names), 1000)
info = mne.create_info(ch_names, 1000.0, "eeg")
raw = mne.io.RawArray(data, info)
Creating RawArray with float64 data, n_channels=20, n_times=1000
Range : 0 ... 999 = 0.000 ... 0.999 secs
Ready.
Get an infant MRI template#
To use an infant head model for M/EEG data, you can use
mne.datasets.fetch_infant_template()
to download an infant template:
subject = mne.datasets.fetch_infant_template("6mo", subjects_dir, verbose=True)
0 files missing from ANTS6-0Months3T.txt in /home/circleci/mne_data/MNE-fsaverage-data/ANTS6-0Months3T
It comes with several helpful built-in files, including a 10-20 montage
in the MRI coordinate frame, which can be used to compute the
MRI<->head transform trans
:
fname_1020 = op.join(subjects_dir, subject, "montages", "10-20-montage.fif")
mon = mne.channels.read_dig_fif(fname_1020)
mon.rename_channels({f"EEG{ii:03d}": ch_name for ii, ch_name in enumerate(ch_names, 1)})
trans = mne.channels.compute_native_head_t(mon)
raw.set_montage(mon)
print(trans)
<Transform | MRI (surface RAS)->head>
[[ 0.99975431 -0.01839625 0.01236795 -0.00087239]
[ 0.0143472 0.96230429 0.27159646 0.01252444]
[-0.01689809 -0.27135229 0.96233177 0.03137219]
[ 0. 0. 0. 1. ]]
There are also BEM and source spaces:
Reading a source space...
Distance information added...
[done]
Reading a source space...
Distance information added...
[done]
2 source spaces read
<SourceSpaces: [<surface (lh), n_vertices=48640, n_used=4098>, <surface (rh), n_vertices=48214, n_used=4098>] MRI (surface RAS) coords, subject 'ANTS6-0Months3T', ~6.9 MB>
Loading surfaces...
Loading the solution matrix...
Three-layer model surfaces loaded.
Loaded linear collocation BEM solution from /home/circleci/mne_data/MNE-fsaverage-data/ANTS6-0Months3T/bem/ANTS6-0Months3T-5120-5120-5120-bem-sol.fif
You can ensure everything is as expected by plotting the result:
fig = mne.viz.plot_alignment(
raw.info,
subject=subject,
subjects_dir=subjects_dir,
trans=trans,
src=src,
bem=bem,
coord_frame="mri",
mri_fiducials=True,
show_axes=True,
surfaces=("white", "outer_skin", "inner_skull", "outer_skull"),
)
mne.viz.set_3d_view(fig, 25, 70, focalpoint=[0, -0.005, 0.01])
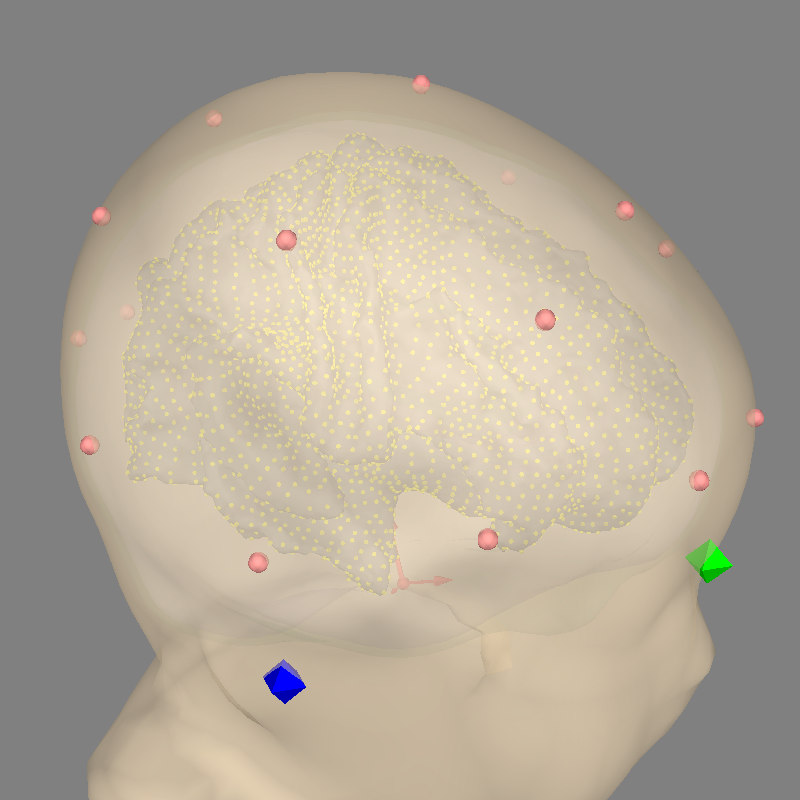
Channel types:: eeg: 20
From here, standard forward and inverse operators can be computed
If you have digitized head positions or MEG data, consider using mne coreg to warp a suitable infant template MRI to your digitization information.
Total running time of the script: (0 minutes 28.005 seconds)
Estimated memory usage: 678 MB