Note
Go to the end to download the full example code
Plotting eye-tracking heatmaps in MNE-Python#
This tutorial covers plotting eye-tracking position data as a heatmap.
# License: BSD-3-Clause
# Copyright the MNE-Python contributors.
Data loading#
As usual we start by importing the modules we need and loading some
example data: eye-tracking data recorded from SR research’s
'.asc'
file format.
import matplotlib.pyplot as plt
import mne
from mne.viz.eyetracking import plot_gaze
task_fpath = mne.datasets.eyelink.data_path() / "freeviewing"
et_fpath = task_fpath / "sub-01_task-freeview_eyetrack.asc"
stim_fpath = task_fpath / "stim" / "naturalistic.png"
raw = mne.io.read_raw_eyelink(et_fpath)
Loading /home/circleci/mne_data/MNE-eyelink-data/freeviewing/sub-01_task-freeview_eyetrack.asc
Pixel coordinate data detected.Pass `scalings=dict(eyegaze=1e3)` when using plot method to make traces more legible.
Pupil-size area detected.
There are 10 recording blocks in this file. Times between blocks will be annotated with BAD_ACQ_SKIP.
Process and epoch the data#
First we will interpolate missing data during blinks and epoch the data.
mne.preprocessing.eyetracking.interpolate_blinks(raw, interpolate_gaze=True)
raw.annotations.rename({"dvns": "natural"}) # more intuitive
event_ids = {"natural": 1}
events, event_dict = mne.events_from_annotations(raw, event_id=event_ids)
epochs = mne.Epochs(
raw, events=events, event_id=event_dict, tmin=0, tmax=20, baseline=None
)
Interpolating missing data during blinks...
Removing 'BAD_' from BAD_blink.
Used Annotations descriptions: ['natural']
Not setting metadata
5 matching events found
No baseline correction applied
0 projection items activated
Plot a heatmap of the eye-tracking data#
To make a heatmap of the eye-tracking data, we can use the function
plot_gaze()
. We will need to define the dimensions of our
canvas; for this file, the eye position data are reported in pixels, so we’ll use the
screen resolution of the participant screen (1920x1080) as the width and height. We
can also use the sigma parameter to smooth the plot.
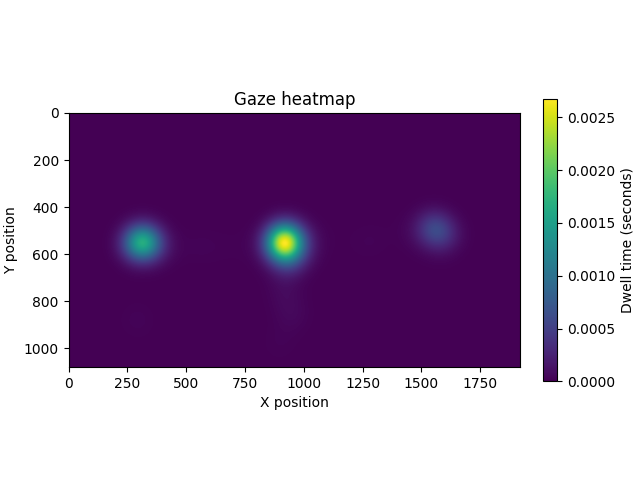
Using data from preloaded Raw for 5 events and 10001 original time points ...
0 bad epochs dropped
Overlaying plots with images#
We can use matplotlib to plot gaze heatmaps on top of stimuli images. We’ll
customize a Colormap
to make some values of the heatmap
completely transparent. We’ll then use the vlim
parameter to force the heatmap to
start at a value greater than the darkest value in our previous heatmap, which will
make the darkest colors of the heatmap transparent.
cmap.set_under("k", alpha=0) # make the lowest values transparent
ax = plt.subplot()
ax.imshow(plt.imread(stim_fpath))
plot_gaze(
epochs["natural"],
width=px_width,
height=px_height,
vlim=(0.0003, None),
sigma=50,
cmap=cmap,
axes=ax,
)
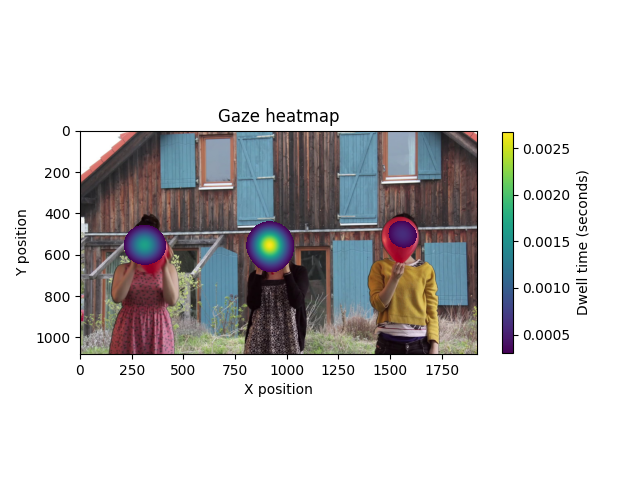
Using data from preloaded Raw for 5 events and 10001 original time points ...
0 bad epochs dropped
Total running time of the script: (0 minutes 7.965 seconds)
Estimated memory usage: 84 MB