Note
Go to the end to download the full example code.
Decoding real-time data๐
This example demonstrates how to decode real-time data using MNE-Python
and Scikit-learn. We will stream the sample_audvis_raw.fif
file from MNEโs sample dataset with a PlayerLSL
, process the
signal through a StreamLSL
, and decode the epochs created with
EpochsStream
.
import time
import uuid
import numpy as np
from matplotlib import pyplot as plt
from mne.decoding import Vectorizer
from mne.io import read_raw_fif
from sklearn.linear_model import LogisticRegression
from sklearn.model_selection import ShuffleSplit, cross_val_score
from sklearn.pipeline import Pipeline
from sklearn.preprocessing import StandardScaler
from mne_lsl.datasets import sample
from mne_lsl.player import PlayerLSL
from mne_lsl.stream import EpochsStream, StreamLSL
fname = sample.data_path() / "mne-sample" / "sample_audvis_raw.fif"
raw = read_raw_fif(fname, preload=False).pick(("meg", "stim")).load_data()
source_id = uuid.uuid4().hex
player = PlayerLSL(raw, chunk_size=200, name="real-time-decoding", source_id=source_id)
player.start()
player.info
Signal processing๐
We will apply minimal signal processing to the data. First, only the gradiometers will be used for decoding, thus other channels are removed. Then we mark bad channels and applying a low-pass filter at 40 Hz.
stream = StreamLSL(bufsize=5, name="real-time-decoding", source_id=source_id)
stream.connect(acquisition_delay=0.1, processing_flags="all")
stream.info["bads"] = ["MEG 2443"]
stream.pick(("grad", "stim")).filter(None, 40, picks="grad")
stream.info
Epoch the signal๐
Next, we will create epochs around the event 1
(audio left) and 3
(visual
left).
epochs = EpochsStream(
stream,
bufsize=10,
event_id=dict(audio_left=1, visual_left=3),
event_channels="STI 014",
tmin=-0.2,
tmax=0.5,
baseline=(None, 0),
reject=dict(grad=4000e-13), # unit: T / m (gradiometers)
).connect(acquisition_delay=0.1)
epochs.info
Define the classifier๐
We will use a LogisticRegression
classifier to decode
the epochs.
Note
The object Vectorizer
is used to transform the epochs in a
2D array of shape (n_epochs, n_features). Itโs simply reshapes the epochs data
with:
data = epochs.get_data()
data = data.reshape(data.shape[0], -1)
vectorizer = Vectorizer()
scaler = StandardScaler()
clf = LogisticRegression()
classifier = Pipeline([("vector", vectorizer), ("scaler", scaler), ("svm", clf)])
Decode๐
First, we will wait for a minimum number of epochs to be available. Then, the classifier will be trained for the first time and future epochs will be used to retrain the classifier every 5 epochs.
min_epochs = 10
while epochs.n_new_epochs < min_epochs:
time.sleep(0.5)
# prepare figure to plot classifiation score
if not plt.isinteractive():
plt.ion()
fig, ax = plt.subplots()
ax.set_xlabel("Epochsn nยฐ")
ax.set_ylabel("Classification score (% correct)")
ax.set_title("Real-time decoding")
ax.set_xlim([min_epochs, 50])
ax.set_ylim([30, 105])
ax.axhline(50, color="k", linestyle="--", label="Chance level")
plt.show()
# decoding loop
scores_x, scores, std_scores = [], [], []
while True:
if len(scores_x) != 0 and 50 <= scores_x[-1]:
break
n_epochs = epochs.n_new_epochs
if n_epochs == 0 or n_epochs % 5 != 0:
time.sleep(0.5) # give time to the streaming and acquisition threads
continue
if len(scores_x) == 0: # first training
X = epochs.get_data(n_epochs=n_epochs)
y = epochs.events[-n_epochs:]
else:
X = np.concatenate((X, epochs.get_data(n_epochs=n_epochs)), axis=0)
y = np.concatenate((y, epochs.events[-n_epochs:]))
cv = ShuffleSplit(5, test_size=0.2, random_state=42)
scores_t = cross_val_score(classifier, X, y, cv=cv, n_jobs=1) * 100
std_scores.append(scores_t.std())
scores.append(scores_t.mean())
scores_x.append(scores_x[-1] + n_epochs if len(scores_x) != 0 else n_epochs)
# update figure
ax.plot(scores_x[-2:], scores[-2:], "-x", color="b")
hyp_limits = (
np.asarray(scores[-2:]) - np.asarray(std_scores[-2:]),
np.asarray(scores[-2:]) + np.asarray(std_scores[-2:]),
)
fill = ax.fill_between(
scores_x[-2:], y1=hyp_limits[0], y2=hyp_limits[1], color="b", alpha=0.5
)
plt.pause(0.1)
plt.draw()
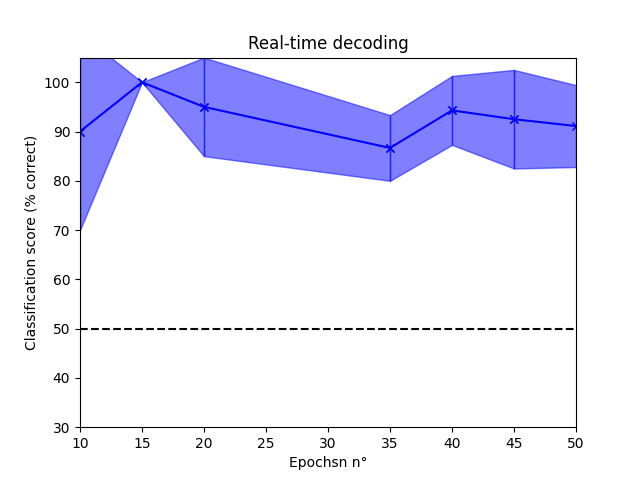
/opt/hostedtoolcache/Python/3.13.0/x64/lib/python3.13/site-packages/sklearn/utils/_tags.py:322: FutureWarning: Estimator <mne.decoding.transformer.Vectorizer object at 0x7f72d23bf8c0> has no __sklearn_tags__ attribute, which is defined in `sklearn.base.BaseEstimator`. This will raise an error in scikit-learn 1.8. Please define the __sklearn_tags__ method, or inherit from `sklearn.base.BaseEstimator` and other appropriate mixins such as `sklearn.base.TransformerMixin`, `sklearn.base.ClassifierMixin`, `sklearn.base.RegressorMixin`, and `sklearn.base.ClusterMixin`, and `sklearn.base.OutlierMixin`.
warnings.warn(
/opt/hostedtoolcache/Python/3.13.0/x64/lib/python3.13/site-packages/sklearn/utils/_tags.py:322: FutureWarning: Estimator <mne.decoding.transformer.Vectorizer object at 0x7f72d3c9e710> has no __sklearn_tags__ attribute, which is defined in `sklearn.base.BaseEstimator`. This will raise an error in scikit-learn 1.8. Please define the __sklearn_tags__ method, or inherit from `sklearn.base.BaseEstimator` and other appropriate mixins such as `sklearn.base.TransformerMixin`, `sklearn.base.ClassifierMixin`, `sklearn.base.RegressorMixin`, and `sklearn.base.ClusterMixin`, and `sklearn.base.OutlierMixin`.
warnings.warn(
/opt/hostedtoolcache/Python/3.13.0/x64/lib/python3.13/site-packages/sklearn/utils/_tags.py:322: FutureWarning: Estimator <mne.decoding.transformer.Vectorizer object at 0x7f72d3c9e710> has no __sklearn_tags__ attribute, which is defined in `sklearn.base.BaseEstimator`. This will raise an error in scikit-learn 1.8. Please define the __sklearn_tags__ method, or inherit from `sklearn.base.BaseEstimator` and other appropriate mixins such as `sklearn.base.TransformerMixin`, `sklearn.base.ClassifierMixin`, `sklearn.base.RegressorMixin`, and `sklearn.base.ClusterMixin`, and `sklearn.base.OutlierMixin`.
warnings.warn(
/opt/hostedtoolcache/Python/3.13.0/x64/lib/python3.13/site-packages/sklearn/utils/_tags.py:322: FutureWarning: Estimator <mne.decoding.transformer.Vectorizer object at 0x7f72d3c9ee90> has no __sklearn_tags__ attribute, which is defined in `sklearn.base.BaseEstimator`. This will raise an error in scikit-learn 1.8. Please define the __sklearn_tags__ method, or inherit from `sklearn.base.BaseEstimator` and other appropriate mixins such as `sklearn.base.TransformerMixin`, `sklearn.base.ClassifierMixin`, `sklearn.base.RegressorMixin`, and `sklearn.base.ClusterMixin`, and `sklearn.base.OutlierMixin`.
warnings.warn(
/opt/hostedtoolcache/Python/3.13.0/x64/lib/python3.13/site-packages/sklearn/utils/_tags.py:322: FutureWarning: Estimator <mne.decoding.transformer.Vectorizer object at 0x7f72d3c9ee90> has no __sklearn_tags__ attribute, which is defined in `sklearn.base.BaseEstimator`. This will raise an error in scikit-learn 1.8. Please define the __sklearn_tags__ method, or inherit from `sklearn.base.BaseEstimator` and other appropriate mixins such as `sklearn.base.TransformerMixin`, `sklearn.base.ClassifierMixin`, `sklearn.base.RegressorMixin`, and `sklearn.base.ClusterMixin`, and `sklearn.base.OutlierMixin`.
warnings.warn(
/opt/hostedtoolcache/Python/3.13.0/x64/lib/python3.13/site-packages/sklearn/utils/_tags.py:322: FutureWarning: Estimator <mne.decoding.transformer.Vectorizer object at 0x7f72d3d08c30> has no __sklearn_tags__ attribute, which is defined in `sklearn.base.BaseEstimator`. This will raise an error in scikit-learn 1.8. Please define the __sklearn_tags__ method, or inherit from `sklearn.base.BaseEstimator` and other appropriate mixins such as `sklearn.base.TransformerMixin`, `sklearn.base.ClassifierMixin`, `sklearn.base.RegressorMixin`, and `sklearn.base.ClusterMixin`, and `sklearn.base.OutlierMixin`.
warnings.warn(
/opt/hostedtoolcache/Python/3.13.0/x64/lib/python3.13/site-packages/sklearn/utils/_tags.py:322: FutureWarning: Estimator <mne.decoding.transformer.Vectorizer object at 0x7f72d3d08c30> has no __sklearn_tags__ attribute, which is defined in `sklearn.base.BaseEstimator`. This will raise an error in scikit-learn 1.8. Please define the __sklearn_tags__ method, or inherit from `sklearn.base.BaseEstimator` and other appropriate mixins such as `sklearn.base.TransformerMixin`, `sklearn.base.ClassifierMixin`, `sklearn.base.RegressorMixin`, and `sklearn.base.ClusterMixin`, and `sklearn.base.OutlierMixin`.
warnings.warn(
/opt/hostedtoolcache/Python/3.13.0/x64/lib/python3.13/site-packages/sklearn/utils/_tags.py:322: FutureWarning: Estimator <mne.decoding.transformer.Vectorizer object at 0x7f72d3d0afd0> has no __sklearn_tags__ attribute, which is defined in `sklearn.base.BaseEstimator`. This will raise an error in scikit-learn 1.8. Please define the __sklearn_tags__ method, or inherit from `sklearn.base.BaseEstimator` and other appropriate mixins such as `sklearn.base.TransformerMixin`, `sklearn.base.ClassifierMixin`, `sklearn.base.RegressorMixin`, and `sklearn.base.ClusterMixin`, and `sklearn.base.OutlierMixin`.
warnings.warn(
/opt/hostedtoolcache/Python/3.13.0/x64/lib/python3.13/site-packages/sklearn/utils/_tags.py:322: FutureWarning: Estimator <mne.decoding.transformer.Vectorizer object at 0x7f72d3d0afd0> has no __sklearn_tags__ attribute, which is defined in `sklearn.base.BaseEstimator`. This will raise an error in scikit-learn 1.8. Please define the __sklearn_tags__ method, or inherit from `sklearn.base.BaseEstimator` and other appropriate mixins such as `sklearn.base.TransformerMixin`, `sklearn.base.ClassifierMixin`, `sklearn.base.RegressorMixin`, and `sklearn.base.ClusterMixin`, and `sklearn.base.OutlierMixin`.
warnings.warn(
/opt/hostedtoolcache/Python/3.13.0/x64/lib/python3.13/site-packages/sklearn/utils/_tags.py:322: FutureWarning: Estimator <mne.decoding.transformer.Vectorizer object at 0x7f72d3c10a70> has no __sklearn_tags__ attribute, which is defined in `sklearn.base.BaseEstimator`. This will raise an error in scikit-learn 1.8. Please define the __sklearn_tags__ method, or inherit from `sklearn.base.BaseEstimator` and other appropriate mixins such as `sklearn.base.TransformerMixin`, `sklearn.base.ClassifierMixin`, `sklearn.base.RegressorMixin`, and `sklearn.base.ClusterMixin`, and `sklearn.base.OutlierMixin`.
warnings.warn(
/opt/hostedtoolcache/Python/3.13.0/x64/lib/python3.13/site-packages/sklearn/utils/_tags.py:322: FutureWarning: Estimator <mne.decoding.transformer.Vectorizer object at 0x7f72d3c10a70> has no __sklearn_tags__ attribute, which is defined in `sklearn.base.BaseEstimator`. This will raise an error in scikit-learn 1.8. Please define the __sklearn_tags__ method, or inherit from `sklearn.base.BaseEstimator` and other appropriate mixins such as `sklearn.base.TransformerMixin`, `sklearn.base.ClassifierMixin`, `sklearn.base.RegressorMixin`, and `sklearn.base.ClusterMixin`, and `sklearn.base.OutlierMixin`.
warnings.warn(
/opt/hostedtoolcache/Python/3.13.0/x64/lib/python3.13/site-packages/sklearn/utils/_tags.py:322: FutureWarning: Estimator <mne.decoding.transformer.Vectorizer object at 0x7f72d3c10a70> has no __sklearn_tags__ attribute, which is defined in `sklearn.base.BaseEstimator`. This will raise an error in scikit-learn 1.8. Please define the __sklearn_tags__ method, or inherit from `sklearn.base.BaseEstimator` and other appropriate mixins such as `sklearn.base.TransformerMixin`, `sklearn.base.ClassifierMixin`, `sklearn.base.RegressorMixin`, and `sklearn.base.ClusterMixin`, and `sklearn.base.OutlierMixin`.
warnings.warn(
/opt/hostedtoolcache/Python/3.13.0/x64/lib/python3.13/site-packages/sklearn/utils/_tags.py:322: FutureWarning: Estimator <mne.decoding.transformer.Vectorizer object at 0x7f72d23bf8c0> has no __sklearn_tags__ attribute, which is defined in `sklearn.base.BaseEstimator`. This will raise an error in scikit-learn 1.8. Please define the __sklearn_tags__ method, or inherit from `sklearn.base.BaseEstimator` and other appropriate mixins such as `sklearn.base.TransformerMixin`, `sklearn.base.ClassifierMixin`, `sklearn.base.RegressorMixin`, and `sklearn.base.ClusterMixin`, and `sklearn.base.OutlierMixin`.
warnings.warn(
/opt/hostedtoolcache/Python/3.13.0/x64/lib/python3.13/site-packages/sklearn/utils/_tags.py:322: FutureWarning: Estimator <mne.decoding.transformer.Vectorizer object at 0x7f72d3c548d0> has no __sklearn_tags__ attribute, which is defined in `sklearn.base.BaseEstimator`. This will raise an error in scikit-learn 1.8. Please define the __sklearn_tags__ method, or inherit from `sklearn.base.BaseEstimator` and other appropriate mixins such as `sklearn.base.TransformerMixin`, `sklearn.base.ClassifierMixin`, `sklearn.base.RegressorMixin`, and `sklearn.base.ClusterMixin`, and `sklearn.base.OutlierMixin`.
warnings.warn(
/opt/hostedtoolcache/Python/3.13.0/x64/lib/python3.13/site-packages/sklearn/utils/_tags.py:322: FutureWarning: Estimator <mne.decoding.transformer.Vectorizer object at 0x7f72d3c548d0> has no __sklearn_tags__ attribute, which is defined in `sklearn.base.BaseEstimator`. This will raise an error in scikit-learn 1.8. Please define the __sklearn_tags__ method, or inherit from `sklearn.base.BaseEstimator` and other appropriate mixins such as `sklearn.base.TransformerMixin`, `sklearn.base.ClassifierMixin`, `sklearn.base.RegressorMixin`, and `sklearn.base.ClusterMixin`, and `sklearn.base.OutlierMixin`.
warnings.warn(
/opt/hostedtoolcache/Python/3.13.0/x64/lib/python3.13/site-packages/sklearn/utils/_tags.py:322: FutureWarning: Estimator <mne.decoding.transformer.Vectorizer object at 0x7f72d3c54af0> has no __sklearn_tags__ attribute, which is defined in `sklearn.base.BaseEstimator`. This will raise an error in scikit-learn 1.8. Please define the __sklearn_tags__ method, or inherit from `sklearn.base.BaseEstimator` and other appropriate mixins such as `sklearn.base.TransformerMixin`, `sklearn.base.ClassifierMixin`, `sklearn.base.RegressorMixin`, and `sklearn.base.ClusterMixin`, and `sklearn.base.OutlierMixin`.
warnings.warn(
/opt/hostedtoolcache/Python/3.13.0/x64/lib/python3.13/site-packages/sklearn/utils/_tags.py:322: FutureWarning: Estimator <mne.decoding.transformer.Vectorizer object at 0x7f72d3c54af0> has no __sklearn_tags__ attribute, which is defined in `sklearn.base.BaseEstimator`. This will raise an error in scikit-learn 1.8. Please define the __sklearn_tags__ method, or inherit from `sklearn.base.BaseEstimator` and other appropriate mixins such as `sklearn.base.TransformerMixin`, `sklearn.base.ClassifierMixin`, `sklearn.base.RegressorMixin`, and `sklearn.base.ClusterMixin`, and `sklearn.base.OutlierMixin`.
warnings.warn(
/opt/hostedtoolcache/Python/3.13.0/x64/lib/python3.13/site-packages/sklearn/utils/_tags.py:322: FutureWarning: Estimator <mne.decoding.transformer.Vectorizer object at 0x7f72d3d72a50> has no __sklearn_tags__ attribute, which is defined in `sklearn.base.BaseEstimator`. This will raise an error in scikit-learn 1.8. Please define the __sklearn_tags__ method, or inherit from `sklearn.base.BaseEstimator` and other appropriate mixins such as `sklearn.base.TransformerMixin`, `sklearn.base.ClassifierMixin`, `sklearn.base.RegressorMixin`, and `sklearn.base.ClusterMixin`, and `sklearn.base.OutlierMixin`.
warnings.warn(
/opt/hostedtoolcache/Python/3.13.0/x64/lib/python3.13/site-packages/sklearn/utils/_tags.py:322: FutureWarning: Estimator <mne.decoding.transformer.Vectorizer object at 0x7f72d3d72a50> has no __sklearn_tags__ attribute, which is defined in `sklearn.base.BaseEstimator`. This will raise an error in scikit-learn 1.8. Please define the __sklearn_tags__ method, or inherit from `sklearn.base.BaseEstimator` and other appropriate mixins such as `sklearn.base.TransformerMixin`, `sklearn.base.ClassifierMixin`, `sklearn.base.RegressorMixin`, and `sklearn.base.ClusterMixin`, and `sklearn.base.OutlierMixin`.
warnings.warn(
/opt/hostedtoolcache/Python/3.13.0/x64/lib/python3.13/site-packages/sklearn/utils/_tags.py:322: FutureWarning: Estimator <mne.decoding.transformer.Vectorizer object at 0x7f72d3d73050> has no __sklearn_tags__ attribute, which is defined in `sklearn.base.BaseEstimator`. This will raise an error in scikit-learn 1.8. Please define the __sklearn_tags__ method, or inherit from `sklearn.base.BaseEstimator` and other appropriate mixins such as `sklearn.base.TransformerMixin`, `sklearn.base.ClassifierMixin`, `sklearn.base.RegressorMixin`, and `sklearn.base.ClusterMixin`, and `sklearn.base.OutlierMixin`.
warnings.warn(
/opt/hostedtoolcache/Python/3.13.0/x64/lib/python3.13/site-packages/sklearn/utils/_tags.py:322: FutureWarning: Estimator <mne.decoding.transformer.Vectorizer object at 0x7f72d3d73050> has no __sklearn_tags__ attribute, which is defined in `sklearn.base.BaseEstimator`. This will raise an error in scikit-learn 1.8. Please define the __sklearn_tags__ method, or inherit from `sklearn.base.BaseEstimator` and other appropriate mixins such as `sklearn.base.TransformerMixin`, `sklearn.base.ClassifierMixin`, `sklearn.base.RegressorMixin`, and `sklearn.base.ClusterMixin`, and `sklearn.base.OutlierMixin`.
warnings.warn(
/opt/hostedtoolcache/Python/3.13.0/x64/lib/python3.13/site-packages/sklearn/utils/_tags.py:322: FutureWarning: Estimator <mne.decoding.transformer.Vectorizer object at 0x7f72d26906e0> has no __sklearn_tags__ attribute, which is defined in `sklearn.base.BaseEstimator`. This will raise an error in scikit-learn 1.8. Please define the __sklearn_tags__ method, or inherit from `sklearn.base.BaseEstimator` and other appropriate mixins such as `sklearn.base.TransformerMixin`, `sklearn.base.ClassifierMixin`, `sklearn.base.RegressorMixin`, and `sklearn.base.ClusterMixin`, and `sklearn.base.OutlierMixin`.
warnings.warn(
/opt/hostedtoolcache/Python/3.13.0/x64/lib/python3.13/site-packages/sklearn/utils/_tags.py:322: FutureWarning: Estimator <mne.decoding.transformer.Vectorizer object at 0x7f72d26906e0> has no __sklearn_tags__ attribute, which is defined in `sklearn.base.BaseEstimator`. This will raise an error in scikit-learn 1.8. Please define the __sklearn_tags__ method, or inherit from `sklearn.base.BaseEstimator` and other appropriate mixins such as `sklearn.base.TransformerMixin`, `sklearn.base.ClassifierMixin`, `sklearn.base.RegressorMixin`, and `sklearn.base.ClusterMixin`, and `sklearn.base.OutlierMixin`.
warnings.warn(
/opt/hostedtoolcache/Python/3.13.0/x64/lib/python3.13/site-packages/sklearn/utils/_tags.py:322: FutureWarning: Estimator <mne.decoding.transformer.Vectorizer object at 0x7f72d23bf8c0> has no __sklearn_tags__ attribute, which is defined in `sklearn.base.BaseEstimator`. This will raise an error in scikit-learn 1.8. Please define the __sklearn_tags__ method, or inherit from `sklearn.base.BaseEstimator` and other appropriate mixins such as `sklearn.base.TransformerMixin`, `sklearn.base.ClassifierMixin`, `sklearn.base.RegressorMixin`, and `sklearn.base.ClusterMixin`, and `sklearn.base.OutlierMixin`.
warnings.warn(
/opt/hostedtoolcache/Python/3.13.0/x64/lib/python3.13/site-packages/sklearn/utils/_tags.py:322: FutureWarning: Estimator <mne.decoding.transformer.Vectorizer object at 0x7f72d2692990> has no __sklearn_tags__ attribute, which is defined in `sklearn.base.BaseEstimator`. This will raise an error in scikit-learn 1.8. Please define the __sklearn_tags__ method, or inherit from `sklearn.base.BaseEstimator` and other appropriate mixins such as `sklearn.base.TransformerMixin`, `sklearn.base.ClassifierMixin`, `sklearn.base.RegressorMixin`, and `sklearn.base.ClusterMixin`, and `sklearn.base.OutlierMixin`.
warnings.warn(
/opt/hostedtoolcache/Python/3.13.0/x64/lib/python3.13/site-packages/sklearn/utils/_tags.py:322: FutureWarning: Estimator <mne.decoding.transformer.Vectorizer object at 0x7f72d2692990> has no __sklearn_tags__ attribute, which is defined in `sklearn.base.BaseEstimator`. This will raise an error in scikit-learn 1.8. Please define the __sklearn_tags__ method, or inherit from `sklearn.base.BaseEstimator` and other appropriate mixins such as `sklearn.base.TransformerMixin`, `sklearn.base.ClassifierMixin`, `sklearn.base.RegressorMixin`, and `sklearn.base.ClusterMixin`, and `sklearn.base.OutlierMixin`.
warnings.warn(
/opt/hostedtoolcache/Python/3.13.0/x64/lib/python3.13/site-packages/sklearn/utils/_tags.py:322: FutureWarning: Estimator <mne.decoding.transformer.Vectorizer object at 0x7f72d26d8d70> has no __sklearn_tags__ attribute, which is defined in `sklearn.base.BaseEstimator`. This will raise an error in scikit-learn 1.8. Please define the __sklearn_tags__ method, or inherit from `sklearn.base.BaseEstimator` and other appropriate mixins such as `sklearn.base.TransformerMixin`, `sklearn.base.ClassifierMixin`, `sklearn.base.RegressorMixin`, and `sklearn.base.ClusterMixin`, and `sklearn.base.OutlierMixin`.
warnings.warn(
/opt/hostedtoolcache/Python/3.13.0/x64/lib/python3.13/site-packages/sklearn/utils/_tags.py:322: FutureWarning: Estimator <mne.decoding.transformer.Vectorizer object at 0x7f72d26d8d70> has no __sklearn_tags__ attribute, which is defined in `sklearn.base.BaseEstimator`. This will raise an error in scikit-learn 1.8. Please define the __sklearn_tags__ method, or inherit from `sklearn.base.BaseEstimator` and other appropriate mixins such as `sklearn.base.TransformerMixin`, `sklearn.base.ClassifierMixin`, `sklearn.base.RegressorMixin`, and `sklearn.base.ClusterMixin`, and `sklearn.base.OutlierMixin`.
warnings.warn(
/opt/hostedtoolcache/Python/3.13.0/x64/lib/python3.13/site-packages/sklearn/utils/_tags.py:322: FutureWarning: Estimator <mne.decoding.transformer.Vectorizer object at 0x7f72d26d90f0> has no __sklearn_tags__ attribute, which is defined in `sklearn.base.BaseEstimator`. This will raise an error in scikit-learn 1.8. Please define the __sklearn_tags__ method, or inherit from `sklearn.base.BaseEstimator` and other appropriate mixins such as `sklearn.base.TransformerMixin`, `sklearn.base.ClassifierMixin`, `sklearn.base.RegressorMixin`, and `sklearn.base.ClusterMixin`, and `sklearn.base.OutlierMixin`.
warnings.warn(
/opt/hostedtoolcache/Python/3.13.0/x64/lib/python3.13/site-packages/sklearn/utils/_tags.py:322: FutureWarning: Estimator <mne.decoding.transformer.Vectorizer object at 0x7f72d26d90f0> has no __sklearn_tags__ attribute, which is defined in `sklearn.base.BaseEstimator`. This will raise an error in scikit-learn 1.8. Please define the __sklearn_tags__ method, or inherit from `sklearn.base.BaseEstimator` and other appropriate mixins such as `sklearn.base.TransformerMixin`, `sklearn.base.ClassifierMixin`, `sklearn.base.RegressorMixin`, and `sklearn.base.ClusterMixin`, and `sklearn.base.OutlierMixin`.
warnings.warn(
/opt/hostedtoolcache/Python/3.13.0/x64/lib/python3.13/site-packages/sklearn/utils/_tags.py:322: FutureWarning: Estimator <mne.decoding.transformer.Vectorizer object at 0x7f72d3c7bad0> has no __sklearn_tags__ attribute, which is defined in `sklearn.base.BaseEstimator`. This will raise an error in scikit-learn 1.8. Please define the __sklearn_tags__ method, or inherit from `sklearn.base.BaseEstimator` and other appropriate mixins such as `sklearn.base.TransformerMixin`, `sklearn.base.ClassifierMixin`, `sklearn.base.RegressorMixin`, and `sklearn.base.ClusterMixin`, and `sklearn.base.OutlierMixin`.
warnings.warn(
/opt/hostedtoolcache/Python/3.13.0/x64/lib/python3.13/site-packages/sklearn/utils/_tags.py:322: FutureWarning: Estimator <mne.decoding.transformer.Vectorizer object at 0x7f72d3c7bad0> has no __sklearn_tags__ attribute, which is defined in `sklearn.base.BaseEstimator`. This will raise an error in scikit-learn 1.8. Please define the __sklearn_tags__ method, or inherit from `sklearn.base.BaseEstimator` and other appropriate mixins such as `sklearn.base.TransformerMixin`, `sklearn.base.ClassifierMixin`, `sklearn.base.RegressorMixin`, and `sklearn.base.ClusterMixin`, and `sklearn.base.OutlierMixin`.
warnings.warn(
/opt/hostedtoolcache/Python/3.13.0/x64/lib/python3.13/site-packages/sklearn/utils/_tags.py:322: FutureWarning: Estimator <mne.decoding.transformer.Vectorizer object at 0x7f72d3a1a390> has no __sklearn_tags__ attribute, which is defined in `sklearn.base.BaseEstimator`. This will raise an error in scikit-learn 1.8. Please define the __sklearn_tags__ method, or inherit from `sklearn.base.BaseEstimator` and other appropriate mixins such as `sklearn.base.TransformerMixin`, `sklearn.base.ClassifierMixin`, `sklearn.base.RegressorMixin`, and `sklearn.base.ClusterMixin`, and `sklearn.base.OutlierMixin`.
warnings.warn(
/opt/hostedtoolcache/Python/3.13.0/x64/lib/python3.13/site-packages/sklearn/utils/_tags.py:322: FutureWarning: Estimator <mne.decoding.transformer.Vectorizer object at 0x7f72d3a1a390> has no __sklearn_tags__ attribute, which is defined in `sklearn.base.BaseEstimator`. This will raise an error in scikit-learn 1.8. Please define the __sklearn_tags__ method, or inherit from `sklearn.base.BaseEstimator` and other appropriate mixins such as `sklearn.base.TransformerMixin`, `sklearn.base.ClassifierMixin`, `sklearn.base.RegressorMixin`, and `sklearn.base.ClusterMixin`, and `sklearn.base.OutlierMixin`.
warnings.warn(
/opt/hostedtoolcache/Python/3.13.0/x64/lib/python3.13/site-packages/sklearn/utils/_tags.py:322: FutureWarning: Estimator <mne.decoding.transformer.Vectorizer object at 0x7f72d23bf8c0> has no __sklearn_tags__ attribute, which is defined in `sklearn.base.BaseEstimator`. This will raise an error in scikit-learn 1.8. Please define the __sklearn_tags__ method, or inherit from `sklearn.base.BaseEstimator` and other appropriate mixins such as `sklearn.base.TransformerMixin`, `sklearn.base.ClassifierMixin`, `sklearn.base.RegressorMixin`, and `sklearn.base.ClusterMixin`, and `sklearn.base.OutlierMixin`.
warnings.warn(
/opt/hostedtoolcache/Python/3.13.0/x64/lib/python3.13/site-packages/sklearn/utils/_tags.py:322: FutureWarning: Estimator <mne.decoding.transformer.Vectorizer object at 0x7f72d3a1a2d0> has no __sklearn_tags__ attribute, which is defined in `sklearn.base.BaseEstimator`. This will raise an error in scikit-learn 1.8. Please define the __sklearn_tags__ method, or inherit from `sklearn.base.BaseEstimator` and other appropriate mixins such as `sklearn.base.TransformerMixin`, `sklearn.base.ClassifierMixin`, `sklearn.base.RegressorMixin`, and `sklearn.base.ClusterMixin`, and `sklearn.base.OutlierMixin`.
warnings.warn(
/opt/hostedtoolcache/Python/3.13.0/x64/lib/python3.13/site-packages/sklearn/utils/_tags.py:322: FutureWarning: Estimator <mne.decoding.transformer.Vectorizer object at 0x7f72d3a1a2d0> has no __sklearn_tags__ attribute, which is defined in `sklearn.base.BaseEstimator`. This will raise an error in scikit-learn 1.8. Please define the __sklearn_tags__ method, or inherit from `sklearn.base.BaseEstimator` and other appropriate mixins such as `sklearn.base.TransformerMixin`, `sklearn.base.ClassifierMixin`, `sklearn.base.RegressorMixin`, and `sklearn.base.ClusterMixin`, and `sklearn.base.OutlierMixin`.
warnings.warn(
/opt/hostedtoolcache/Python/3.13.0/x64/lib/python3.13/site-packages/sklearn/utils/_tags.py:322: FutureWarning: Estimator <mne.decoding.transformer.Vectorizer object at 0x7f72d3daa200> has no __sklearn_tags__ attribute, which is defined in `sklearn.base.BaseEstimator`. This will raise an error in scikit-learn 1.8. Please define the __sklearn_tags__ method, or inherit from `sklearn.base.BaseEstimator` and other appropriate mixins such as `sklearn.base.TransformerMixin`, `sklearn.base.ClassifierMixin`, `sklearn.base.RegressorMixin`, and `sklearn.base.ClusterMixin`, and `sklearn.base.OutlierMixin`.
warnings.warn(
/opt/hostedtoolcache/Python/3.13.0/x64/lib/python3.13/site-packages/sklearn/utils/_tags.py:322: FutureWarning: Estimator <mne.decoding.transformer.Vectorizer object at 0x7f72d3daa200> has no __sklearn_tags__ attribute, which is defined in `sklearn.base.BaseEstimator`. This will raise an error in scikit-learn 1.8. Please define the __sklearn_tags__ method, or inherit from `sklearn.base.BaseEstimator` and other appropriate mixins such as `sklearn.base.TransformerMixin`, `sklearn.base.ClassifierMixin`, `sklearn.base.RegressorMixin`, and `sklearn.base.ClusterMixin`, and `sklearn.base.OutlierMixin`.
warnings.warn(
/opt/hostedtoolcache/Python/3.13.0/x64/lib/python3.13/site-packages/sklearn/utils/_tags.py:322: FutureWarning: Estimator <mne.decoding.transformer.Vectorizer object at 0x7f72d3da9d30> has no __sklearn_tags__ attribute, which is defined in `sklearn.base.BaseEstimator`. This will raise an error in scikit-learn 1.8. Please define the __sklearn_tags__ method, or inherit from `sklearn.base.BaseEstimator` and other appropriate mixins such as `sklearn.base.TransformerMixin`, `sklearn.base.ClassifierMixin`, `sklearn.base.RegressorMixin`, and `sklearn.base.ClusterMixin`, and `sklearn.base.OutlierMixin`.
warnings.warn(
/opt/hostedtoolcache/Python/3.13.0/x64/lib/python3.13/site-packages/sklearn/utils/_tags.py:322: FutureWarning: Estimator <mne.decoding.transformer.Vectorizer object at 0x7f72d3da9d30> has no __sklearn_tags__ attribute, which is defined in `sklearn.base.BaseEstimator`. This will raise an error in scikit-learn 1.8. Please define the __sklearn_tags__ method, or inherit from `sklearn.base.BaseEstimator` and other appropriate mixins such as `sklearn.base.TransformerMixin`, `sklearn.base.ClassifierMixin`, `sklearn.base.RegressorMixin`, and `sklearn.base.ClusterMixin`, and `sklearn.base.OutlierMixin`.
warnings.warn(
/opt/hostedtoolcache/Python/3.13.0/x64/lib/python3.13/site-packages/sklearn/utils/_tags.py:322: FutureWarning: Estimator <mne.decoding.transformer.Vectorizer object at 0x7f72d2775db0> has no __sklearn_tags__ attribute, which is defined in `sklearn.base.BaseEstimator`. This will raise an error in scikit-learn 1.8. Please define the __sklearn_tags__ method, or inherit from `sklearn.base.BaseEstimator` and other appropriate mixins such as `sklearn.base.TransformerMixin`, `sklearn.base.ClassifierMixin`, `sklearn.base.RegressorMixin`, and `sklearn.base.ClusterMixin`, and `sklearn.base.OutlierMixin`.
warnings.warn(
/opt/hostedtoolcache/Python/3.13.0/x64/lib/python3.13/site-packages/sklearn/utils/_tags.py:322: FutureWarning: Estimator <mne.decoding.transformer.Vectorizer object at 0x7f72d2775db0> has no __sklearn_tags__ attribute, which is defined in `sklearn.base.BaseEstimator`. This will raise an error in scikit-learn 1.8. Please define the __sklearn_tags__ method, or inherit from `sklearn.base.BaseEstimator` and other appropriate mixins such as `sklearn.base.TransformerMixin`, `sklearn.base.ClassifierMixin`, `sklearn.base.RegressorMixin`, and `sklearn.base.ClusterMixin`, and `sklearn.base.OutlierMixin`.
warnings.warn(
/opt/hostedtoolcache/Python/3.13.0/x64/lib/python3.13/site-packages/sklearn/utils/_tags.py:322: FutureWarning: Estimator <mne.decoding.transformer.Vectorizer object at 0x7f72d2777070> has no __sklearn_tags__ attribute, which is defined in `sklearn.base.BaseEstimator`. This will raise an error in scikit-learn 1.8. Please define the __sklearn_tags__ method, or inherit from `sklearn.base.BaseEstimator` and other appropriate mixins such as `sklearn.base.TransformerMixin`, `sklearn.base.ClassifierMixin`, `sklearn.base.RegressorMixin`, and `sklearn.base.ClusterMixin`, and `sklearn.base.OutlierMixin`.
warnings.warn(
/opt/hostedtoolcache/Python/3.13.0/x64/lib/python3.13/site-packages/sklearn/utils/_tags.py:322: FutureWarning: Estimator <mne.decoding.transformer.Vectorizer object at 0x7f72d2777070> has no __sklearn_tags__ attribute, which is defined in `sklearn.base.BaseEstimator`. This will raise an error in scikit-learn 1.8. Please define the __sklearn_tags__ method, or inherit from `sklearn.base.BaseEstimator` and other appropriate mixins such as `sklearn.base.TransformerMixin`, `sklearn.base.ClassifierMixin`, `sklearn.base.RegressorMixin`, and `sklearn.base.ClusterMixin`, and `sklearn.base.OutlierMixin`.
warnings.warn(
/opt/hostedtoolcache/Python/3.13.0/x64/lib/python3.13/site-packages/sklearn/utils/_tags.py:322: FutureWarning: Estimator <mne.decoding.transformer.Vectorizer object at 0x7f72d23bf8c0> has no __sklearn_tags__ attribute, which is defined in `sklearn.base.BaseEstimator`. This will raise an error in scikit-learn 1.8. Please define the __sklearn_tags__ method, or inherit from `sklearn.base.BaseEstimator` and other appropriate mixins such as `sklearn.base.TransformerMixin`, `sklearn.base.ClassifierMixin`, `sklearn.base.RegressorMixin`, and `sklearn.base.ClusterMixin`, and `sklearn.base.OutlierMixin`.
warnings.warn(
/opt/hostedtoolcache/Python/3.13.0/x64/lib/python3.13/site-packages/sklearn/utils/_tags.py:322: FutureWarning: Estimator <mne.decoding.transformer.Vectorizer object at 0x7f72d3dd9520> has no __sklearn_tags__ attribute, which is defined in `sklearn.base.BaseEstimator`. This will raise an error in scikit-learn 1.8. Please define the __sklearn_tags__ method, or inherit from `sklearn.base.BaseEstimator` and other appropriate mixins such as `sklearn.base.TransformerMixin`, `sklearn.base.ClassifierMixin`, `sklearn.base.RegressorMixin`, and `sklearn.base.ClusterMixin`, and `sklearn.base.OutlierMixin`.
warnings.warn(
/opt/hostedtoolcache/Python/3.13.0/x64/lib/python3.13/site-packages/sklearn/utils/_tags.py:322: FutureWarning: Estimator <mne.decoding.transformer.Vectorizer object at 0x7f72d3dd9520> has no __sklearn_tags__ attribute, which is defined in `sklearn.base.BaseEstimator`. This will raise an error in scikit-learn 1.8. Please define the __sklearn_tags__ method, or inherit from `sklearn.base.BaseEstimator` and other appropriate mixins such as `sklearn.base.TransformerMixin`, `sklearn.base.ClassifierMixin`, `sklearn.base.RegressorMixin`, and `sklearn.base.ClusterMixin`, and `sklearn.base.OutlierMixin`.
warnings.warn(
/opt/hostedtoolcache/Python/3.13.0/x64/lib/python3.13/site-packages/sklearn/utils/_tags.py:322: FutureWarning: Estimator <mne.decoding.transformer.Vectorizer object at 0x7f7274131cd0> has no __sklearn_tags__ attribute, which is defined in `sklearn.base.BaseEstimator`. This will raise an error in scikit-learn 1.8. Please define the __sklearn_tags__ method, or inherit from `sklearn.base.BaseEstimator` and other appropriate mixins such as `sklearn.base.TransformerMixin`, `sklearn.base.ClassifierMixin`, `sklearn.base.RegressorMixin`, and `sklearn.base.ClusterMixin`, and `sklearn.base.OutlierMixin`.
warnings.warn(
/opt/hostedtoolcache/Python/3.13.0/x64/lib/python3.13/site-packages/sklearn/utils/_tags.py:322: FutureWarning: Estimator <mne.decoding.transformer.Vectorizer object at 0x7f7274131cd0> has no __sklearn_tags__ attribute, which is defined in `sklearn.base.BaseEstimator`. This will raise an error in scikit-learn 1.8. Please define the __sklearn_tags__ method, or inherit from `sklearn.base.BaseEstimator` and other appropriate mixins such as `sklearn.base.TransformerMixin`, `sklearn.base.ClassifierMixin`, `sklearn.base.RegressorMixin`, and `sklearn.base.ClusterMixin`, and `sklearn.base.OutlierMixin`.
warnings.warn(
/opt/hostedtoolcache/Python/3.13.0/x64/lib/python3.13/site-packages/sklearn/utils/_tags.py:322: FutureWarning: Estimator <mne.decoding.transformer.Vectorizer object at 0x7f7274131ed0> has no __sklearn_tags__ attribute, which is defined in `sklearn.base.BaseEstimator`. This will raise an error in scikit-learn 1.8. Please define the __sklearn_tags__ method, or inherit from `sklearn.base.BaseEstimator` and other appropriate mixins such as `sklearn.base.TransformerMixin`, `sklearn.base.ClassifierMixin`, `sklearn.base.RegressorMixin`, and `sklearn.base.ClusterMixin`, and `sklearn.base.OutlierMixin`.
warnings.warn(
/opt/hostedtoolcache/Python/3.13.0/x64/lib/python3.13/site-packages/sklearn/utils/_tags.py:322: FutureWarning: Estimator <mne.decoding.transformer.Vectorizer object at 0x7f7274131ed0> has no __sklearn_tags__ attribute, which is defined in `sklearn.base.BaseEstimator`. This will raise an error in scikit-learn 1.8. Please define the __sklearn_tags__ method, or inherit from `sklearn.base.BaseEstimator` and other appropriate mixins such as `sklearn.base.TransformerMixin`, `sklearn.base.ClassifierMixin`, `sklearn.base.RegressorMixin`, and `sklearn.base.ClusterMixin`, and `sklearn.base.OutlierMixin`.
warnings.warn(
/opt/hostedtoolcache/Python/3.13.0/x64/lib/python3.13/site-packages/sklearn/utils/_tags.py:322: FutureWarning: Estimator <mne.decoding.transformer.Vectorizer object at 0x7f72d3135ef0> has no __sklearn_tags__ attribute, which is defined in `sklearn.base.BaseEstimator`. This will raise an error in scikit-learn 1.8. Please define the __sklearn_tags__ method, or inherit from `sklearn.base.BaseEstimator` and other appropriate mixins such as `sklearn.base.TransformerMixin`, `sklearn.base.ClassifierMixin`, `sklearn.base.RegressorMixin`, and `sklearn.base.ClusterMixin`, and `sklearn.base.OutlierMixin`.
warnings.warn(
/opt/hostedtoolcache/Python/3.13.0/x64/lib/python3.13/site-packages/sklearn/utils/_tags.py:322: FutureWarning: Estimator <mne.decoding.transformer.Vectorizer object at 0x7f72d3135ef0> has no __sklearn_tags__ attribute, which is defined in `sklearn.base.BaseEstimator`. This will raise an error in scikit-learn 1.8. Please define the __sklearn_tags__ method, or inherit from `sklearn.base.BaseEstimator` and other appropriate mixins such as `sklearn.base.TransformerMixin`, `sklearn.base.ClassifierMixin`, `sklearn.base.RegressorMixin`, and `sklearn.base.ClusterMixin`, and `sklearn.base.OutlierMixin`.
warnings.warn(
/opt/hostedtoolcache/Python/3.13.0/x64/lib/python3.13/site-packages/sklearn/utils/_tags.py:322: FutureWarning: Estimator <mne.decoding.transformer.Vectorizer object at 0x7f72d3136660> has no __sklearn_tags__ attribute, which is defined in `sklearn.base.BaseEstimator`. This will raise an error in scikit-learn 1.8. Please define the __sklearn_tags__ method, or inherit from `sklearn.base.BaseEstimator` and other appropriate mixins such as `sklearn.base.TransformerMixin`, `sklearn.base.ClassifierMixin`, `sklearn.base.RegressorMixin`, and `sklearn.base.ClusterMixin`, and `sklearn.base.OutlierMixin`.
warnings.warn(
/opt/hostedtoolcache/Python/3.13.0/x64/lib/python3.13/site-packages/sklearn/utils/_tags.py:322: FutureWarning: Estimator <mne.decoding.transformer.Vectorizer object at 0x7f72d3136660> has no __sklearn_tags__ attribute, which is defined in `sklearn.base.BaseEstimator`. This will raise an error in scikit-learn 1.8. Please define the __sklearn_tags__ method, or inherit from `sklearn.base.BaseEstimator` and other appropriate mixins such as `sklearn.base.TransformerMixin`, `sklearn.base.ClassifierMixin`, `sklearn.base.RegressorMixin`, and `sklearn.base.ClusterMixin`, and `sklearn.base.OutlierMixin`.
warnings.warn(
/opt/hostedtoolcache/Python/3.13.0/x64/lib/python3.13/site-packages/sklearn/utils/_tags.py:322: FutureWarning: Estimator <mne.decoding.transformer.Vectorizer object at 0x7f72d3136660> has no __sklearn_tags__ attribute, which is defined in `sklearn.base.BaseEstimator`. This will raise an error in scikit-learn 1.8. Please define the __sklearn_tags__ method, or inherit from `sklearn.base.BaseEstimator` and other appropriate mixins such as `sklearn.base.TransformerMixin`, `sklearn.base.ClassifierMixin`, `sklearn.base.RegressorMixin`, and `sklearn.base.ClusterMixin`, and `sklearn.base.OutlierMixin`.
warnings.warn(
/opt/hostedtoolcache/Python/3.13.0/x64/lib/python3.13/site-packages/sklearn/utils/_tags.py:322: FutureWarning: Estimator <mne.decoding.transformer.Vectorizer object at 0x7f72d23bf8c0> has no __sklearn_tags__ attribute, which is defined in `sklearn.base.BaseEstimator`. This will raise an error in scikit-learn 1.8. Please define the __sklearn_tags__ method, or inherit from `sklearn.base.BaseEstimator` and other appropriate mixins such as `sklearn.base.TransformerMixin`, `sklearn.base.ClassifierMixin`, `sklearn.base.RegressorMixin`, and `sklearn.base.ClusterMixin`, and `sklearn.base.OutlierMixin`.
warnings.warn(
/opt/hostedtoolcache/Python/3.13.0/x64/lib/python3.13/site-packages/sklearn/utils/_tags.py:322: FutureWarning: Estimator <mne.decoding.transformer.Vectorizer object at 0x7f72d2debd10> has no __sklearn_tags__ attribute, which is defined in `sklearn.base.BaseEstimator`. This will raise an error in scikit-learn 1.8. Please define the __sklearn_tags__ method, or inherit from `sklearn.base.BaseEstimator` and other appropriate mixins such as `sklearn.base.TransformerMixin`, `sklearn.base.ClassifierMixin`, `sklearn.base.RegressorMixin`, and `sklearn.base.ClusterMixin`, and `sklearn.base.OutlierMixin`.
warnings.warn(
/opt/hostedtoolcache/Python/3.13.0/x64/lib/python3.13/site-packages/sklearn/utils/_tags.py:322: FutureWarning: Estimator <mne.decoding.transformer.Vectorizer object at 0x7f72d2debd10> has no __sklearn_tags__ attribute, which is defined in `sklearn.base.BaseEstimator`. This will raise an error in scikit-learn 1.8. Please define the __sklearn_tags__ method, or inherit from `sklearn.base.BaseEstimator` and other appropriate mixins such as `sklearn.base.TransformerMixin`, `sklearn.base.ClassifierMixin`, `sklearn.base.RegressorMixin`, and `sklearn.base.ClusterMixin`, and `sklearn.base.OutlierMixin`.
warnings.warn(
/opt/hostedtoolcache/Python/3.13.0/x64/lib/python3.13/site-packages/sklearn/utils/_tags.py:322: FutureWarning: Estimator <mne.decoding.transformer.Vectorizer object at 0x7f72d2debd10> has no __sklearn_tags__ attribute, which is defined in `sklearn.base.BaseEstimator`. This will raise an error in scikit-learn 1.8. Please define the __sklearn_tags__ method, or inherit from `sklearn.base.BaseEstimator` and other appropriate mixins such as `sklearn.base.TransformerMixin`, `sklearn.base.ClassifierMixin`, `sklearn.base.RegressorMixin`, and `sklearn.base.ClusterMixin`, and `sklearn.base.OutlierMixin`.
warnings.warn(
/opt/hostedtoolcache/Python/3.13.0/x64/lib/python3.13/site-packages/sklearn/utils/_tags.py:322: FutureWarning: Estimator <mne.decoding.transformer.Vectorizer object at 0x7f72d2deb4d0> has no __sklearn_tags__ attribute, which is defined in `sklearn.base.BaseEstimator`. This will raise an error in scikit-learn 1.8. Please define the __sklearn_tags__ method, or inherit from `sklearn.base.BaseEstimator` and other appropriate mixins such as `sklearn.base.TransformerMixin`, `sklearn.base.ClassifierMixin`, `sklearn.base.RegressorMixin`, and `sklearn.base.ClusterMixin`, and `sklearn.base.OutlierMixin`.
warnings.warn(
/opt/hostedtoolcache/Python/3.13.0/x64/lib/python3.13/site-packages/sklearn/utils/_tags.py:322: FutureWarning: Estimator <mne.decoding.transformer.Vectorizer object at 0x7f72d2deb4d0> has no __sklearn_tags__ attribute, which is defined in `sklearn.base.BaseEstimator`. This will raise an error in scikit-learn 1.8. Please define the __sklearn_tags__ method, or inherit from `sklearn.base.BaseEstimator` and other appropriate mixins such as `sklearn.base.TransformerMixin`, `sklearn.base.ClassifierMixin`, `sklearn.base.RegressorMixin`, and `sklearn.base.ClusterMixin`, and `sklearn.base.OutlierMixin`.
warnings.warn(
/opt/hostedtoolcache/Python/3.13.0/x64/lib/python3.13/site-packages/sklearn/utils/_tags.py:322: FutureWarning: Estimator <mne.decoding.transformer.Vectorizer object at 0x7f72bc816490> has no __sklearn_tags__ attribute, which is defined in `sklearn.base.BaseEstimator`. This will raise an error in scikit-learn 1.8. Please define the __sklearn_tags__ method, or inherit from `sklearn.base.BaseEstimator` and other appropriate mixins such as `sklearn.base.TransformerMixin`, `sklearn.base.ClassifierMixin`, `sklearn.base.RegressorMixin`, and `sklearn.base.ClusterMixin`, and `sklearn.base.OutlierMixin`.
warnings.warn(
/opt/hostedtoolcache/Python/3.13.0/x64/lib/python3.13/site-packages/sklearn/utils/_tags.py:322: FutureWarning: Estimator <mne.decoding.transformer.Vectorizer object at 0x7f72bc816490> has no __sklearn_tags__ attribute, which is defined in `sklearn.base.BaseEstimator`. This will raise an error in scikit-learn 1.8. Please define the __sklearn_tags__ method, or inherit from `sklearn.base.BaseEstimator` and other appropriate mixins such as `sklearn.base.TransformerMixin`, `sklearn.base.ClassifierMixin`, `sklearn.base.RegressorMixin`, and `sklearn.base.ClusterMixin`, and `sklearn.base.OutlierMixin`.
warnings.warn(
/opt/hostedtoolcache/Python/3.13.0/x64/lib/python3.13/site-packages/sklearn/utils/_tags.py:322: FutureWarning: Estimator <mne.decoding.transformer.Vectorizer object at 0x7f72bc8155e0> has no __sklearn_tags__ attribute, which is defined in `sklearn.base.BaseEstimator`. This will raise an error in scikit-learn 1.8. Please define the __sklearn_tags__ method, or inherit from `sklearn.base.BaseEstimator` and other appropriate mixins such as `sklearn.base.TransformerMixin`, `sklearn.base.ClassifierMixin`, `sklearn.base.RegressorMixin`, and `sklearn.base.ClusterMixin`, and `sklearn.base.OutlierMixin`.
warnings.warn(
/opt/hostedtoolcache/Python/3.13.0/x64/lib/python3.13/site-packages/sklearn/utils/_tags.py:322: FutureWarning: Estimator <mne.decoding.transformer.Vectorizer object at 0x7f72bc8155e0> has no __sklearn_tags__ attribute, which is defined in `sklearn.base.BaseEstimator`. This will raise an error in scikit-learn 1.8. Please define the __sklearn_tags__ method, or inherit from `sklearn.base.BaseEstimator` and other appropriate mixins such as `sklearn.base.TransformerMixin`, `sklearn.base.ClassifierMixin`, `sklearn.base.RegressorMixin`, and `sklearn.base.ClusterMixin`, and `sklearn.base.OutlierMixin`.
warnings.warn(
/opt/hostedtoolcache/Python/3.13.0/x64/lib/python3.13/site-packages/sklearn/utils/_tags.py:322: FutureWarning: Estimator <mne.decoding.transformer.Vectorizer object at 0x7f72bc1dd6d0> has no __sklearn_tags__ attribute, which is defined in `sklearn.base.BaseEstimator`. This will raise an error in scikit-learn 1.8. Please define the __sklearn_tags__ method, or inherit from `sklearn.base.BaseEstimator` and other appropriate mixins such as `sklearn.base.TransformerMixin`, `sklearn.base.ClassifierMixin`, `sklearn.base.RegressorMixin`, and `sklearn.base.ClusterMixin`, and `sklearn.base.OutlierMixin`.
warnings.warn(
/opt/hostedtoolcache/Python/3.13.0/x64/lib/python3.13/site-packages/sklearn/utils/_tags.py:322: FutureWarning: Estimator <mne.decoding.transformer.Vectorizer object at 0x7f72bc1dd6d0> has no __sklearn_tags__ attribute, which is defined in `sklearn.base.BaseEstimator`. This will raise an error in scikit-learn 1.8. Please define the __sklearn_tags__ method, or inherit from `sklearn.base.BaseEstimator` and other appropriate mixins such as `sklearn.base.TransformerMixin`, `sklearn.base.ClassifierMixin`, `sklearn.base.RegressorMixin`, and `sklearn.base.ClusterMixin`, and `sklearn.base.OutlierMixin`.
warnings.warn(
/opt/hostedtoolcache/Python/3.13.0/x64/lib/python3.13/site-packages/sklearn/utils/_tags.py:322: FutureWarning: Estimator <mne.decoding.transformer.Vectorizer object at 0x7f72d23bf8c0> has no __sklearn_tags__ attribute, which is defined in `sklearn.base.BaseEstimator`. This will raise an error in scikit-learn 1.8. Please define the __sklearn_tags__ method, or inherit from `sklearn.base.BaseEstimator` and other appropriate mixins such as `sklearn.base.TransformerMixin`, `sklearn.base.ClassifierMixin`, `sklearn.base.RegressorMixin`, and `sklearn.base.ClusterMixin`, and `sklearn.base.OutlierMixin`.
warnings.warn(
/opt/hostedtoolcache/Python/3.13.0/x64/lib/python3.13/site-packages/sklearn/utils/_tags.py:322: FutureWarning: Estimator <mne.decoding.transformer.Vectorizer object at 0x7f72d22f47d0> has no __sklearn_tags__ attribute, which is defined in `sklearn.base.BaseEstimator`. This will raise an error in scikit-learn 1.8. Please define the __sklearn_tags__ method, or inherit from `sklearn.base.BaseEstimator` and other appropriate mixins such as `sklearn.base.TransformerMixin`, `sklearn.base.ClassifierMixin`, `sklearn.base.RegressorMixin`, and `sklearn.base.ClusterMixin`, and `sklearn.base.OutlierMixin`.
warnings.warn(
/opt/hostedtoolcache/Python/3.13.0/x64/lib/python3.13/site-packages/sklearn/utils/_tags.py:322: FutureWarning: Estimator <mne.decoding.transformer.Vectorizer object at 0x7f72d22f47d0> has no __sklearn_tags__ attribute, which is defined in `sklearn.base.BaseEstimator`. This will raise an error in scikit-learn 1.8. Please define the __sklearn_tags__ method, or inherit from `sklearn.base.BaseEstimator` and other appropriate mixins such as `sklearn.base.TransformerMixin`, `sklearn.base.ClassifierMixin`, `sklearn.base.RegressorMixin`, and `sklearn.base.ClusterMixin`, and `sklearn.base.OutlierMixin`.
warnings.warn(
/opt/hostedtoolcache/Python/3.13.0/x64/lib/python3.13/site-packages/sklearn/utils/_tags.py:322: FutureWarning: Estimator <mne.decoding.transformer.Vectorizer object at 0x7f72d22f6da0> has no __sklearn_tags__ attribute, which is defined in `sklearn.base.BaseEstimator`. This will raise an error in scikit-learn 1.8. Please define the __sklearn_tags__ method, or inherit from `sklearn.base.BaseEstimator` and other appropriate mixins such as `sklearn.base.TransformerMixin`, `sklearn.base.ClassifierMixin`, `sklearn.base.RegressorMixin`, and `sklearn.base.ClusterMixin`, and `sklearn.base.OutlierMixin`.
warnings.warn(
/opt/hostedtoolcache/Python/3.13.0/x64/lib/python3.13/site-packages/sklearn/utils/_tags.py:322: FutureWarning: Estimator <mne.decoding.transformer.Vectorizer object at 0x7f72d22f6da0> has no __sklearn_tags__ attribute, which is defined in `sklearn.base.BaseEstimator`. This will raise an error in scikit-learn 1.8. Please define the __sklearn_tags__ method, or inherit from `sklearn.base.BaseEstimator` and other appropriate mixins such as `sklearn.base.TransformerMixin`, `sklearn.base.ClassifierMixin`, `sklearn.base.RegressorMixin`, and `sklearn.base.ClusterMixin`, and `sklearn.base.OutlierMixin`.
warnings.warn(
/opt/hostedtoolcache/Python/3.13.0/x64/lib/python3.13/site-packages/sklearn/utils/_tags.py:322: FutureWarning: Estimator <mne.decoding.transformer.Vectorizer object at 0x7f72d22f73e0> has no __sklearn_tags__ attribute, which is defined in `sklearn.base.BaseEstimator`. This will raise an error in scikit-learn 1.8. Please define the __sklearn_tags__ method, or inherit from `sklearn.base.BaseEstimator` and other appropriate mixins such as `sklearn.base.TransformerMixin`, `sklearn.base.ClassifierMixin`, `sklearn.base.RegressorMixin`, and `sklearn.base.ClusterMixin`, and `sklearn.base.OutlierMixin`.
warnings.warn(
/opt/hostedtoolcache/Python/3.13.0/x64/lib/python3.13/site-packages/sklearn/utils/_tags.py:322: FutureWarning: Estimator <mne.decoding.transformer.Vectorizer object at 0x7f72d22f73e0> has no __sklearn_tags__ attribute, which is defined in `sklearn.base.BaseEstimator`. This will raise an error in scikit-learn 1.8. Please define the __sklearn_tags__ method, or inherit from `sklearn.base.BaseEstimator` and other appropriate mixins such as `sklearn.base.TransformerMixin`, `sklearn.base.ClassifierMixin`, `sklearn.base.RegressorMixin`, and `sklearn.base.ClusterMixin`, and `sklearn.base.OutlierMixin`.
warnings.warn(
/opt/hostedtoolcache/Python/3.13.0/x64/lib/python3.13/site-packages/sklearn/utils/_tags.py:322: FutureWarning: Estimator <mne.decoding.transformer.Vectorizer object at 0x7f72d22f4230> has no __sklearn_tags__ attribute, which is defined in `sklearn.base.BaseEstimator`. This will raise an error in scikit-learn 1.8. Please define the __sklearn_tags__ method, or inherit from `sklearn.base.BaseEstimator` and other appropriate mixins such as `sklearn.base.TransformerMixin`, `sklearn.base.ClassifierMixin`, `sklearn.base.RegressorMixin`, and `sklearn.base.ClusterMixin`, and `sklearn.base.OutlierMixin`.
warnings.warn(
/opt/hostedtoolcache/Python/3.13.0/x64/lib/python3.13/site-packages/sklearn/utils/_tags.py:322: FutureWarning: Estimator <mne.decoding.transformer.Vectorizer object at 0x7f72d22f4230> has no __sklearn_tags__ attribute, which is defined in `sklearn.base.BaseEstimator`. This will raise an error in scikit-learn 1.8. Please define the __sklearn_tags__ method, or inherit from `sklearn.base.BaseEstimator` and other appropriate mixins such as `sklearn.base.TransformerMixin`, `sklearn.base.ClassifierMixin`, `sklearn.base.RegressorMixin`, and `sklearn.base.ClusterMixin`, and `sklearn.base.OutlierMixin`.
warnings.warn(
/opt/hostedtoolcache/Python/3.13.0/x64/lib/python3.13/site-packages/sklearn/utils/_tags.py:322: FutureWarning: Estimator <mne.decoding.transformer.Vectorizer object at 0x7f72d22f63f0> has no __sklearn_tags__ attribute, which is defined in `sklearn.base.BaseEstimator`. This will raise an error in scikit-learn 1.8. Please define the __sklearn_tags__ method, or inherit from `sklearn.base.BaseEstimator` and other appropriate mixins such as `sklearn.base.TransformerMixin`, `sklearn.base.ClassifierMixin`, `sklearn.base.RegressorMixin`, and `sklearn.base.ClusterMixin`, and `sklearn.base.OutlierMixin`.
warnings.warn(
/opt/hostedtoolcache/Python/3.13.0/x64/lib/python3.13/site-packages/sklearn/utils/_tags.py:322: FutureWarning: Estimator <mne.decoding.transformer.Vectorizer object at 0x7f72d22f63f0> has no __sklearn_tags__ attribute, which is defined in `sklearn.base.BaseEstimator`. This will raise an error in scikit-learn 1.8. Please define the __sklearn_tags__ method, or inherit from `sklearn.base.BaseEstimator` and other appropriate mixins such as `sklearn.base.TransformerMixin`, `sklearn.base.ClassifierMixin`, `sklearn.base.RegressorMixin`, and `sklearn.base.ClusterMixin`, and `sklearn.base.OutlierMixin`.
warnings.warn(
/opt/hostedtoolcache/Python/3.13.0/x64/lib/python3.13/site-packages/sklearn/utils/_tags.py:322: FutureWarning: Estimator <mne.decoding.transformer.Vectorizer object at 0x7f72d23bf8c0> has no __sklearn_tags__ attribute, which is defined in `sklearn.base.BaseEstimator`. This will raise an error in scikit-learn 1.8. Please define the __sklearn_tags__ method, or inherit from `sklearn.base.BaseEstimator` and other appropriate mixins such as `sklearn.base.TransformerMixin`, `sklearn.base.ClassifierMixin`, `sklearn.base.RegressorMixin`, and `sklearn.base.ClusterMixin`, and `sklearn.base.OutlierMixin`.
warnings.warn(
/opt/hostedtoolcache/Python/3.13.0/x64/lib/python3.13/site-packages/sklearn/utils/_tags.py:322: FutureWarning: Estimator <mne.decoding.transformer.Vectorizer object at 0x7f72d22f7250> has no __sklearn_tags__ attribute, which is defined in `sklearn.base.BaseEstimator`. This will raise an error in scikit-learn 1.8. Please define the __sklearn_tags__ method, or inherit from `sklearn.base.BaseEstimator` and other appropriate mixins such as `sklearn.base.TransformerMixin`, `sklearn.base.ClassifierMixin`, `sklearn.base.RegressorMixin`, and `sklearn.base.ClusterMixin`, and `sklearn.base.OutlierMixin`.
warnings.warn(
/opt/hostedtoolcache/Python/3.13.0/x64/lib/python3.13/site-packages/sklearn/utils/_tags.py:322: FutureWarning: Estimator <mne.decoding.transformer.Vectorizer object at 0x7f72d22f7250> has no __sklearn_tags__ attribute, which is defined in `sklearn.base.BaseEstimator`. This will raise an error in scikit-learn 1.8. Please define the __sklearn_tags__ method, or inherit from `sklearn.base.BaseEstimator` and other appropriate mixins such as `sklearn.base.TransformerMixin`, `sklearn.base.ClassifierMixin`, `sklearn.base.RegressorMixin`, and `sklearn.base.ClusterMixin`, and `sklearn.base.OutlierMixin`.
warnings.warn(
/opt/hostedtoolcache/Python/3.13.0/x64/lib/python3.13/site-packages/sklearn/utils/_tags.py:322: FutureWarning: Estimator <mne.decoding.transformer.Vectorizer object at 0x7f72d22f54f0> has no __sklearn_tags__ attribute, which is defined in `sklearn.base.BaseEstimator`. This will raise an error in scikit-learn 1.8. Please define the __sklearn_tags__ method, or inherit from `sklearn.base.BaseEstimator` and other appropriate mixins such as `sklearn.base.TransformerMixin`, `sklearn.base.ClassifierMixin`, `sklearn.base.RegressorMixin`, and `sklearn.base.ClusterMixin`, and `sklearn.base.OutlierMixin`.
warnings.warn(
/opt/hostedtoolcache/Python/3.13.0/x64/lib/python3.13/site-packages/sklearn/utils/_tags.py:322: FutureWarning: Estimator <mne.decoding.transformer.Vectorizer object at 0x7f72d22f54f0> has no __sklearn_tags__ attribute, which is defined in `sklearn.base.BaseEstimator`. This will raise an error in scikit-learn 1.8. Please define the __sklearn_tags__ method, or inherit from `sklearn.base.BaseEstimator` and other appropriate mixins such as `sklearn.base.TransformerMixin`, `sklearn.base.ClassifierMixin`, `sklearn.base.RegressorMixin`, and `sklearn.base.ClusterMixin`, and `sklearn.base.OutlierMixin`.
warnings.warn(
/opt/hostedtoolcache/Python/3.13.0/x64/lib/python3.13/site-packages/sklearn/utils/_tags.py:322: FutureWarning: Estimator <mne.decoding.transformer.Vectorizer object at 0x7f72d22f5130> has no __sklearn_tags__ attribute, which is defined in `sklearn.base.BaseEstimator`. This will raise an error in scikit-learn 1.8. Please define the __sklearn_tags__ method, or inherit from `sklearn.base.BaseEstimator` and other appropriate mixins such as `sklearn.base.TransformerMixin`, `sklearn.base.ClassifierMixin`, `sklearn.base.RegressorMixin`, and `sklearn.base.ClusterMixin`, and `sklearn.base.OutlierMixin`.
warnings.warn(
/opt/hostedtoolcache/Python/3.13.0/x64/lib/python3.13/site-packages/sklearn/utils/_tags.py:322: FutureWarning: Estimator <mne.decoding.transformer.Vectorizer object at 0x7f72d22f5130> has no __sklearn_tags__ attribute, which is defined in `sklearn.base.BaseEstimator`. This will raise an error in scikit-learn 1.8. Please define the __sklearn_tags__ method, or inherit from `sklearn.base.BaseEstimator` and other appropriate mixins such as `sklearn.base.TransformerMixin`, `sklearn.base.ClassifierMixin`, `sklearn.base.RegressorMixin`, and `sklearn.base.ClusterMixin`, and `sklearn.base.OutlierMixin`.
warnings.warn(
/opt/hostedtoolcache/Python/3.13.0/x64/lib/python3.13/site-packages/sklearn/utils/_tags.py:322: FutureWarning: Estimator <mne.decoding.transformer.Vectorizer object at 0x7f72d22f7a20> has no __sklearn_tags__ attribute, which is defined in `sklearn.base.BaseEstimator`. This will raise an error in scikit-learn 1.8. Please define the __sklearn_tags__ method, or inherit from `sklearn.base.BaseEstimator` and other appropriate mixins such as `sklearn.base.TransformerMixin`, `sklearn.base.ClassifierMixin`, `sklearn.base.RegressorMixin`, and `sklearn.base.ClusterMixin`, and `sklearn.base.OutlierMixin`.
warnings.warn(
/opt/hostedtoolcache/Python/3.13.0/x64/lib/python3.13/site-packages/sklearn/utils/_tags.py:322: FutureWarning: Estimator <mne.decoding.transformer.Vectorizer object at 0x7f72d22f7a20> has no __sklearn_tags__ attribute, which is defined in `sklearn.base.BaseEstimator`. This will raise an error in scikit-learn 1.8. Please define the __sklearn_tags__ method, or inherit from `sklearn.base.BaseEstimator` and other appropriate mixins such as `sklearn.base.TransformerMixin`, `sklearn.base.ClassifierMixin`, `sklearn.base.RegressorMixin`, and `sklearn.base.ClusterMixin`, and `sklearn.base.OutlierMixin`.
warnings.warn(
/opt/hostedtoolcache/Python/3.13.0/x64/lib/python3.13/site-packages/sklearn/utils/_tags.py:322: FutureWarning: Estimator <mne.decoding.transformer.Vectorizer object at 0x7f72d22f5cc0> has no __sklearn_tags__ attribute, which is defined in `sklearn.base.BaseEstimator`. This will raise an error in scikit-learn 1.8. Please define the __sklearn_tags__ method, or inherit from `sklearn.base.BaseEstimator` and other appropriate mixins such as `sklearn.base.TransformerMixin`, `sklearn.base.ClassifierMixin`, `sklearn.base.RegressorMixin`, and `sklearn.base.ClusterMixin`, and `sklearn.base.OutlierMixin`.
warnings.warn(
/opt/hostedtoolcache/Python/3.13.0/x64/lib/python3.13/site-packages/sklearn/utils/_tags.py:322: FutureWarning: Estimator <mne.decoding.transformer.Vectorizer object at 0x7f72d22f5cc0> has no __sklearn_tags__ attribute, which is defined in `sklearn.base.BaseEstimator`. This will raise an error in scikit-learn 1.8. Please define the __sklearn_tags__ method, or inherit from `sklearn.base.BaseEstimator` and other appropriate mixins such as `sklearn.base.TransformerMixin`, `sklearn.base.ClassifierMixin`, `sklearn.base.RegressorMixin`, and `sklearn.base.ClusterMixin`, and `sklearn.base.OutlierMixin`.
warnings.warn(
/opt/hostedtoolcache/Python/3.13.0/x64/lib/python3.13/site-packages/sklearn/utils/_tags.py:322: FutureWarning: Estimator <mne.decoding.transformer.Vectorizer object at 0x7f72d22f5cc0> has no __sklearn_tags__ attribute, which is defined in `sklearn.base.BaseEstimator`. This will raise an error in scikit-learn 1.8. Please define the __sklearn_tags__ method, or inherit from `sklearn.base.BaseEstimator` and other appropriate mixins such as `sklearn.base.TransformerMixin`, `sklearn.base.ClassifierMixin`, `sklearn.base.RegressorMixin`, and `sklearn.base.ClusterMixin`, and `sklearn.base.OutlierMixin`.
warnings.warn(
Free resources๐
When you are done with a PlayerLSL
,
StreamLSL
or EpochsStream
, donโt
forget to free the resources they use to continuously mock an LSL stream or receive
new data from an LSL stream.
<EpochsStream OFF (n: 10 between (-0.2, 0.5 seconds)> connected to:
<Stream: ON | real-time-decoding (source: fb89dd4888a34fdeb03725dbf176cd35)>
<Stream: OFF | real-time-decoding (source: fb89dd4888a34fdeb03725dbf176cd35)>
<Player: real-time-decoding | OFF | /home/runner/mne_data/MNE-LSL-data/sample/mne-sample/sample_audvis_raw.fif>
Total running time of the script: (1 minutes 18.138 seconds)
Estimated memory usage: 606 MB