mne.find_events#
- mne.find_events(raw, stim_channel=None, output='onset', consecutive='increasing', min_duration=0, shortest_event=2, mask=None, uint_cast=False, mask_type='and', initial_event=False, verbose=None)[source]#
Find events from raw file.
See Parsing events from raw data and Working with events for more information about events.
- Parameters
- raw
Raw
object The raw data.
- stim_channel
None
|str
|list
ofstr
Name of the stim channel or all the stim channels affected by triggers. If None, the config variables ‘MNE_STIM_CHANNEL’, ‘MNE_STIM_CHANNEL_1’, ‘MNE_STIM_CHANNEL_2’, etc. are read. If these are not found, it will fall back to ‘STI 014’ if present, then fall back to the first channel of type ‘stim’, if present. If multiple channels are provided then the returned events are the union of all the events extracted from individual stim channels.
- output‘onset’ | ‘offset’ | ‘step’
Whether to report when events start, when events end, or both.
- consecutive
bool
| ‘increasing’ If True, consider instances where the value of the events channel changes without first returning to zero as multiple events. If False, report only instances where the value of the events channel changes from/to zero. If ‘increasing’, report adjacent events only when the second event code is greater than the first.
- min_duration
float
The minimum duration of a change in the events channel required to consider it as an event (in seconds).
- shortest_event
int
Minimum number of samples an event must last (default is 2). If the duration is less than this an exception will be raised.
- mask
int
|None
The value of the digital mask to apply to the stim channel values. If None (default), no masking is performed.
- uint_cast
bool
If True (default False), do a cast to
uint16
on the channel data. This can be used to fix a bug with STI101 and STI014 in Neuromag acquisition setups that use channel STI016 (channel 16 turns data into e.g. -32768), similar tomne_fix_stim14 --32
in MNE-C.New in v0.12.
- mask_type‘and’ | ‘not_and’
The type of operation between the mask and the trigger. Choose ‘and’ (default) for MNE-C masking behavior.
New in v0.13.
- initial_event
bool
If True (default False), an event is created if the stim channel has a value different from 0 as its first sample. This is useful if an event at t=0s is present.
New in v0.16.
- verbose
bool
|str
|int
|None
Control verbosity of the logging output. If
None
, use the default verbosity level. See the logging documentation andmne.verbose()
for details. Should only be passed as a keyword argument.
- raw
- Returns
- events
array
ofint
, shape (n_events, 3) The array of events. The first column contains the event time in samples, with first_samp included. The third column contains the event id.
- events
See also
find_stim_steps
Find all the steps in the stim channel.
read_events
Read events from disk.
write_events
Write events to disk.
Notes
Warning
If you are working with downsampled data, events computed before decimation are no longer valid. Please recompute your events after decimation, but note this reduces the precision of event timing.
Examples
Consider data with a stim channel that looks like:
[0, 32, 32, 33, 32, 0]
By default, find_events returns all samples at which the value of the stim channel increases:
>>> print(find_events(raw)) [[ 1 0 32] [ 3 32 33]]
If consecutive is False, find_events only returns the samples at which the stim channel changes from zero to a non-zero value:
>>> print(find_events(raw, consecutive=False)) [[ 1 0 32]]
If consecutive is True, find_events returns samples at which the event changes, regardless of whether it first returns to zero:
>>> print(find_events(raw, consecutive=True)) [[ 1 0 32] [ 3 32 33] [ 4 33 32]]
If output is ‘offset’, find_events returns the last sample of each event instead of the first one:
>>> print(find_events(raw, consecutive=True, ... output='offset')) [[ 2 33 32] [ 3 32 33] [ 4 0 32]]
If output is ‘step’, find_events returns the samples at which an event starts or ends:
>>> print(find_events(raw, consecutive=True, ... output='step')) [[ 1 0 32] [ 3 32 33] [ 4 33 32] [ 5 32 0]]
To ignore spurious events, it is also possible to specify a minimum event duration. Assuming our events channel has a sample rate of 1000 Hz:
>>> print(find_events(raw, consecutive=True, ... min_duration=0.002)) [[ 1 0 32]]
For the digital mask, if mask_type is set to ‘and’ it will take the binary representation of the digital mask, e.g. 5 -> ‘00000101’, and will allow the values to pass where mask is one, e.g.:
7 '0000111' <- trigger value 37 '0100101' <- mask ---------------- 5 '0000101'
For the digital mask, if mask_type is set to ‘not_and’ it will take the binary representation of the digital mask, e.g. 5 -> ‘00000101’, and will block the values where mask is one, e.g.:
7 '0000111' <- trigger value 37 '0100101' <- mask ---------------- 2 '0000010'
Examples using mne.find_events
#
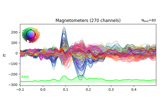
Working with CTF data: the Brainstorm auditory dataset
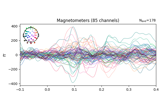
Preprocessing optically pumped magnetometer (OPM) MEG data
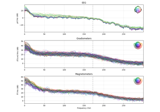
The Spectrum and EpochsSpectrum classes: frequency-domain data
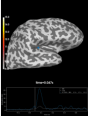
Source localization with MNE, dSPM, sLORETA, and eLORETA
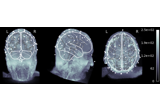
EEG source localization given electrode locations on an MRI
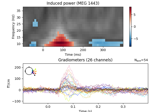
Non-parametric 1 sample cluster statistic on single trial power
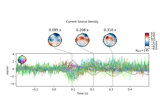
Transform EEG data using current source density (CSD)
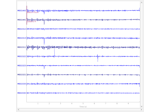
Plot sensor denoising using oversampled temporal projection
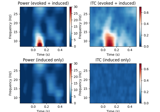
Compute power and phase lock in label of the source space
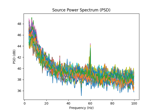
Compute source power spectral density (PSD) in a label
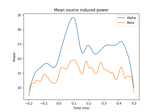
Compute induced power in the source space with dSPM
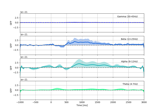
Explore event-related dynamics for specific frequency bands
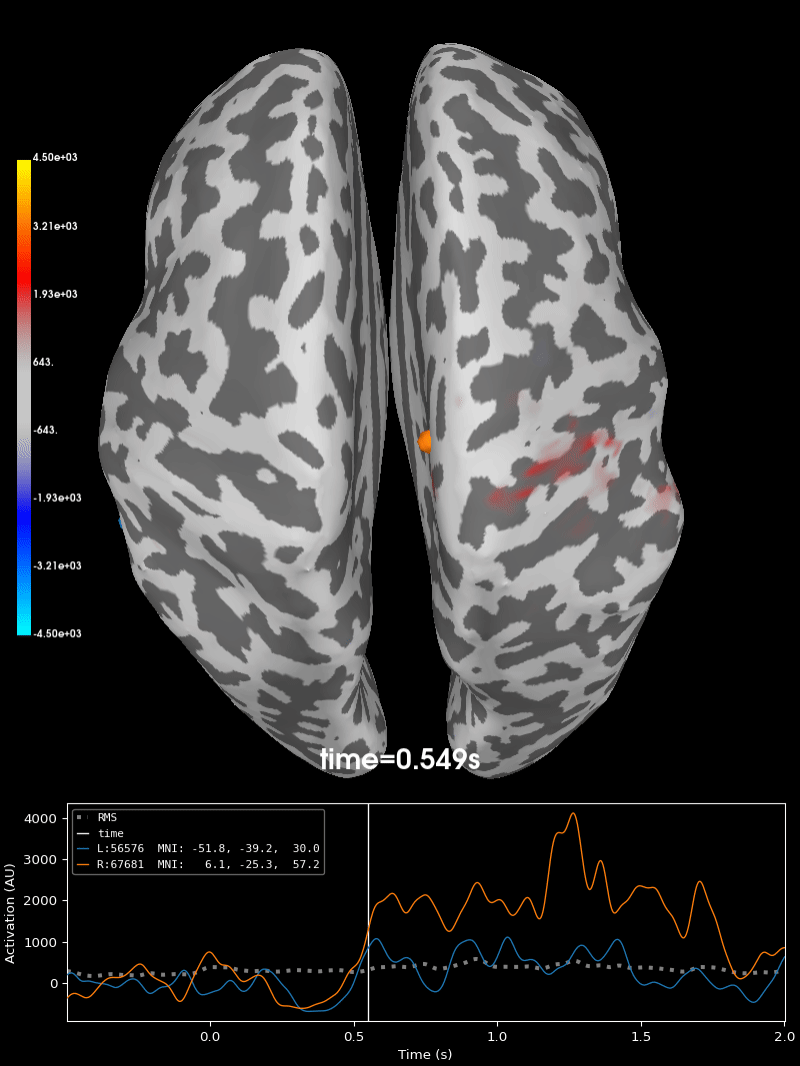
Compute source level time-frequency timecourses using a DICS beamformer
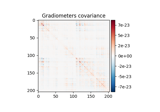
Compute evoked ERS source power using DICS, LCMV beamformer, and dSPM
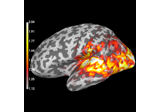
Compute source power estimate by projecting the covariance with MNE
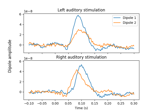
Computing source timecourses with an XFit-like multi-dipole model
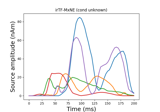
Compute iterative reweighted TF-MxNE with multiscale time-frequency dictionary