Note
Click here to download the full example code
Analysis of evoked response using ICA and PCA reduction techniques¶
This example computes PCA and ICA of evoked or epochs data. Then the PCA / ICA components, a.k.a. spatial filters, are used to transform the channel data to new sources / virtual channels. The output is visualized on the average of all the epochs.
# Authors: Jean-Remi King <jeanremi.king@gmail.com>
# Asish Panda <asishrocks95@gmail.com>
#
# License: BSD (3-clause)
import numpy as np
import matplotlib.pyplot as plt
import mne
from mne.datasets import sample
from mne.decoding import UnsupervisedSpatialFilter
from sklearn.decomposition import PCA, FastICA
print(__doc__)
# Preprocess data
data_path = sample.data_path()
# Load and filter data, set up epochs
raw_fname = data_path + '/MEG/sample/sample_audvis_filt-0-40_raw.fif'
event_fname = data_path + '/MEG/sample/sample_audvis_filt-0-40_raw-eve.fif'
tmin, tmax = -0.1, 0.3
event_id = dict(aud_l=1, aud_r=2, vis_l=3, vis_r=4)
raw = mne.io.read_raw_fif(raw_fname, preload=True)
raw.filter(1, 20, fir_design='firwin')
events = mne.read_events(event_fname)
picks = mne.pick_types(raw.info, meg=False, eeg=True, stim=False, eog=False,
exclude='bads')
epochs = mne.Epochs(raw, events, event_id, tmin, tmax, proj=False,
picks=picks, baseline=None, preload=True,
verbose=False)
X = epochs.get_data()
Out:
Opening raw data file /home/circleci/mne_data/MNE-sample-data/MEG/sample/sample_audvis_filt-0-40_raw.fif...
Read a total of 4 projection items:
PCA-v1 (1 x 102) idle
PCA-v2 (1 x 102) idle
PCA-v3 (1 x 102) idle
Average EEG reference (1 x 60) idle
Range : 6450 ... 48149 = 42.956 ... 320.665 secs
Ready.
Reading 0 ... 41699 = 0.000 ... 277.709 secs...
Filtering raw data in 1 contiguous segment
Setting up band-pass filter from 1 - 20 Hz
FIR filter parameters
---------------------
Designing a one-pass, zero-phase, non-causal bandpass filter:
- Windowed time-domain design (firwin) method
- Hamming window with 0.0194 passband ripple and 53 dB stopband attenuation
- Lower passband edge: 1.00
- Lower transition bandwidth: 1.00 Hz (-6 dB cutoff frequency: 0.50 Hz)
- Upper passband edge: 20.00 Hz
- Upper transition bandwidth: 5.00 Hz (-6 dB cutoff frequency: 22.50 Hz)
- Filter length: 497 samples (3.310 sec)
Transform data with PCA computed on the average ie evoked response
pca = UnsupervisedSpatialFilter(PCA(30), average=False)
pca_data = pca.fit_transform(X)
ev = mne.EvokedArray(np.mean(pca_data, axis=0),
mne.create_info(30, epochs.info['sfreq'],
ch_types='eeg'), tmin=tmin)
ev.plot(show=False, window_title="PCA", time_unit='s')
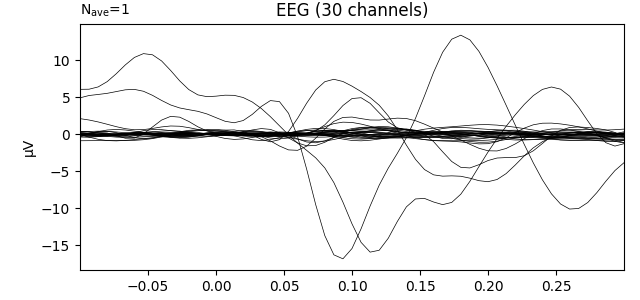
Transform data with ICA computed on the raw epochs (no averaging)
ica = UnsupervisedSpatialFilter(FastICA(30), average=False)
ica_data = ica.fit_transform(X)
ev1 = mne.EvokedArray(np.mean(ica_data, axis=0),
mne.create_info(30, epochs.info['sfreq'],
ch_types='eeg'), tmin=tmin)
ev1.plot(show=False, window_title='ICA', time_unit='s')
plt.show()
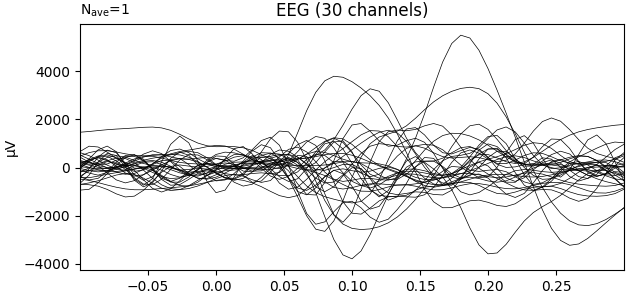
Total running time of the script: ( 0 minutes 8.559 seconds)
Estimated memory usage: 130 MB