Note
Click here to download the full example code
Compare the different ICA algorithms in MNE¶
Different ICA algorithms are fit to raw MEG data, and the corresponding maps are displayed.
Read and preprocess the data. Preprocessing consists of:
MEG channel selection
1-30 Hz band-pass filter
data_path = sample.data_path()
raw_fname = data_path + '/MEG/sample/sample_audvis_filt-0-40_raw.fif'
raw = mne.io.read_raw_fif(raw_fname, preload=True)
picks = mne.pick_types(raw.info, meg=True)
reject = dict(mag=5e-12, grad=4000e-13)
raw.filter(1, 30, fir_design='firwin')
Out:
Opening raw data file /home/circleci/mne_data/MNE-sample-data/MEG/sample/sample_audvis_filt-0-40_raw.fif...
Read a total of 4 projection items:
PCA-v1 (1 x 102) idle
PCA-v2 (1 x 102) idle
PCA-v3 (1 x 102) idle
Average EEG reference (1 x 60) idle
Range : 6450 ... 48149 = 42.956 ... 320.665 secs
Ready.
Reading 0 ... 41699 = 0.000 ... 277.709 secs...
Filtering raw data in 1 contiguous segment
Setting up band-pass filter from 1 - 30 Hz
FIR filter parameters
---------------------
Designing a one-pass, zero-phase, non-causal bandpass filter:
- Windowed time-domain design (firwin) method
- Hamming window with 0.0194 passband ripple and 53 dB stopband attenuation
- Lower passband edge: 1.00
- Lower transition bandwidth: 1.00 Hz (-6 dB cutoff frequency: 0.50 Hz)
- Upper passband edge: 30.00 Hz
- Upper transition bandwidth: 7.50 Hz (-6 dB cutoff frequency: 33.75 Hz)
- Filter length: 497 samples (3.310 sec)
Define a function that runs ICA on the raw MEG data and plots the components
def run_ica(method, fit_params=None):
ica = ICA(n_components=20, method=method, fit_params=fit_params,
max_iter='auto', random_state=0)
t0 = time()
ica.fit(raw, picks=picks, reject=reject)
fit_time = time() - t0
title = ('ICA decomposition using %s (took %.1fs)' % (method, fit_time))
ica.plot_components(title=title)
FastICA
run_ica('fastica')
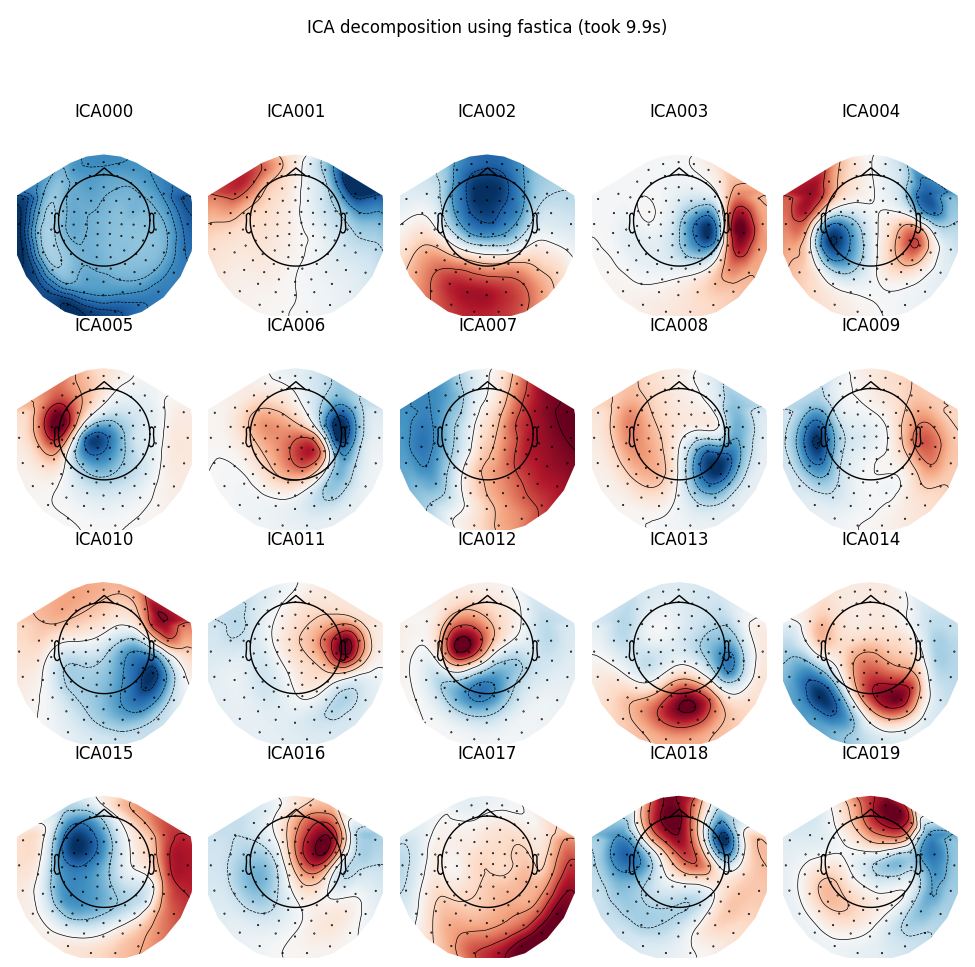
Out:
Fitting ICA to data using 305 channels (please be patient, this may take a while)
Rejecting epoch based on MAG : ['MEG 1711']
Artifact detected in [12642, 12943]
Rejecting epoch based on MAG : ['MEG 1711']
Artifact detected in [17458, 17759]
Selecting by number: 20 components
Fitting ICA took 9.9s.
Picard
run_ica('picard')
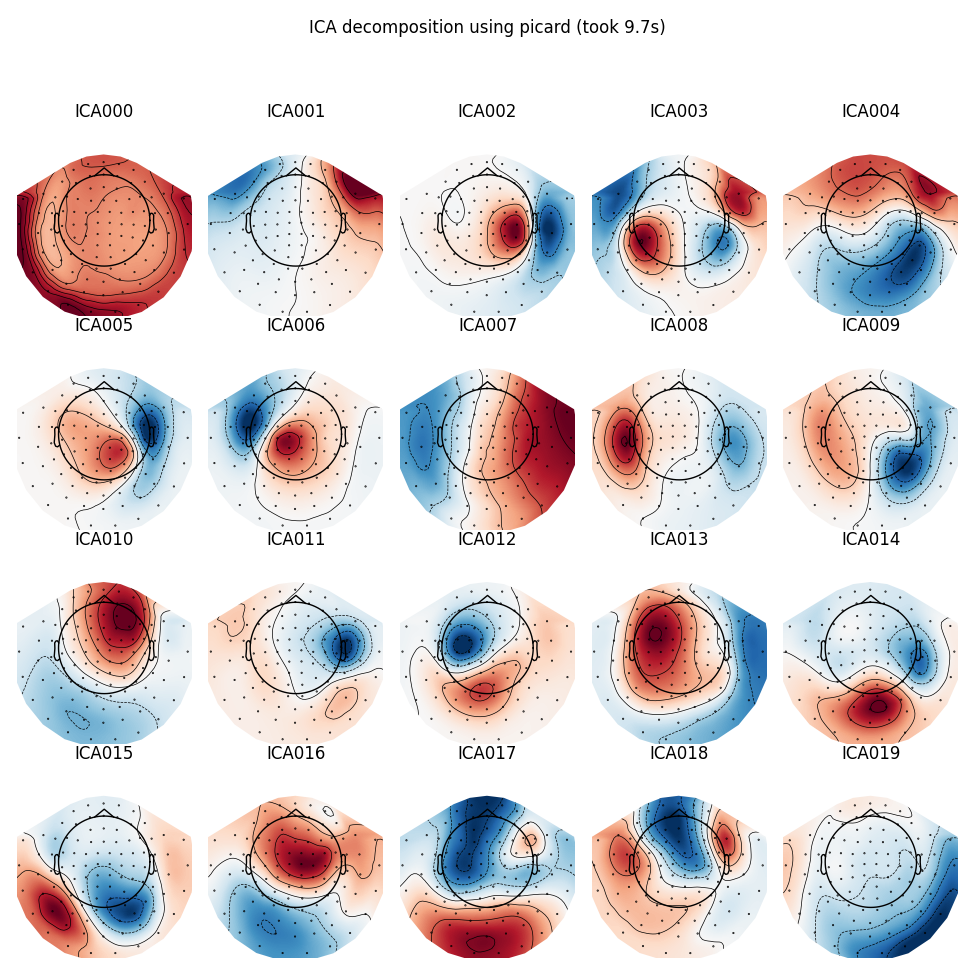
Out:
Fitting ICA to data using 305 channels (please be patient, this may take a while)
Rejecting epoch based on MAG : ['MEG 1711']
Artifact detected in [12642, 12943]
Rejecting epoch based on MAG : ['MEG 1711']
Artifact detected in [17458, 17759]
Selecting by number: 20 components
Fitting ICA took 9.7s.
Infomax
run_ica('infomax')
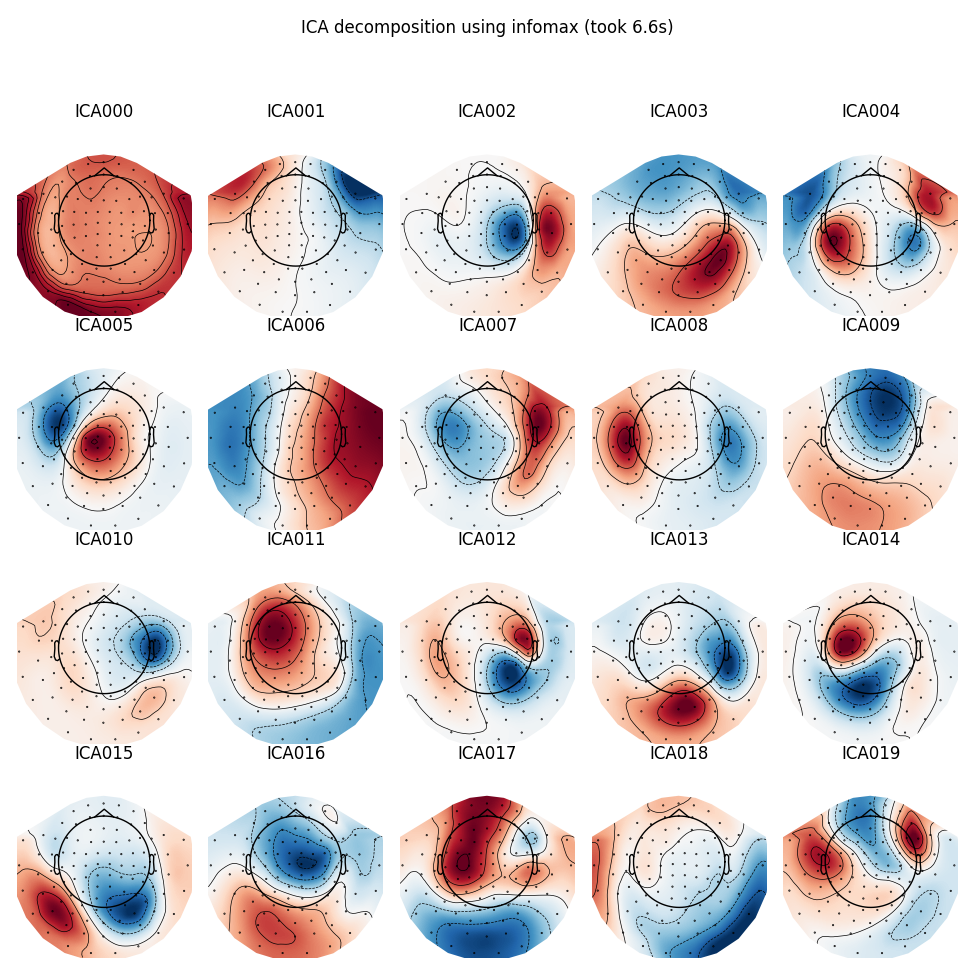
Out:
Fitting ICA to data using 305 channels (please be patient, this may take a while)
Rejecting epoch based on MAG : ['MEG 1711']
Artifact detected in [12642, 12943]
Rejecting epoch based on MAG : ['MEG 1711']
Artifact detected in [17458, 17759]
Selecting by number: 20 components
Fitting ICA took 6.6s.
Extended Infomax
run_ica('infomax', fit_params=dict(extended=True))
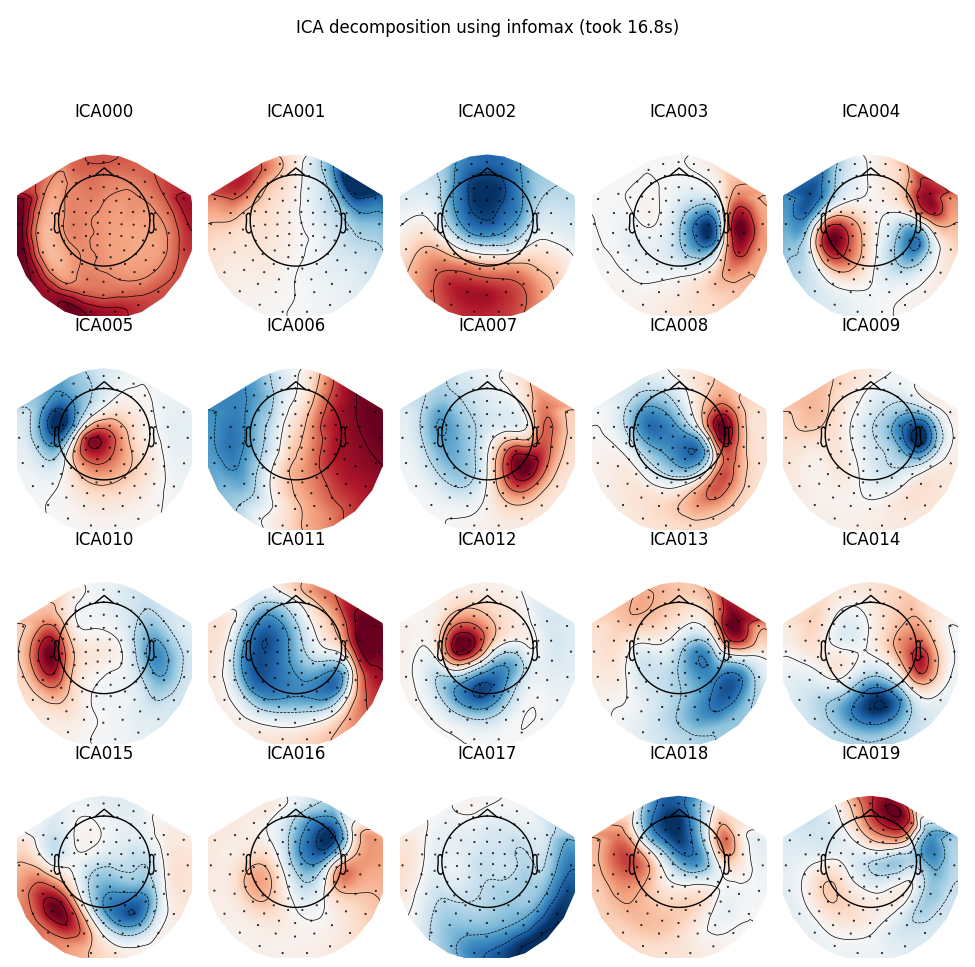
Out:
Fitting ICA to data using 305 channels (please be patient, this may take a while)
Rejecting epoch based on MAG : ['MEG 1711']
Artifact detected in [12642, 12943]
Rejecting epoch based on MAG : ['MEG 1711']
Artifact detected in [17458, 17759]
Selecting by number: 20 components
Computing Extended Infomax ICA
Fitting ICA took 16.8s.
Total running time of the script: ( 1 minutes 5.986 seconds)
Estimated memory usage: 511 MB