Note
Click here to download the full example code
EEG processing and Event Related Potentials (ERPs)¶
This tutorial shows how to perform standard ERP analyses in MNE-Python. Most of the material here is covered in other tutorials too, but for convenience the functions and methods most useful for ERP analyses are collected here, with links to other tutorials where more detailed information is given.
As usual we’ll start by importing the modules we need and loading some example data. Instead of parsing the events from the raw data’s stim channel (like we do in this tutorial), we’ll load the events from an external events file. Finally, to speed up computations so our documentation server can handle them, we’ll crop the raw data from ~4.5 minutes down to 90 seconds.
import os
import numpy as np
import pandas as pd
import matplotlib.pyplot as plt
import mne
sample_data_folder = mne.datasets.sample.data_path()
sample_data_raw_file = os.path.join(sample_data_folder, 'MEG', 'sample',
'sample_audvis_filt-0-40_raw.fif')
raw = mne.io.read_raw_fif(sample_data_raw_file, preload=False)
sample_data_events_file = os.path.join(sample_data_folder, 'MEG', 'sample',
'sample_audvis_filt-0-40_raw-eve.fif')
events = mne.read_events(sample_data_events_file)
raw.crop(tmax=90) # in seconds; happens in-place
# discard events >90 seconds (not strictly necessary: avoids some warnings)
events = events[events[:, 0] <= raw.last_samp]
Out:
Opening raw data file /home/circleci/mne_data/MNE-sample-data/MEG/sample/sample_audvis_filt-0-40_raw.fif...
Read a total of 4 projection items:
PCA-v1 (1 x 102) idle
PCA-v2 (1 x 102) idle
PCA-v3 (1 x 102) idle
Average EEG reference (1 x 60) idle
Range : 6450 ... 48149 = 42.956 ... 320.665 secs
Ready.
The file that we loaded has already been partially processed: 3D sensor
locations have been saved as part of the .fif
file, the data have been
low-pass filtered at 40 Hz, and a common average reference is set for the
EEG channels, stored as a projector (see Creating the average reference as a projector in the
Setting the EEG reference tutorial for more info about when you may want to do
this). We’ll discuss how to do each of these below.
Since this is a combined EEG+MEG dataset, let’s start by restricting the data to just the EEG and EOG channels. This will cause the other projectors saved in the file (which apply only to magnetometer channels) to be removed. By looking at the measurement info we can see that we now have 59 EEG channels and 1 EOG channel.
Out:
Removing projector <Projection | PCA-v1, active : False, n_channels : 102>
Removing projector <Projection | PCA-v2, active : False, n_channels : 102>
Removing projector <Projection | PCA-v3, active : False, n_channels : 102>
Reading 0 ... 13514 = 0.000 ... 90.001 secs...
Measurement date | December 03, 2002 19:01:10 GMT |
---|---|
Experimenter | Unknown | Participant | Unknown |
Digitized points | 146 points |
Good channels | 60 EEG, 1 EOG |
Bad channels | EEG 053 |
EOG channels | EOG 061 |
ECG channels | Not available |
Sampling frequency | 150.15 Hz |
Highpass | 0.10 Hz |
Lowpass | 40.00 Hz |
Projections | Average EEG reference: off |
Channel names and types¶
In practice it’s quite common to have some channels labelled as EEG that are
actually EOG channels. Raw
objects have a
set_channel_types
method that you can use to change a channel
that is labeled as eeg
into an eog
type. You can also rename channels
using the rename_channels
method. Detailed examples of both of
these methods can be found in the tutorial The Raw data structure: continuous data. In this data
the channel types are all correct already, so for now we’ll just rename the
channels to remove a space and a leading zero in the channel names, and
convert to lowercase:
channel_renaming_dict = {name: name.replace(' 0', '').lower()
for name in raw.ch_names}
_ = raw.rename_channels(channel_renaming_dict) # happens in-place
Channel locations¶
The tutorial Working with sensor locations describes MNE-Python’s handling of
sensor positions in great detail. To briefly summarize: MNE-Python
distinguishes montages (which contain sensor positions in
3D: x
, y
, z
, in meters) from layouts (which
define 2D arrangements of sensors for plotting approximate overhead diagrams
of sensor positions). Additionally, montages may specify idealized sensor
positions (based on, e.g., an idealized spherical headshape model) or they
may contain realistic sensor positions obtained by digitizing the 3D
locations of the sensors when placed on the actual subject’s head.
This dataset has realistic digitized 3D sensor locations saved as part of the
.fif
file, so we can view the sensor locations in 2D or 3D using the
plot_sensors
method:
raw.plot_sensors(show_names=True)
fig = raw.plot_sensors('3d')
If you’re working with a standard montage like the 10-20
system, you can add sensor locations to the data like this:
raw.set_montage('standard_1020')
. See Working with sensor locations for
info on what other standard montages are built-in to MNE-Python.
If you have digitized realistic sensor locations, there are dedicated
functions for loading those digitization files into MNE-Python; see
Reading sensor digitization files for discussion and Supported formats for digitized 3D locations for a list
of supported formats. Once loaded, the digitized sensor locations can be
added to the data by passing the loaded montage object to
raw.set_montage()
.
Setting the EEG reference¶
As mentioned above, this data already has an EEG common average reference added as a projector. We can view the effect of this on the raw data by plotting with and without the projector applied:
for proj in (False, True):
fig = raw.plot(n_channels=5, proj=proj, scalings=dict(eeg=50e-6))
fig.subplots_adjust(top=0.9) # make room for title
ref = 'Average' if proj else 'No'
fig.suptitle(f'{ref} reference', size='xx-large', weight='bold')
The referencing scheme can be changed with the function
mne.set_eeg_reference
(which by default operates on a copy of the data)
or the raw.set_eeg_reference()
method (which
always modifies the data in-place). The tutorial Setting the EEG reference shows
several examples of this.
Filtering¶
MNE-Python has extensive support for different ways of filtering data. For a general discussion of filter characteristics and MNE-Python defaults, see Background information on filtering. For practical examples of how to apply filters to your data, see Filtering and resampling data. Here, we’ll apply a simple high-pass filter for illustration:
raw.filter(l_freq=0.1, h_freq=None)
Out:
Filtering raw data in 1 contiguous segment
Setting up high-pass filter at 0.1 Hz
FIR filter parameters
---------------------
Designing a one-pass, zero-phase, non-causal highpass filter:
- Windowed time-domain design (firwin) method
- Hamming window with 0.0194 passband ripple and 53 dB stopband attenuation
- Lower passband edge: 0.10
- Lower transition bandwidth: 0.10 Hz (-6 dB cutoff frequency: 0.05 Hz)
- Filter length: 4957 samples (33.013 sec)
Measurement date | December 03, 2002 19:01:10 GMT |
---|---|
Experimenter | Unknown | Participant | Unknown |
Digitized points | 146 points |
Good channels | 60 EEG, 1 EOG |
Bad channels | eeg53 |
EOG channels | eog61 |
ECG channels | Not available |
Sampling frequency | 150.15 Hz |
Highpass | 0.10 Hz |
Lowpass | 40.00 Hz |
Projections | Average EEG reference: off |
Filenames | sample_audvis_filt-0-40_raw.fif |
Duration | 00:01:30 (HH:MM:SS) |
Evoked responses: epoching and averaging¶
The general process for extracting evoked responses from continuous data is
to use the Epochs
constructor, and then average the resulting epochs
to create an Evoked
object. In MNE-Python, events are represented as
a NumPy array
of sample numbers and integer event
codes. The event codes are stored in the last column of the events array:
The Working with events tutorial discusses event arrays in more detail.
Integer event codes are mapped to more descriptive text using a Python
dictionary
usually called event_id
. This mapping is
determined by your experiment code (i.e., it reflects which event codes you
chose to use to represent different experimental events or conditions). For
the Sample data has the following mapping:
event_dict = {'auditory/left': 1, 'auditory/right': 2, 'visual/left': 3,
'visual/right': 4, 'face': 5, 'buttonpress': 32}
Now we can extract epochs from the continuous data. An interactive plot
allows you to click on epochs to mark them as “bad” and drop them from the
analysis (it is not interactive on the documentation website, but will be
when you run epochs.plot()
in a Python console).
epochs = mne.Epochs(raw, events, event_id=event_dict, tmin=-0.3, tmax=0.7,
preload=True)
fig = epochs.plot(events=events)
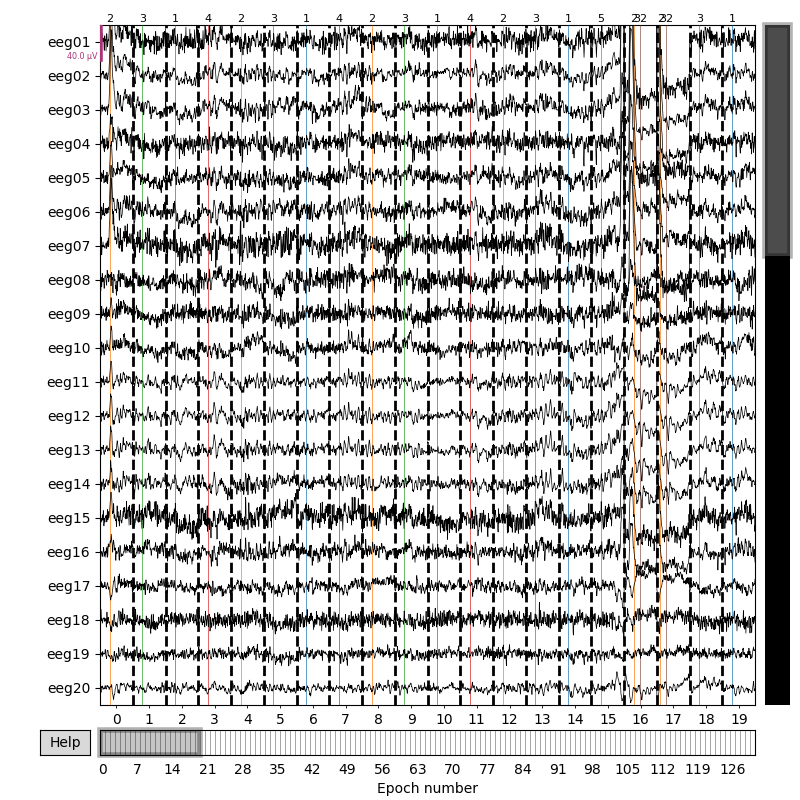
Out:
Not setting metadata
Not setting metadata
132 matching events found
Setting baseline interval to [-0.2996928197375818, 0.0] sec
Applying baseline correction (mode: mean)
Created an SSP operator (subspace dimension = 1)
1 projection items activated
Loading data for 132 events and 151 original time points ...
1 bad epochs dropped
You seem to have overlapping epochs. Some event lines may be duplicated in the plot.
It is also possible to automatically drop epochs, when first creating them or
later on, by providing maximum peak-to-peak signal value thresholds (pass to
the Epochs
constructor as the reject
parameter; see
Rejecting Epochs based on channel amplitude for details). You can also do this after
the epochs are already created, using the drop_bad
method:
reject_criteria = dict(eeg=100e-6, # 100 µV
eog=200e-6) # 200 µV
_ = epochs.drop_bad(reject=reject_criteria)
Out:
Rejecting epoch based on EEG : ['eeg03']
Rejecting epoch based on EEG : ['eeg01', 'eeg02', 'eeg03', 'eeg04', 'eeg06', 'eeg07']
Rejecting epoch based on EEG : ['eeg01', 'eeg02', 'eeg03', 'eeg04', 'eeg06', 'eeg07']
Rejecting epoch based on EEG : ['eeg01', 'eeg02', 'eeg03', 'eeg04', 'eeg06', 'eeg07']
Rejecting epoch based on EEG : ['eeg01', 'eeg02', 'eeg03', 'eeg07']
Rejecting epoch based on EEG : ['eeg01', 'eeg02', 'eeg03', 'eeg07']
Rejecting epoch based on EEG : ['eeg01']
Rejecting epoch based on EEG : ['eeg03', 'eeg07']
Rejecting epoch based on EEG : ['eeg03', 'eeg07']
Rejecting epoch based on EEG : ['eeg07']
Rejecting epoch based on EEG : ['eeg03', 'eeg07']
Rejecting epoch based on EEG : ['eeg03', 'eeg07']
Rejecting epoch based on EEG : ['eeg07']
Rejecting epoch based on EEG : ['eeg07']
Rejecting epoch based on EEG : ['eeg03']
Rejecting epoch based on EEG : ['eeg25']
Rejecting epoch based on EEG : ['eeg25']
17 bad epochs dropped
Next we generate a barplot of which channels contributed most to epochs
getting rejected. If one channel is responsible for lots of epoch rejections,
it may be worthwhile to mark that channel as “bad” in the Raw
object and then re-run epoching (fewer channels w/ more good epochs may be
preferable to keeping all channels but losing many epochs). See
Handling bad channels for more info.
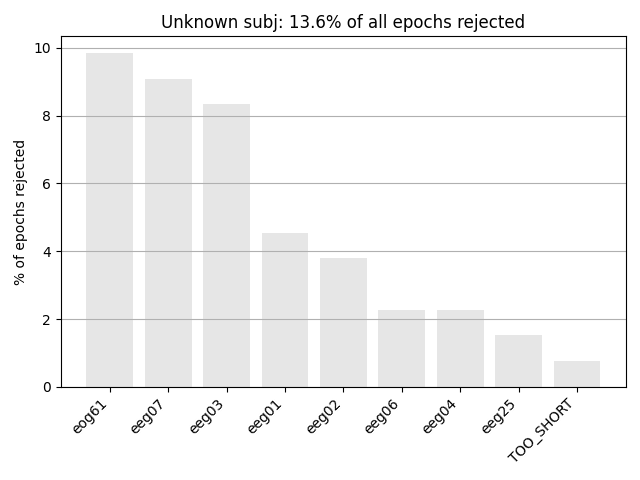
Another way in which epochs can be automatically dropped is if the event
around which the epoch is formed is too close to the start or end of the
Raw
object (e.g., if the epoch’s tmax
would be past the end of
the file; this is the cause of the “TOO_SHORT” entry in the
plot_drop_log
plot above). Epochs may also be automatically
dropped if the Raw
object contains annotations that begin
with either bad
or edge
(“edge” annotations are automatically
inserted when concatenating two separate Raw
objects together). See
Rejecting bad data spans and breaks for more information about annotation-based
epoch rejection.
Now that we’ve dropped the bad epochs, let’s look at our evoked responses for
some conditions we care about. Here the average
method will
create an Evoked
object, which we can then plot. Notice that we
select which condition we want to average using the square-bracket indexing
(like a dictionary
); that returns a smaller epochs object
containing just the epochs from that condition, to which we then apply the
average
method:
These Evoked
objects have their own interactive plotting method
(though again, it won’t be interactive on the documentation website):
click-dragging a span of time will generate a scalp field topography for that
time span. Here we also demonstrate built-in color-coding the channel traces
by location:
fig1 = l_aud.plot()
fig2 = l_vis.plot(spatial_colors=True)
Scalp topographies can also be obtained non-interactively with the
plot_topomap
method. Here we display topomaps of the average
field in 50 ms time windows centered at -200 ms, 100 ms, and 400 ms.
l_aud.plot_topomap(times=[-0.2, 0.1, 0.4], average=0.05)
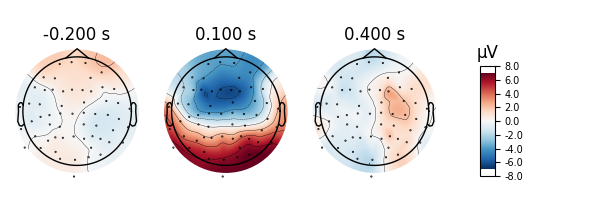
Considerable customization of these plots is possible, see the docstring of
plot_topomap
for details.
There is also a built-in method for combining “butterfly” plots of the
signals with scalp topographies, called plot_joint
. Like
plot_topomap
you can specify times for the scalp topographies
or you can let the method choose times automatically, as is done here:
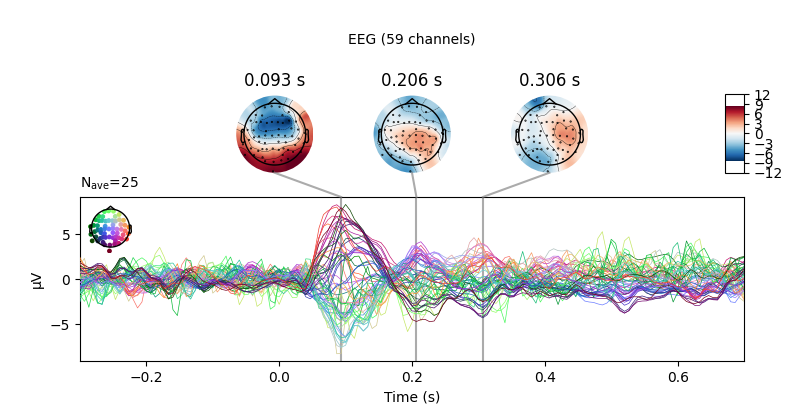
Out:
Projections have already been applied. Setting proj attribute to True.
Global field power (GFP)¶
Global field power 123 is, generally speaking, a measure of agreement of the signals picked up by all sensors across the entire scalp: if all sensors have the same value at a given time point, the GFP will be zero at that time point; if the signals differ, the GFP will be non-zero at that time point. GFP peaks may reflect “interesting” brain activity, warranting further investigation. Mathematically, the GFP is the population standard deviation across all sensors, calculated separately for every time point.
You can plot the GFP using evoked.plot(gfp=True)
. The GFP
trace will be black if spatial_colors=True
and green otherwise. The EEG
reference does not affect the GFP:
To plot the GFP by itself you can pass gfp='only'
(this makes it easier
to read off the GFP data values, because the scale is aligned):
l_aud.plot(gfp='only')
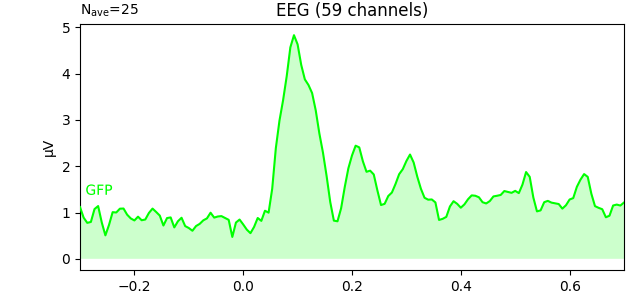
As stated above, the GFP is the population standard deviation of the signal
across channels. To compute it manually, we can leverage the fact that
evoked.data
is a NumPy array
,
and verify by plotting it using matplotlib commands:
gfp = l_aud.data.std(axis=0, ddof=0)
# Reproducing the MNE-Python plot style seen above
fig, ax = plt.subplots()
ax.plot(l_aud.times, gfp * 1e6, color='lime')
ax.fill_between(l_aud.times, gfp * 1e6, color='lime', alpha=0.2)
ax.set(xlabel='Time (s)', ylabel='GFP (µV)', title='EEG')
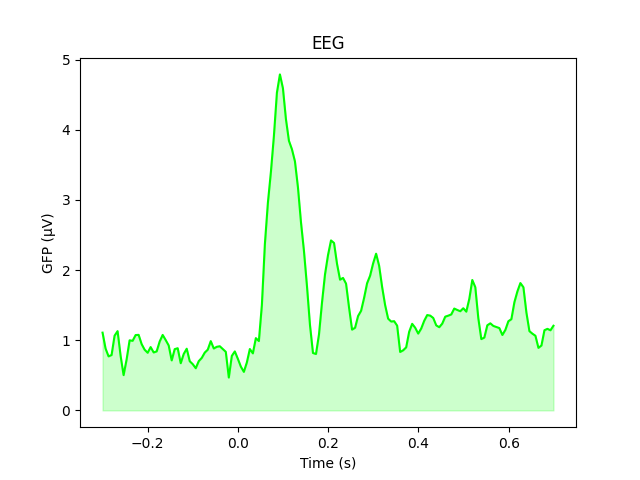
Analyzing regions of interest (ROIs): averaging across channels¶
Since our sample data is responses to left and right auditory and visual stimuli, we may want to compare left versus right ROIs. To average across channels in a region of interest, we first find the channel indices we want. Looking back at the 2D sensor plot above, we might choose the following for left and right ROIs:
left = ['eeg17', 'eeg18', 'eeg25', 'eeg26']
right = ['eeg23', 'eeg24', 'eeg34', 'eeg35']
left_ix = mne.pick_channels(l_aud.info['ch_names'], include=left)
right_ix = mne.pick_channels(l_aud.info['ch_names'], include=right)
Now we can create a new Evoked with 2 virtual channels (one for each ROI):
roi_dict = dict(left_ROI=left_ix, right_ROI=right_ix)
roi_evoked = mne.channels.combine_channels(l_aud, roi_dict, method='mean')
print(roi_evoked.info['ch_names'])
roi_evoked.plot()
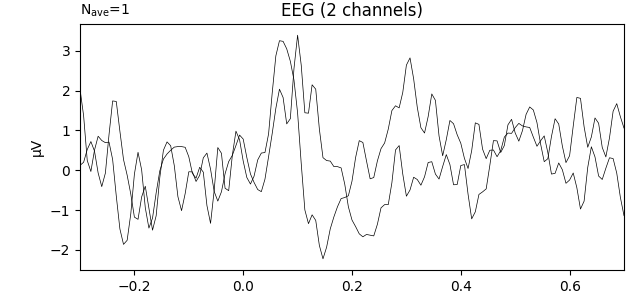
Out:
['left_ROI', 'right_ROI']
Comparing conditions¶
If we wanted to compare our auditory and visual stimuli, a useful function is
mne.viz.plot_compare_evokeds
. By default this will combine all channels in
each evoked object using global field power (or RMS for MEG channels); here
instead we specify to combine by averaging, and restrict it to a subset of
channels by passing picks
:
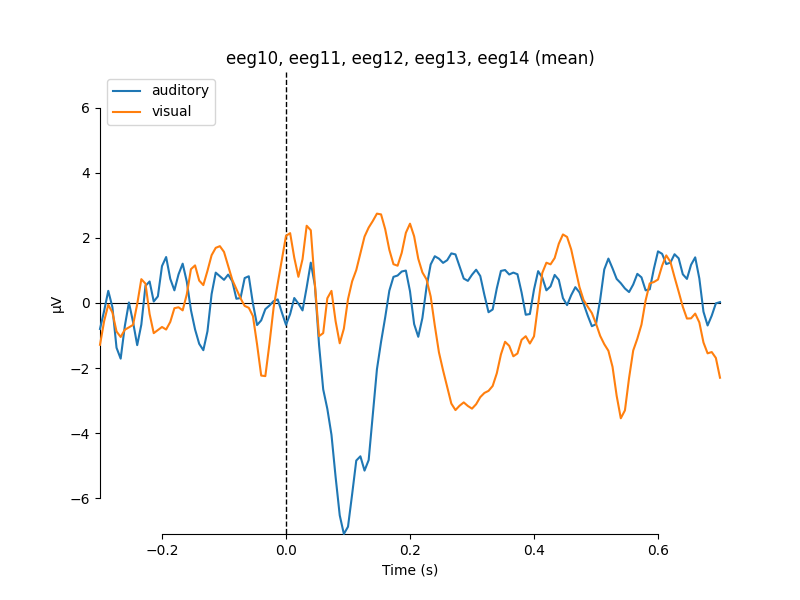
Out:
combining channels using "mean"
combining channels using "mean"
We can also easily get confidence intervals by treating each epoch as a
separate observation using the iter_evoked
method. A confidence
interval across subjects could also be obtained, by passing a list of
Evoked
objects (one per subject) to the
plot_compare_evokeds
function.
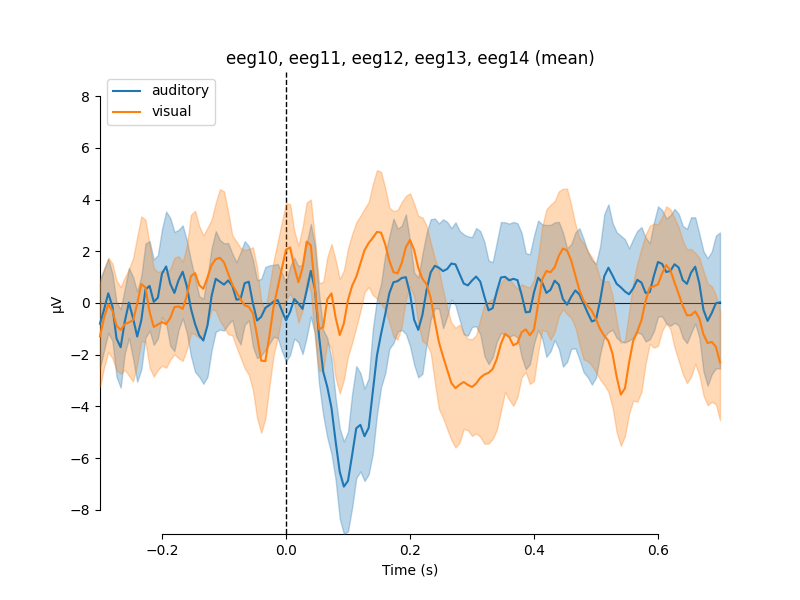
Out:
combining channels using "mean"
combining channels using "mean"
We can also compare conditions by subtracting one Evoked
object from
another using the mne.combine_evoked
function (this function also allows
pooling of epochs without subtraction).
aud_minus_vis = mne.combine_evoked([l_aud, l_vis], weights=[1, -1])
aud_minus_vis.plot_joint()
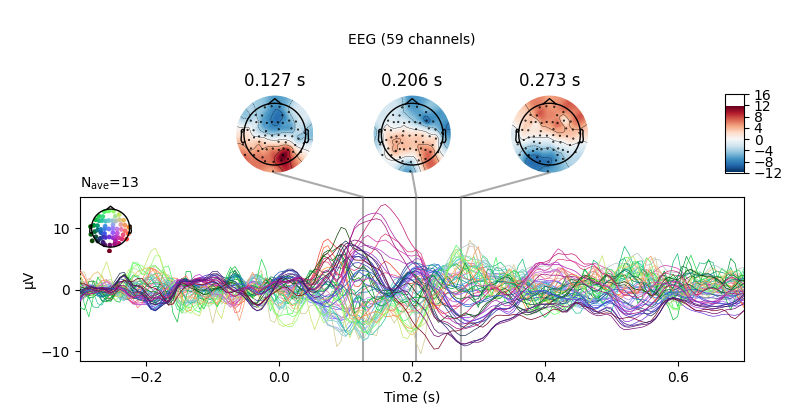
Out:
Projections have already been applied. Setting proj attribute to True.
Warning
The code above yields an equal-weighted difference. If you have
imbalanced trial numbers, you might want to equalize the number of events
per condition first by using epochs.equalize_event_counts()
before averaging.
Grand averages¶
To compute grand averages across conditions (or subjects), you can pass a
list of Evoked
objects to mne.grand_average
. The result is another
Evoked
object.
grand_average = mne.grand_average([l_aud, l_vis])
print(grand_average)
Out:
Interpolating bad channels
Automatic origin fit: head of radius 91.0 mm
Computing interpolation matrix from 59 sensor positions
Interpolating 1 sensors
Interpolating bad channels
Automatic origin fit: head of radius 91.0 mm
Computing interpolation matrix from 59 sensor positions
Interpolating 1 sensors
Identifying common channels ...
<Evoked | 'Grand average (n = 2)' (average, N=2), -0.29969 – 0.69928 sec, baseline -0.299693 – 0 sec, 60 ch, ~3.0 MB>
For combining conditions it is also possible to make use of HED tags in the condition names when selecting which epochs to average. For example, we have the condition names:
list(event_dict)
We can select the auditory conditions (left and right together) by passing:
epochs['auditory'].average()
see Subselecting epochs for details.
The tutorials The Epochs data structure: discontinuous data and The Evoked data structure: evoked/averaged data have many
more details about working with the Epochs
and Evoked
classes.
Amplitude and latency measures¶
It is common in ERP research to extract measures of amplitude or latency to compare across different conditions. There are many measures that can be extracted from ERPs, and many of these are detailed (including the respective strengths and weaknesses) in chapter 9 of Luck 4 (also see the Measurement Tool in the ERPLAB Toolbox 5).
This part of the tutorial will demonstrate how to extract three common measures:
Peak latency
Peak amplitude
Mean amplitude
Peak latency and amplitude¶
The most common measures of amplitude and latency are peak measures. Peak measures are basically the maximum amplitude of the signal in a specified time window and the time point (or latency) at which the peak amplitude occurred.
Peak measures can be obtained using the get_peak()
method.
There are two important things to point out about
get_peak()
method. First, it finds the strongest peak
looking across all channels of the selected type that are available in
the Evoked
object. As a consequence, if you want to restrict
the search for the peak to a group of channels or a single channel, you
should first use the pick()
or
pick_channels()
methods. Second, the
get_peak()
method can find different types of peaks using
the mode
argument. There are three options:
mode='pos'
: finds the peak with a positive voltage (ignores negative voltages)mode='neg'
: finds the peak with a negative voltage (ignores positive voltages)mode='abs'
: finds the peak with the largest absolute voltage regardless of sign (positive or negative)
The following example demonstrates how to find the first positive peak in the
ERP (i.e., the P100) for the left visual condition (i.e., the
l_vis
Evoked
object). The time window used to search for
the peak ranges from .08 to .12 s. This time window was selected because it
is when P100 typically occurs. Note that all 'eeg'
channels are submitted
to the get_peak()
method.
# Define a function to print out the channel (ch) containing the
# peak latency (lat; in msec) and amplitude (amp, in µV), with the
# time range (tmin and tmax) that were searched.
# This function will be used throughout the remainder of the tutorial
def print_peak_measures(ch, tmin, tmax, lat, amp):
print(f'Channel: {ch}')
print(f'Time Window: {tmin * 1e3:.3f} - {tmax * 1e3:.3f} ms')
print(f'Peak Latency: {lat * 1e3:.3f} ms')
print(f'Peak Amplitude: {amp * 1e6:.3f} µV')
# Get peak amplitude and latency from a good time window that contains the peak
good_tmin, good_tmax = .08, .12
ch, lat, amp = l_vis.get_peak(ch_type='eeg', tmin=good_tmin, tmax=good_tmax,
mode='pos', return_amplitude=True)
# Print output from the good time window that contains the peak
print('** PEAK MEASURES FROM A GOOD TIME WINDOW **')
print_peak_measures(ch, good_tmin, good_tmax, lat, amp)
Out:
** PEAK MEASURES FROM A GOOD TIME WINDOW **
Channel: eeg55
Time Window: 80.000 - 120.000 ms
Peak Latency: 86.578 ms
Peak Amplitude: 6.508 µV
The output shows that channel eeg55
had the maximum positive peak in
the chosen time window from all of the 'eeg'
channels searched.
In practice, one might want to pull out the peak for
an a priori region of interest or a single channel depending on the study.
This can be done by combining the pick()
or pick_channels()
methods with the
get_peak()
method.
Here, let’s assume we believe the effects of interest will occur
at eeg59
.
# Fist, return a copy of l_vis to select the channel from
l_vis_roi = l_vis.copy().pick('eeg59')
# Get the peak and latency measure from the selected channel
ch_roi, lat_roi, amp_roi = l_vis_roi.get_peak(
tmin=good_tmin, tmax=good_tmax, mode='pos', return_amplitude=True)
# Print output
print('** PEAK MEASURES FOR ONE CHANNEL FROM A GOOD TIME WINDOW **')
print_peak_measures(ch_roi, good_tmin, good_tmax, lat_roi, amp_roi)
Out:
** PEAK MEASURES FOR ONE CHANNEL FROM A GOOD TIME WINDOW **
Channel: eeg59
Time Window: 80.000 - 120.000 ms
Peak Latency: 86.578 ms
Peak Amplitude: 5.713 µV
While the peak latencies are the same in channels eeg55
and eeg59
,
the peak amplitudes differ. This approach can also be applied to virtual
channels created with the combine_channels()
function and
difference waves created with the mne.combine_evoked()
function (see
aud_minus_vis
in section Comparing conditions above).
Peak measures are very susceptible to high frequency noise in the signal (for discussion, see 4). Specifically, high frequency noise positively bias peak amplitude measures. This bias can confound comparisons across conditions where ERPs differ in the level of high frequency noise, such as when the conditions differ in the number of trials contributing to the ERP. One way to avoid this is to apply a non-causal low-pass filter to the ERP. Low-pass filters reduce the contribution of high frequency noise by smoothing out fast (i.e., high frequency) fluctuations in the signal (see Background information on filtering). While this can reduce the positive bias in peak amplitude measures caused by high frequency noise, low-pass filtering the ERP can introduce challenges in interpreting peak latency measures for effects of interest 67.
If using peak measures, it is critical to visually inspect the data to
make sure the selected time window actually contains a peak
(get_peak()
will always identify a peak).
Visual inspection allows to easily verify whether the automatically found
peak is correct. The get_peak()
detects the maximum or
minimum voltage in the specified time range and returns the latency and
amplitude of this peak. There is no guarantee that this method will return
an actual peak. Instead, it may return a value on the rising or falling edge
of the peak we are trying to find.
The following example demonstrates why visual inspection is crucial. Below,
we use a known bad time window (.095 to .135 s) to search for a peak in
channel eeg59
.
# Get BAD peak measures
bad_tmin, bad_tmax = .095, .135
ch_roi, bad_lat_roi, bad_amp_roi = l_vis_roi.get_peak(
mode='pos', tmin=bad_tmin, tmax=bad_tmax, return_amplitude=True)
# Print output
print('** PEAK MEASURES FOR ONE CHANNEL FROM A BAD TIME WINDOW **')
print_peak_measures(ch_roi, bad_tmin, bad_tmax, bad_lat_roi, bad_amp_roi)
Out:
** PEAK MEASURES FOR ONE CHANNEL FROM A BAD TIME WINDOW **
Channel: eeg59
Time Window: 95.000 - 135.000 ms
Peak Latency: 99.898 ms
Peak Amplitude: 1.487 µV
If all we had were the above values, it would be unclear if they are truly
identifying a peak or just a the falling or rising edge of one. However, it
becomes clear that the .095 to .135 s time window is misses the peak on
eeg59
. This is shown in the bottom panel where we see the bad time window
(highlighted in orange) misses the peak (the pink star). In contrast, the
time window defined initially (.08 to .12 s; highlighted in blue) returns
an actual peak instead of a just a maximal or minimal value in the searched
time window. Visual inspection will always help you to convince yourself the
data returned are actual peaks.
fig, axs = plt.subplots(nrows=2, ncols=1)
words = (('Bad', 'missing'), ('Good', 'finding'))
times = (np.array([bad_tmin, bad_tmax]), np.array([good_tmin, good_tmax]))
colors = ('C1', 'C0')
for ix, ax in enumerate(axs):
title = '{} time window {} peak'.format(*words[ix])
l_vis_roi.plot(axes=ax, time_unit='ms', show=False, titles=title)
ax.plot(lat_roi * 1e3, amp_roi * 1e6, marker='*', color='C6')
ax.axvspan(*(times[ix] * 1e3), facecolor=colors[ix], alpha=0.3)
ax.set_xlim(-50, 150) # Show zoomed in around peak
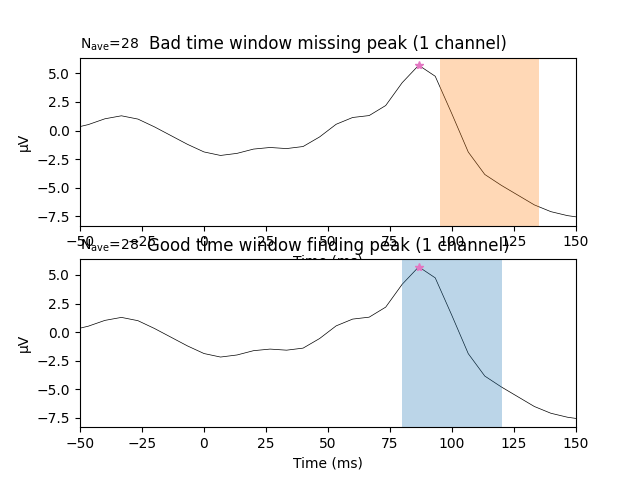
Out:
Need more than one channel to make topography for eeg. Disabling interactivity.
Need more than one channel to make topography for eeg. Disabling interactivity.
Mean Amplitude¶
Another common practice in ERP studies is to define a component (or effect) as the mean amplitude within a specified time window. One advantage of this approach is that it is less sensitive to high frequency noise (compared to peak amplitude measures) because averaging over a time window acts like a low-pass filter (see discussion in the above section Peak latency and amplitude).
When using mean amplitude measures, selecting the time window based on the effect of interest (e.g., the difference between two conditions) can inflate the likelihood of finding false positives in your results because this approach is circular 8. There are other, and better, ways to identify a time window to use for extracting mean amplitude measures. First, you can use a priori time window based on prior research. A second way is to define a time window from an independent condition or set of trials not used in the analysis (e.g., a “localizer”). A third approach is to define a time window using the across-condition grand average. This latter approach is not circular because the across-condition mean and condition difference are independent of one another. The issues discussed above also apply to selecting channels used for analysis.
The following example demonstrates how to pull out the mean amplitude
from the left visual condition (i.e., the l_vis
Evoked
object) using from selected channels and time windows. Stimulating the
left visual field is increases neural activity visual cortex of the
contralateral (i.e., right) hemisphere. We can test this by examining the
amplitude of the ERP for left visual field stimulation over right
(contralateral) and left (ipsilateral) channels. The channels used for this
analysis are eeg54
and eeg57
(left hemisphere), and eeg59
and
eeg55
(right hemisphere). The time window used is .08 (good_tmin
)
to .12 s (good_tmax
) as it corresponds to when P100 typically occurs. The
P100 is sensitive to left and right visual field stimulation. The mean
amplitude is extracted from the above four channels and stored in a
pandas.DataFrame
.
# Select all of the channels and crop to the time window
channels = ['eeg54', 'eeg57', 'eeg55', 'eeg59']
hemisphere = ['left', 'left', 'right', 'right']
l_vis_mean_roi = l_vis.copy().pick(channels).crop(
tmin=good_tmin, tmax=good_tmax)
# Extract mean amplitude in µV over time
mean_amp_roi = l_vis_mean_roi.data.mean(axis=1) * 1e6
# Store the data in a data frame
mean_amp_roi_df = pd.DataFrame({
'ch_name': l_vis_mean_roi.ch_names,
'hemisphere': ['left', 'left', 'right', 'right'],
'mean_amp': mean_amp_roi
})
# Print the data frame
print(mean_amp_roi_df.groupby('hemisphere').mean())
Out:
mean_amp
hemisphere
left -0.279764
right 0.685840
As demonstrated in the above example, the mean amplitude was higher and positive in right compared to left hemisphere channels. It should be reiterated that both that spatial and temporal window you use should be determined in an independent manner (e.g., defined a priori from prior research, a “localizer” or another independent condition) and not based on the data you will use to test your hypotheses.
The above example can be modified to extract the the mean amplitude
from all channels and store the resulting output in
pandas.DataFrame
. This can be useful for statistical analyses
conducted in other programming languages.
# Extract mean amplitude for all channels in l_vis (including `eog`)
l_vis_cropped = l_vis.copy().crop(tmin=good_tmin, tmax=good_tmax)
mean_amp_all = l_vis_cropped.data.mean(axis=1) * 1e6
mean_amp_all_df = pd.DataFrame({
'ch_name': l_vis_cropped.info['ch_names'],
'mean_amp': mean_amp_all
})
mean_amp_all_df['tmin'] = good_tmin
mean_amp_all_df['tmax'] = good_tmax
mean_amp_all_df['condition'] = 'Left/Visual'
print(mean_amp_all_df.head())
print(mean_amp_all_df.tail())
Out:
ch_name ... condition
0 eeg01 ... Left/Visual
1 eeg02 ... Left/Visual
2 eeg03 ... Left/Visual
3 eeg04 ... Left/Visual
4 eeg05 ... Left/Visual
[5 rows x 5 columns]
ch_name ... condition
55 eeg56 ... Left/Visual
56 eeg57 ... Left/Visual
57 eeg58 ... Left/Visual
58 eeg59 ... Left/Visual
59 eeg60 ... Left/Visual
[5 rows x 5 columns]
References¶
- 1
Dietrich Lehmann and Wolfgang Skrandies. Reference-free identification of components of checkerboard-evoked multichannel potential fields. Electroencephalography and Clinical Neurophysiology, 48(6):609–621, 1980. doi:10.1016/0013-4694(80)90419-8.
- 2
Dietrich Lehmann and Wolfgang Skrandies. Spatial analysis of evoked potentials in man—a review. Progress in Neurobiology, 23(3):227–250, 1984. doi:10.1016/0301-0082(84)90003-0.
- 3
Micah M. Murray, Denis Brunet, and Christoph M. Michel. Topographic ERP analyses: A step-by-step tutorial review. Brain Topography, 20(4):249–264, 2008. doi:10.1007/s10548-008-0054-5.
- 4(1,2)
Steven J Luck. An Introduction to the Event-Related Potential Technique. The MIT Press, Cambridge, MA, 2nd edition, 2014. ISBN 978-0-262-52585-5. URL: https://mitpress.mit.edu/books/introduction-event-related-potential-technique-second-edition.
- 5
Javier Lopez-Calderon and Steven J. Luck. Erplab: an open-source toolbox for the analysis of event-related potentials. Frontiers in Human Neuroscience, 2014. doi:10.3389/fnhum.2014.00213.
- 6
Guillaume A. Rousselet. Does filtering preclude us from studying ERP time-courses? Frontiers in Psychology, 2012. doi:10.3389/fpsyg.2012.00131.
- 7
Rufin VanRullen. Four common conceptual fallacies in mapping the time course of recognition. Frontiers in Psychology, 2011. doi:10.3389/fpsyg.2011.00365.
- 8
Steven J. Luck and Nicholas Gaspelin. How to get statistically significant effects in any ERP experiment (and why you shouldn’t). Psychophysiology, 54(1):146–157, 2017. doi:10.1111/psyp.12639.
Total running time of the script: ( 0 minutes 35.291 seconds)
Estimated memory usage: 9 MB