mne.decoding.Vectorizer#
- class mne.decoding.Vectorizer[source]#
Transform n-dimensional array into 2D array of n_samples by n_features.
This class reshapes an n-dimensional array into an n_samples * n_features array, usable by the estimators and transformers of scikit-learn.
Examples
- clf = make_pipeline(SpatialFilter(), _XdawnTransformer(), Vectorizer(),
LogisticRegression())
- Attributes:
- features_shape_
tuple
Stores the original shape of data.
- features_shape_
Methods
fit
(X[, y])Store the shape of the features of X.
fit_transform
(X[, y])Fit the data, then transform in one step.
Transform 2D data back to its original feature shape.
transform
(X)Convert given array into two dimensions.
- fit(X, y=None)[source]#
Store the shape of the features of X.
- Parameters:
- Xarray_like
The data to fit. Can be, for example a list, or an array of at least 2d. The first dimension must be of length n_samples, where samples are the independent samples used by the estimator (e.g. n_epochs for epoched data).
- y
None
|array
, shape (n_samples,) Used for scikit-learn compatibility.
- Returns:
- selfinstance of
Vectorizer
Return the modified instance.
- selfinstance of
- fit_transform(X, y=None)[source]#
Fit the data, then transform in one step.
- Parameters:
- Xarray_like
The data to fit. Can be, for example a list, or an array of at least 2d. The first dimension must be of length n_samples, where samples are the independent samples used by the estimator (e.g. n_epochs for epoched data).
- y
None
|array
, shape (n_samples,) Used for scikit-learn compatibility.
- Returns:
- X
array
, shape (n_samples, -1) The transformed data.
- X
- inverse_transform(X)[source]#
Transform 2D data back to its original feature shape.
- Parameters:
- Xarray_like, shape (n_samples, n_features)
Data to be transformed back to original shape.
- Returns:
- X
array
The data transformed into shape as used in fit. The first dimension is of length n_samples.
- X
- transform(X)[source]#
Convert given array into two dimensions.
- Parameters:
- Xarray_like
The data to fit. Can be, for example a list, or an array of at least 2d. The first dimension must be of length n_samples, where samples are the independent samples used by the estimator (e.g. n_epochs for epoched data).
- Returns:
- X
array
, shape (n_samples, n_features) The transformed data.
- X
Examples using mne.decoding.Vectorizer
#
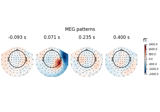
Linear classifier on sensor data with plot patterns and filters