mne.viz.plot_topomap#
- mne.viz.plot_topomap(data, pos, *, ch_type='eeg', sensors=True, names=None, mask=None, mask_params=None, contours=6, outlines='head', sphere=None, image_interp='cubic', extrapolate='auto', border='mean', res=64, size=1, cmap=None, vlim=(None, None), cnorm=None, axes=None, show=True, onselect=None)[source]#
Plot a topographic map as image.
- Parameters:
- data
array
, shape (n_chan,) The data values to plot.
- pos
array
, shape (n_channels, 2) | instance ofInfo
Location information for the channels. If an array, should provide the x and y coordinates for plotting the channels in 2D. If an
Info
object it must contain only one channel type and exactlylen(data)
channels; the x/y coordinates will be inferred from the montage in theInfo
object.- ch_type‘mag’ | ‘grad’ | ‘planar1’ | ‘planar2’ | ‘eeg’ |
None
The channel type to plot. For
'grad'
, the gradiometers are collected in pairs and the RMS for each pair is plotted. IfNone
the first available channel type from order shown above is used. Defaults toNone
.New in v0.21.
- sensorsbool |
str
Whether to add markers for sensor locations. If
str
, should be a valid matplotlib format string (e.g.,'r+'
for red plusses, see the Notes section ofplot()
). IfTrue
(the default), black circles will be used.- names
None
|list
Labels for the sensors. If a
list
, labels should correspond to the order of channels indata
. IfNone
(default), no channel names are plotted.- mask
ndarray
of bool, shape (n_channels,) |None
Array indicating channel(s) to highlight with a distinct plotting style. Array elements set to
True
will be plotted with the parameters given inmask_params
. Defaults toNone
, equivalent to an array of allFalse
elements.- mask_params
dict
|None
Additional plotting parameters for plotting significant sensors. Default (None) equals:
dict(marker='o', markerfacecolor='w', markeredgecolor='k', linewidth=0, markersize=4)
- contours
int
| array_like The number of contour lines to draw. If
0
, no contours will be drawn. If a positive integer, that number of contour levels are chosen using the matplotlib tick locator (may sometimes be inaccurate, use array for accuracy). If array-like, the array values are used as the contour levels. The values should be in µV for EEG, fT for magnetometers and fT/m for gradiometers. Ifcolorbar=True
, the colorbar will have ticks corresponding to the contour levels. Default is6
.- outlines‘head’ |
dict
|None
The outlines to be drawn. If ‘head’, the default head scheme will be drawn. If dict, each key refers to a tuple of x and y positions, the values in ‘mask_pos’ will serve as image mask. Alternatively, a matplotlib patch object can be passed for advanced masking options, either directly or as a function that returns patches (required for multi-axis plots). If None, nothing will be drawn. Defaults to ‘head’.
- sphere
float
| array_like | instance ofConductorModel
|None
| ‘auto’ | ‘eeglab’ The sphere parameters to use for the head outline. Can be array-like of shape (4,) to give the X/Y/Z origin and radius in meters, or a single float to give just the radius (origin assumed 0, 0, 0). Can also be an instance of a spherical
ConductorModel
to use the origin and radius from that object. If'auto'
the sphere is fit to digitization points. If'eeglab'
the head circle is defined by EEG electrodes'Fpz'
,'Oz'
,'T7'
, and'T8'
(if'Fpz'
is not present, it will be approximated from the coordinates of'Oz'
).None
(the default) is equivalent to'auto'
when enough extra digitization points are available, and (0, 0, 0, 0.095) otherwise.New in v0.20.
Changed in version 1.1: Added
'eeglab'
option.- image_interp
str
The image interpolation to be used. Options are
'cubic'
(default) to usescipy.interpolate.CloughTocher2DInterpolator
,'nearest'
to usescipy.spatial.Voronoi
or'linear'
to usescipy.interpolate.LinearNDInterpolator
.- extrapolate
str
Options:
'box'
Extrapolate to four points placed to form a square encompassing all data points, where each side of the square is three times the range of the data in the respective dimension.
'local'
(default for MEG sensors)Extrapolate only to nearby points (approximately to points closer than median inter-electrode distance). This will also set the mask to be polygonal based on the convex hull of the sensors.
'head'
(default for non-MEG sensors)Extrapolate out to the edges of the clipping circle. This will be on the head circle when the sensors are contained within the head circle, but it can extend beyond the head when sensors are plotted outside the head circle.
New in v0.18.
Changed in version 0.21:
The default was changed to
'local'
for MEG sensors.'local'
was changed to use a convex hull mask'head'
was changed to extrapolate out to the clipping circle.
- border
float
| ‘mean’ Value to extrapolate to on the topomap borders. If
'mean'
(default), then each extrapolated point has the average value of its neighbours.New in v0.20.
- res
int
The resolution of the topomap image (number of pixels along each side).
- size
float
Side length of each subplot in inches.
- cmapmatplotlib colormap | (colormap, bool) | ‘interactive’ |
None
Colormap to use. If
tuple
, the first value indicates the colormap to use and the second value is a boolean defining interactivity. In interactive mode the colors are adjustable by clicking and dragging the colorbar with left and right mouse button. Left mouse button moves the scale up and down and right mouse button adjusts the range. Hitting space bar resets the range. Up and down arrows can be used to change the colormap. IfNone
,'Reds'
is used for data that is either all-positive or all-negative, and'RdBu_r'
is used otherwise.'interactive'
is equivalent to(None, True)
. Defaults toNone
.Warning
Interactive mode works smoothly only for a small amount of topomaps. Interactive mode is disabled by default for more than 2 topomaps.
- vlim
tuple
of length 2 Lower and upper bounds of the colormap, typically a numeric value in the same units as the data. If both entries are
None
, the bounds are set at(min(data), max(data))
. ProvidingNone
for just one entry will set the corresponding boundary at the min/max of the data. Defaults to(None, None)
.New in v1.2.
- cnorm
matplotlib.colors.Normalize
|None
How to normalize the colormap. If
None
, standard linear normalization is performed. If notNone
,vmin
andvmax
will be ignored. See Matplotlib docs for more details on colormap normalization, and the ERDs example for an example of its use.New in v0.24.
- axesinstance of
Axes
|None
The axes to plot into. If
None
, a newFigure
will be created. Default isNone
.Changed in version 1.2: If
axes=None
, a newFigure
is created instead of plotting into the current axes.- showbool
Show the figure if
True
.- onselect
callable()
|None
A function to be called when the user selects a set of channels by click-dragging (uses a matplotlib
RectangleSelector
). IfNone
interactive channel selection is disabled. Defaults toNone
.
- data
- Returns:
- im
matplotlib.image.AxesImage
The interpolated data.
- cn
matplotlib.contour.ContourSet
The fieldlines.
- im
Examples using mne.viz.plot_topomap
#
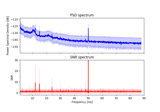
Frequency-tagging: Basic analysis of an SSVEP/vSSR dataset